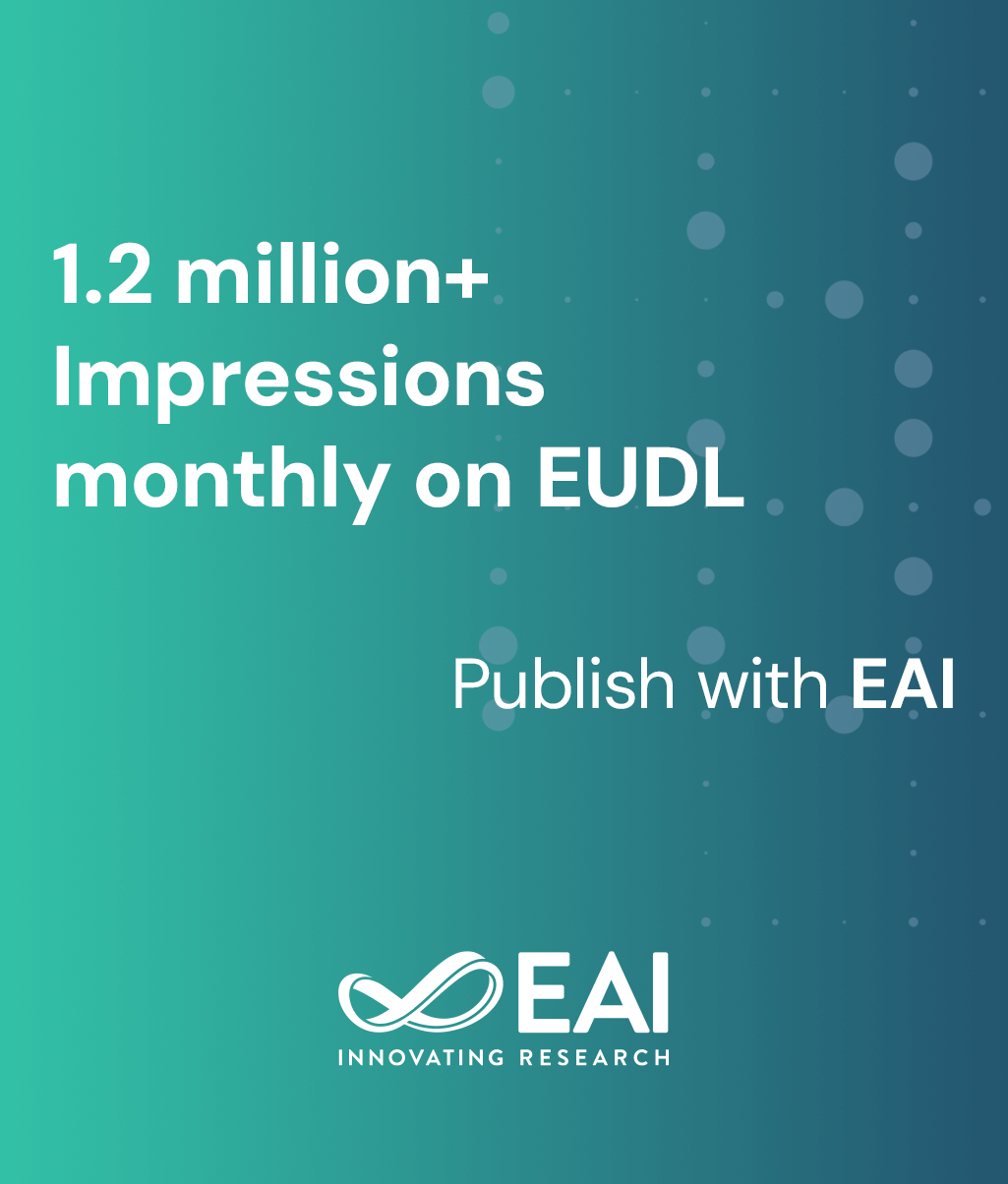
Research Article
Digital Investigation of Network Traffic Using Machine Learning
@ARTICLE{10.4108/eetsis.4055, author={Saswati Chatterjee and Suneeta Satpathy and Arpita Nibedita}, title={Digital Investigation of Network Traffic Using Machine Learning}, journal={EAI Endorsed Transactions on Scalable Information Systems}, volume={11}, number={1}, publisher={EAI}, journal_a={SIS}, year={2023}, month={10}, keywords={KDD, Hybrid Machine Learning, Network forensics, DDoS}, doi={10.4108/eetsis.4055} }
- Saswati Chatterjee
Suneeta Satpathy
Arpita Nibedita
Year: 2023
Digital Investigation of Network Traffic Using Machine Learning
SIS
EAI
DOI: 10.4108/eetsis.4055
Abstract
In this study, an intelligent system that can gather and process network packets is built. Machine learning techniques are used to create a traffic classifier that divides packets into hazardous and non-malicious categories. The system utilizing resources was previously classified using a number of conventional techniques; however, this strategy adds machine learning., a study area that is currently active and has so far yielded promising results. The major aims of this paper are to monitor traffic, analyze incursions, and control them. The flow of data collection is used to develop a traffic classification system based on features of observed internet packets. This classification will aid IT managers in recognizing the vague assault that is becoming more common in the IT industry The suggested methods described in this research help gather network data and detect which threat was launched in a specific network to distinguish between malicious and benign packets. This paper’s major goal is to create a proactive system for detecting network attacks using classifiers based on machine learning that can recognize new packets and distinguish between hostile and benign network packets using rules from the KDD dataset. The algorithm is trained to employ the characteristics of the NSL-KDD dataset.
Copyright © 2023 S. Chatterjee et al., licensed to EAI. This is an open access article distributed under the terms of the CC BY-NC-SA 4.0, which permits copying, redistributing, remixing, transformation, and building upon the material in any medium so long as the original work is properly cited.