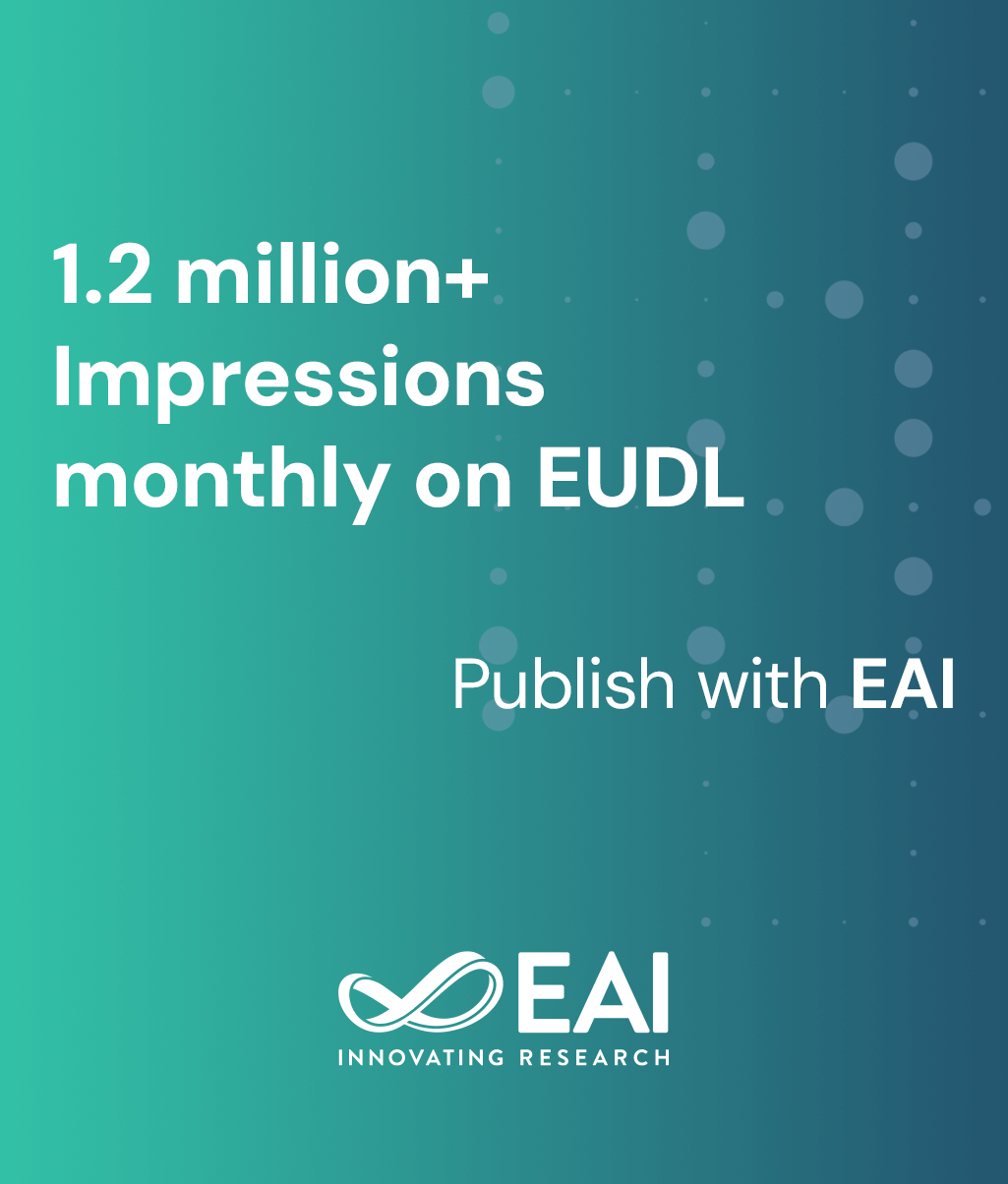
Research Article
Deep spectral network for time series clustering
@ARTICLE{10.4108/eetsis.3907, author={Duc-Trung Hoang and Mahdi Achache and Vinay Kumar Jain}, title={Deep spectral network for time series clustering}, journal={EAI Endorsed Transactions on Scalable Information Systems}, volume={11}, number={3}, publisher={EAI}, journal_a={SIS}, year={2023}, month={9}, keywords={Deep clustering, neural network, time series, spectral clustering, mutual information, multi label classification}, doi={10.4108/eetsis.3907} }
- Duc-Trung Hoang
Mahdi Achache
Vinay Kumar Jain
Year: 2023
Deep spectral network for time series clustering
SIS
EAI
DOI: 10.4108/eetsis.3907
Abstract
Deep clustering is an approach that uses deep learning to cluster data, since it involves training a neural network model to become familiar with a data representation that is suitable for clustering. Deep clustering has been applied to a wide range of data types, including images, texts, time series and has the advantage of being able to automatically learn features from the data, which can be more effective than using hand-crafted features. It is also able to handle high-dimensional data, such as time series with many variables, which can be challenging for traditional clustering techniques. In this paper, we introduce a novel deep neural network type to improve the performance of the auto-encoder part by ignoring the unnecessary extra-noises and labelling the input data. Our approach is helpful when just a limited amount of labelled data is available, but labelling a big amount of data would be costly or time-consuming. It also applies for the data in high-dimensional and difficult to define a good set of features for clustering.
Copyright © 2023 D. T. Hoang et al., licensed to EAI. This is an open access article distributed under the terms of the Creative Commons Attribution license (http://creativecommons.org/licenses/by/4.0/), which permits unlimited use, distribution and reproduction in any medium so long as the original work is properly cited.