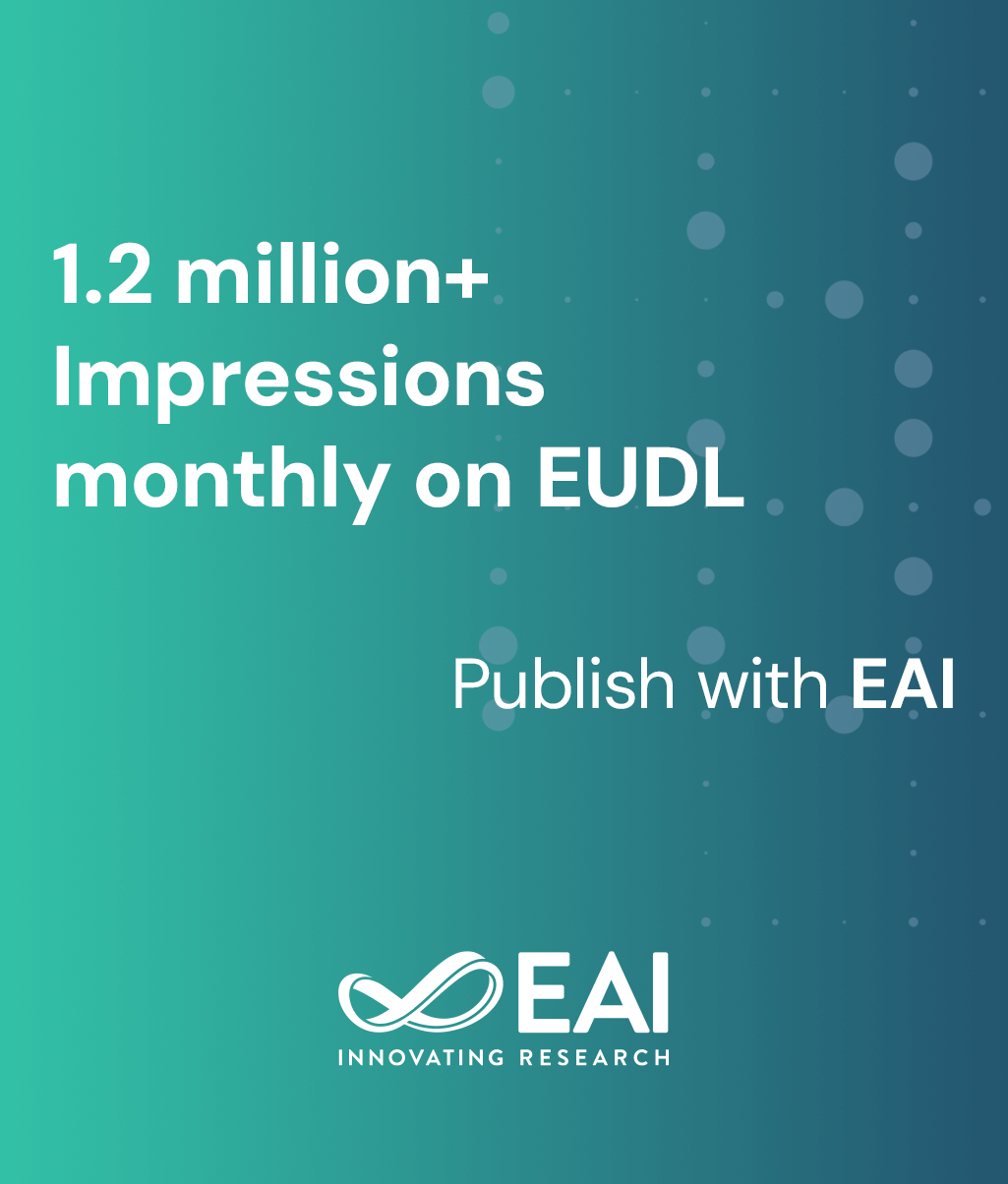
Research Article
Comparative analysis of performance of AutoML algorithms: Classification model of payment arrears in students of a private university
@ARTICLE{10.4108/eetsis.4550, author={Henry Villarreal-Torres and Julio \^{A}ngeles-Morales and Jenny Cano-Mej\^{\i}a and Carmen Mej\^{\i}a-Murillo and Gumercindo Flores-Reyes and Oscar Cruz-Cruz and Manuel Urcia-Quispe and Manuel Palomino-M\^{a}rquez and Miguel \^{A}ngel Solar-Jara and Reyna Escobedo-Zarzosa}, title={Comparative analysis of performance of AutoML algorithms: Classification model of payment arrears in students of a private university}, journal={EAI Endorsed Transactions on Scalable Information Systems}, volume={11}, number={4}, publisher={EAI}, journal_a={SIS}, year={2024}, month={11}, keywords={payment arrears, AutoML, AutoKeras, AutoGluon, HyperOPT, MLJar and H2O}, doi={10.4108/eetsis.4550} }
- Henry Villarreal-Torres
Julio Ángeles-Morales
Jenny Cano-Mejía
Carmen Mejía-Murillo
Gumercindo Flores-Reyes
Oscar Cruz-Cruz
Manuel Urcia-Quispe
Manuel Palomino-Márquez
Miguel Ángel Solar-Jara
Reyna Escobedo-Zarzosa
Year: 2024
Comparative analysis of performance of AutoML algorithms: Classification model of payment arrears in students of a private university
SIS
EAI
DOI: 10.4108/eetsis.4550
Abstract
The impact of artificial intelligence in our society is important due to the innovation of processes through data science to know the academic and sociodemographic factors that contribute to late payments in university students, to identify them and make timely decisions for implementing prevention and correction programs, avoiding student dropout due to this economic problem, and ensuring success in their education in a meaningful and focused way. In this sense, the research aims to compare the performance metrics of classification models for late payments in students of a private university by using AutoML algorithms from various existing platforms and solutions such as AutoKeras, AutoGluon, HyperOPT, MLJar, and H2O in a data set consisting of 8,495 records and the application of data balancing techniques. From the implementation and execution of various algorithms, similar metrics have been obtained based on the parameters and optimization functions used automatically by each tool, providing better performance to the H2O platform through the Stacked Ensemble algorithm with metrics accuracy = 0.778. F1 = 0.870, recall = 0.904 and precision = 0.839. The research can be extended to other contexts or areas of knowledge due to the growing interest in automated machine learning, providing researchers with a valuable tool in data science without the need for deep knowledge.
Copyright © 2023 Villareal-Torres et al., licensed to EAI. This is an open access article distributed under the terms of the CC BY-NCSA 4.0, which permits copying, redistributing, remixing, transformation, and building upon the material in any medium so long as the original work is properly cited.