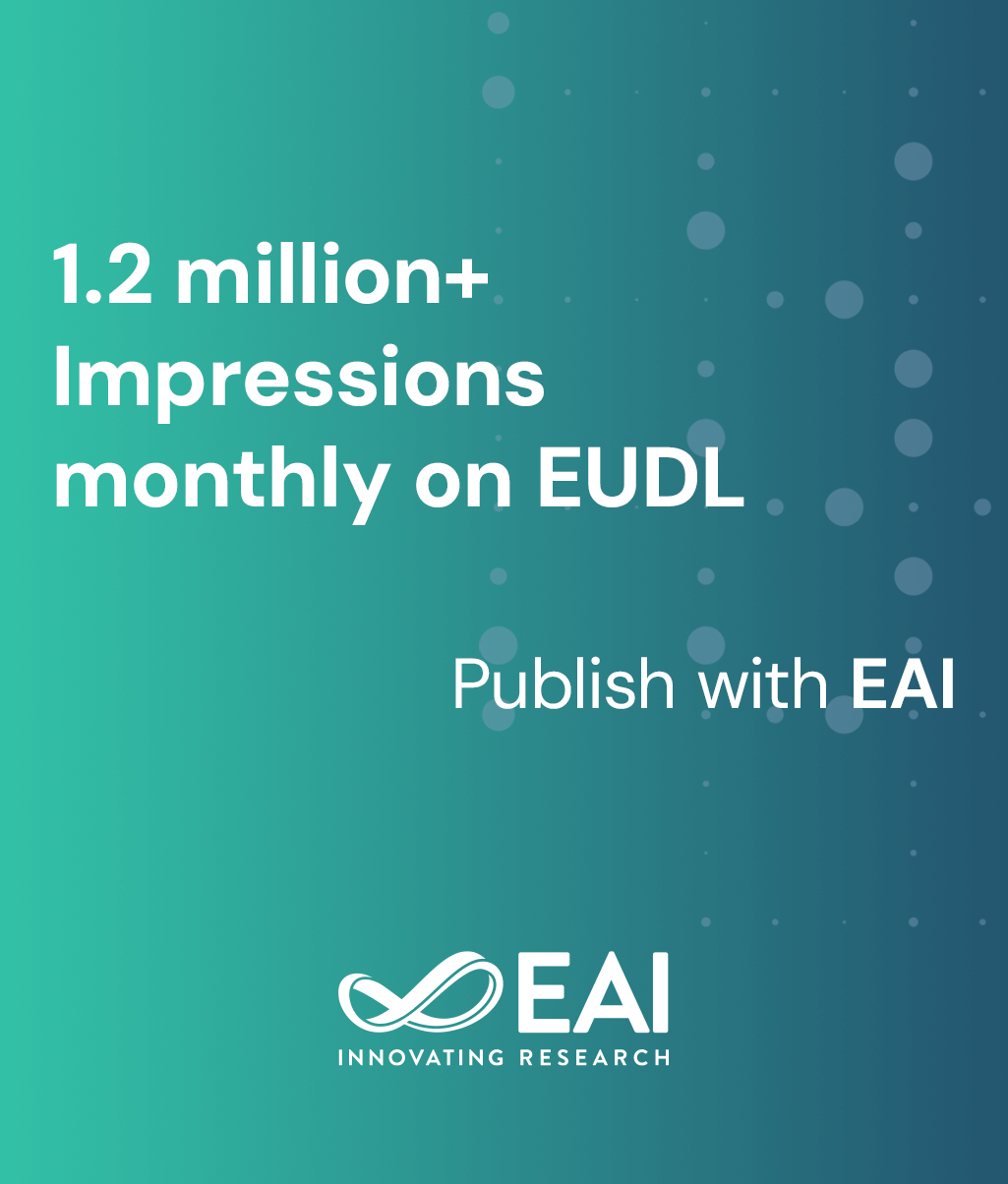
Research Article
Enhanced Task Scheduling Using Optimized Particle Swarm Optimization Algorithm in Cloud Computing Environment
@ARTICLE{10.4108/eetsis.4042, author={Sirisha Potluri and Abdulsattar Abdullah Hamad and Deepthi Godavarthi and Santi Swarup Basa}, title={Enhanced Task Scheduling Using Optimized Particle Swarm Optimization Algorithm in Cloud Computing Environment}, journal={EAI Endorsed Transactions on Scalable Information Systems}, volume={11}, number={3}, publisher={EAI}, journal_a={SIS}, year={2023}, month={8}, keywords={Cloud Computing, Load Balancing, High-Performance Computing, Task Scheduling, Job Scheduling, Particle Swarm Optimization}, doi={10.4108/eetsis.4042} }
- Sirisha Potluri
Abdulsattar Abdullah Hamad
Deepthi Godavarthi
Santi Swarup Basa
Year: 2023
Enhanced Task Scheduling Using Optimized Particle Swarm Optimization Algorithm in Cloud Computing Environment
SIS
EAI
DOI: 10.4108/eetsis.4042
Abstract
The most significant constraint in cloud computing infrastructure is the job/task scheduling which affords the vital role of efficiency of the entire cloud computing services and offerings. Job/ task scheduling in cloud infrastructure means that to assign best appropriate cloud resources for the given job/task by considering of different factors: execution time and cost, infrastructure scalability and reliability, platform availability and throughput, resource utilization and makespan. The proposed enhanced task scheduling algorithm using particle swarm optimization considers optimization of makespan and scheduling time. We propose the proposed model by using dynamic adjustment of parameters with discrete positioning (DAPDP) based algorithm to schedule and allocate cloud jobs/tasks that ensues optimized makespan and scheduling time. DAPDP can witness a substantial role in attaining reliability in by seeing the available, scheduled and allocated cloud resources. Our approach DAPDP compared with other existing particle swarm and optimization job/task scheduling algorithms to prove that DAPDP can save in makespan, scheduling and execution time.
Copyright © 2023 S. Potluri et al., licensed to EAI. This is an open access article distributed under the terms of the CC BY-NC-SA 4.0, which permits copying, redistributing, remixing, transformation, and building upon the material in any medium so long as the original work is properly cited.