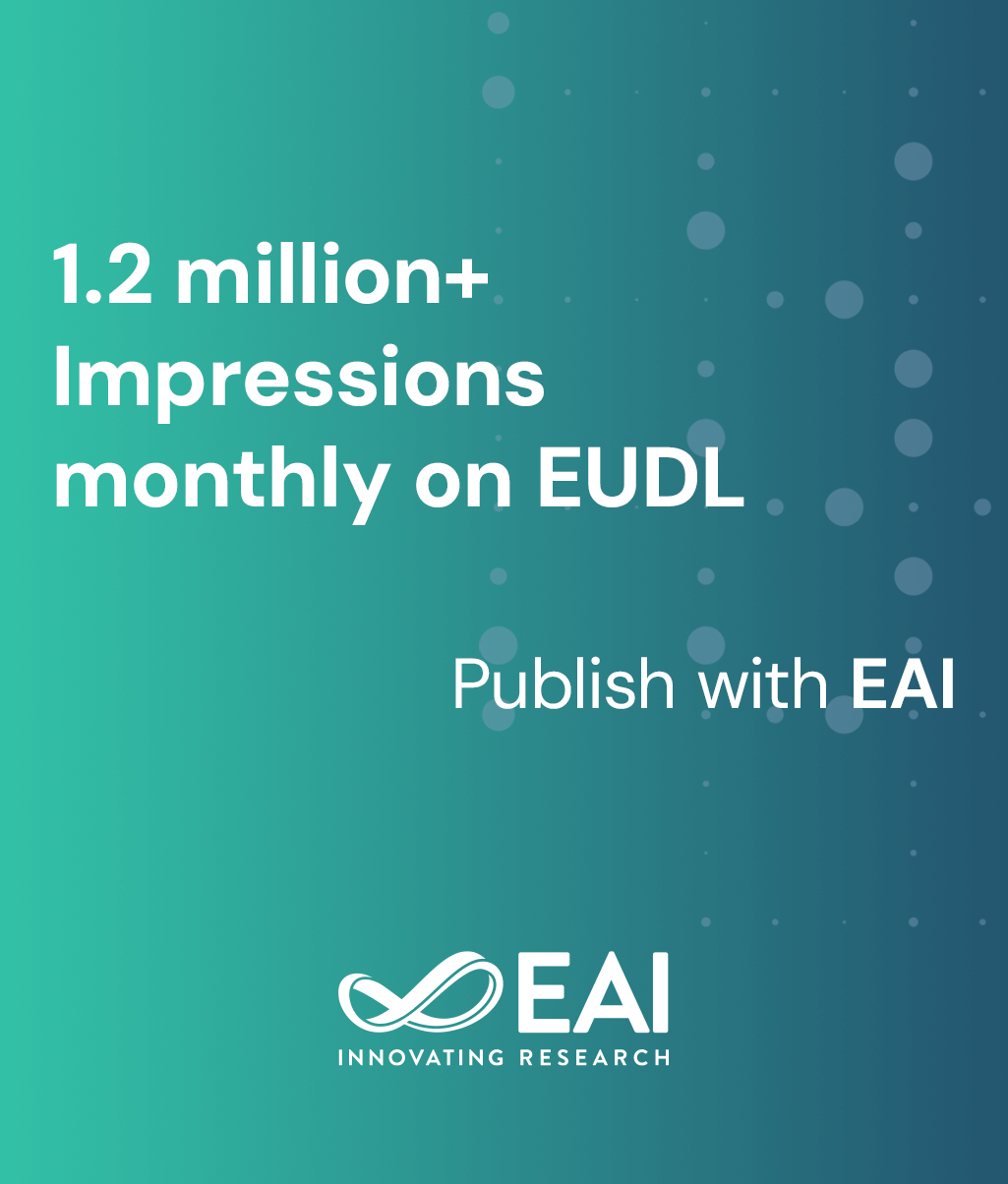
Research Article
Event Extraction with Spectrum Estimation Using Neural Networks Linear Methods
@ARTICLE{10.4108/eetsis.4355, author={Vuyyuru Madhavi}, title={Event Extraction with Spectrum Estimation Using Neural Networks Linear Methods}, journal={EAI Endorsed Transactions on Scalable Information Systems}, volume={11}, number={4}, publisher={EAI}, journal_a={SIS}, year={2023}, month={11}, keywords={Spectrum estimation, Event extraction, Movie scene arguments extraction, Scene trigger extraction, Graph attention system}, doi={10.4108/eetsis.4355} }
- Vuyyuru Madhavi
Year: 2023
Event Extraction with Spectrum Estimation Using Neural Networks Linear Methods
SIS
EAI
DOI: 10.4108/eetsis.4355
Abstract
The timely extraction of event information from enormous amounts of textual data is a critical research problem. Event extraction using deep learning technology has gained academic attention as a result of the fast growth of deep learning. Event extraction requires costly, expert-level, high-quality human annotations. As a result, developing a data-efficient event extraction model that can be trained using only a few labelled samples has emerged as a key difficulty. Existing research work focuses mainly on the structured data with supervised models. The proposed work focuses on Movie Scene Event Extraction, a practical media analysis problem that seeks to extract structured events from unstructured movie screenplays. We suggest using the correlation between various argument roles in situations where different argument roles in a movie scene share similar qualities. This can be beneficial to the Movie Scene Argument Extraction (argument classification and identification) and film scene trigger extraction (Trigger recognition and classifying). In order to represent the relation between different roles in argument and their respective roles, we propose a Superior Concept of Role (SRC) as a top-level idea of a role that is based on the classic argument role, as well as an SRC-based Graph Attention System (GAT). To assess the efficacy of the model we designed, we constructed the dataset MovieSceneEvent to extract movies’ scene-related events. Additionally, we conducted tests on an existing dataset in order to compare results with different models. Results from the experiments like extraction of words, aspect keywords from the documents indicate that our model does better than other models. Furthermore, the information on the relationship between the argument roles helps improve the effectiveness of film scene extraction of events.
Copyright © 2023 V. Madhavi et al., licensed to EAI. This is an open access article distributed under the terms of the CC BY-NC-SA 4.0, which permits copying, redistributing, remixing, transformation, and building upon the material in any medium so long as the original work is properly cited.