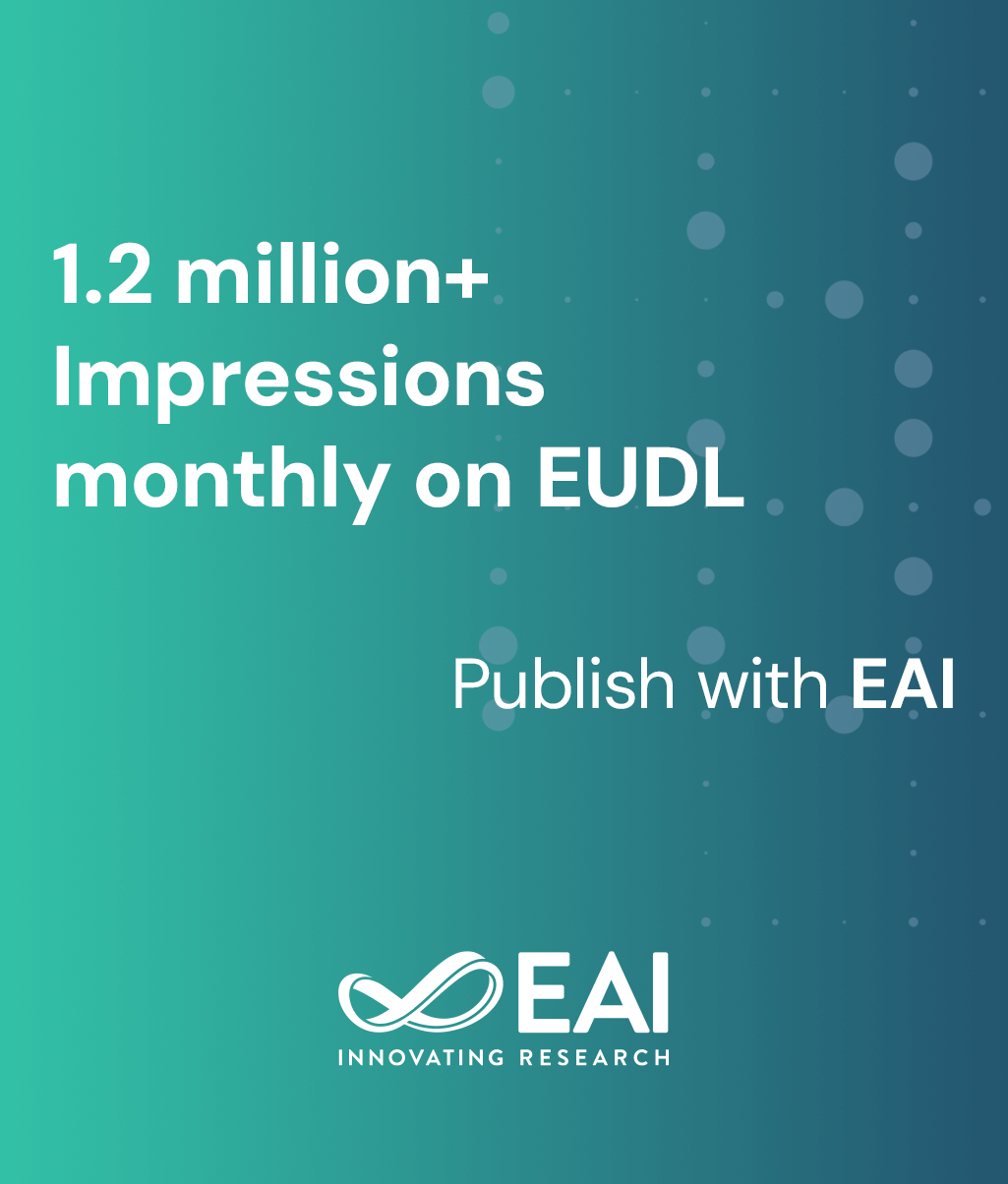
Research Article
Cloud DDoS Attack Detection Model with Data Fusion & Machine Learning Classifiers
@ARTICLE{10.4108/eetsis.3936, author={Lal Mohan Pattnaik and Pratik Kumar Swain and Suneeta Satpathy and Aditya N. Panda}, title={Cloud DDoS Attack Detection Model with Data Fusion \& Machine Learning Classifiers}, journal={EAI Endorsed Transactions on Scalable Information Systems}, volume={10}, number={6}, publisher={EAI}, journal_a={SIS}, year={2023}, month={9}, keywords={Cloud Security, DDoS, Machine Learning, Data Fusion}, doi={10.4108/eetsis.3936} }
- Lal Mohan Pattnaik
Pratik Kumar Swain
Suneeta Satpathy
Aditya N. Panda
Year: 2023
Cloud DDoS Attack Detection Model with Data Fusion & Machine Learning Classifiers
SIS
EAI
DOI: 10.4108/eetsis.3936
Abstract
In the current situation, digital technology is a necessary component of daily life for people. During the Covid-19 pandemic, every profit and non-profit making businesses organizations moved online, which caused an exponential rise in incursions and attacks on the digital platform. The Distributed Denial of Service (DDoS) attack, which may quickly paralyse Internet-based services and applications, is one of the deadly threats to emerge. The attackers regularly update their skill tactics, which allows them to get around the current detection and protection systems. The standard detection systems are ineffective for identifying novel DDoS attacks since the volume of data generated and stored has multiplied. So, the main goal of this work is to employ data fusion applications for secure cloud services and demonstrate the detection of DDoS attacks with the applications of machine learning classifiers that can further be helpful for cloud forensic investigation process. A variety of machine learning models, including decision trees, Navies Bayes, SVM, and KNN are used to detect and classify cloud DDoS attacks. The outcomes of the experiments demonstrated that decision tree is the most feasible and better performer method to classify cloud DDoS attacks.
Copyright © 2023 L. M. Pattnaik et al., licensed to EAI. This is an open access article distributed under the terms of the CC BY-NCSA 4.0, which permits copying, redistributing, remixing, transformation, and building upon the material in any medium so long as the original work is properly cited.