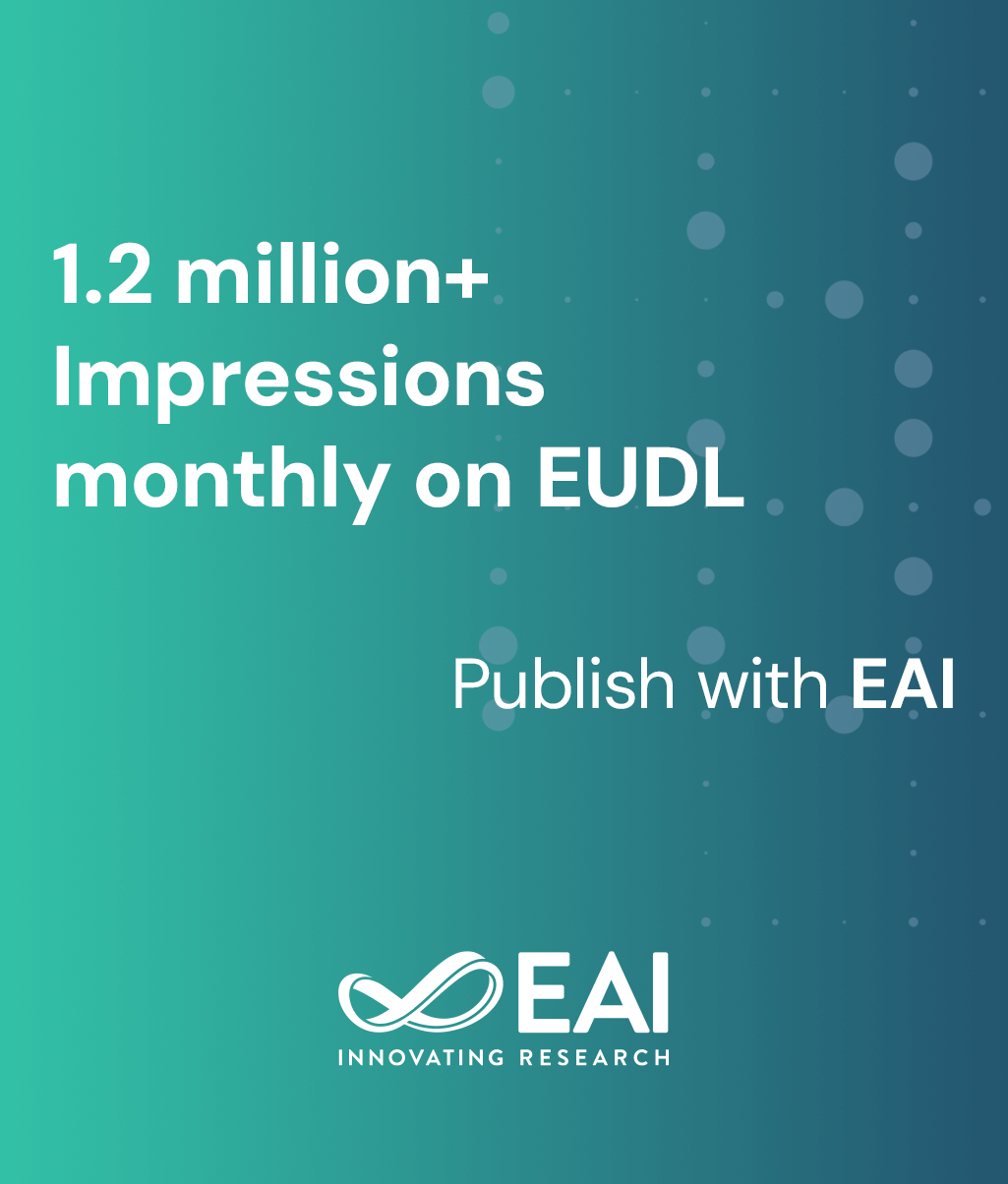
Research Article
Enhancing IoT Botnet Detection through Machine Learning-based Feature Selection and Ensemble Models
@ARTICLE{10.4108/eetsis.3971, author={Ravi Sharma and Saika Mohi ud din and Nonita Sharma and Arun Kumar}, title={Enhancing IoT Botnet Detection through Machine Learning-based Feature Selection and Ensemble Models}, journal={EAI Endorsed Transactions on Scalable Information Systems}, volume={11}, number={2}, publisher={EAI}, journal_a={SIS}, year={2023}, month={9}, keywords={IoT, Botnet, Botnet Detection, Ensemble Model, Voting Ensemble, Ada Boost, KNN, Bootstrap Aggregation}, doi={10.4108/eetsis.3971} }
- Ravi Sharma
Saika Mohi ud din
Nonita Sharma
Arun Kumar
Year: 2023
Enhancing IoT Botnet Detection through Machine Learning-based Feature Selection and Ensemble Models
SIS
EAI
DOI: 10.4108/eetsis.3971
Abstract
An increase in cyberattacks has coincided with the Internet of Things (IoT) expansion. When numerous systems are connected, more botnet attacks are possible. Because botnet attacks are constantly evolving to take advantage of security holes and weaknesses in internet traffic and IoT devices, they must be recognized. Voting ensemble (VE), Ada boost, K-Nearest Neighbour (KNN), and bootstrap aggregation are some methods used in this work for botnet detection. This study aims to first incorporate feature significance for enhanced efficacy, then estimate effectiveness in IoT botnet detection using traditional model-based machine learning, and finally evaluate the outcomes using ensemble models. It has been demonstrated that applying feature importance increases the effectiveness of ensemble models. VE algorithm provides the best botnet traffic detection compared to all currently used approaches.
Copyright © 2023 R Sharma et al., licensed to EAI. This is an open access article distributed under the terms of the CC BY-NC-SA 4.0, which permits copying, redistributing, remixing, transformation, and building upon the material in any medium so long as the original work is properly cited.