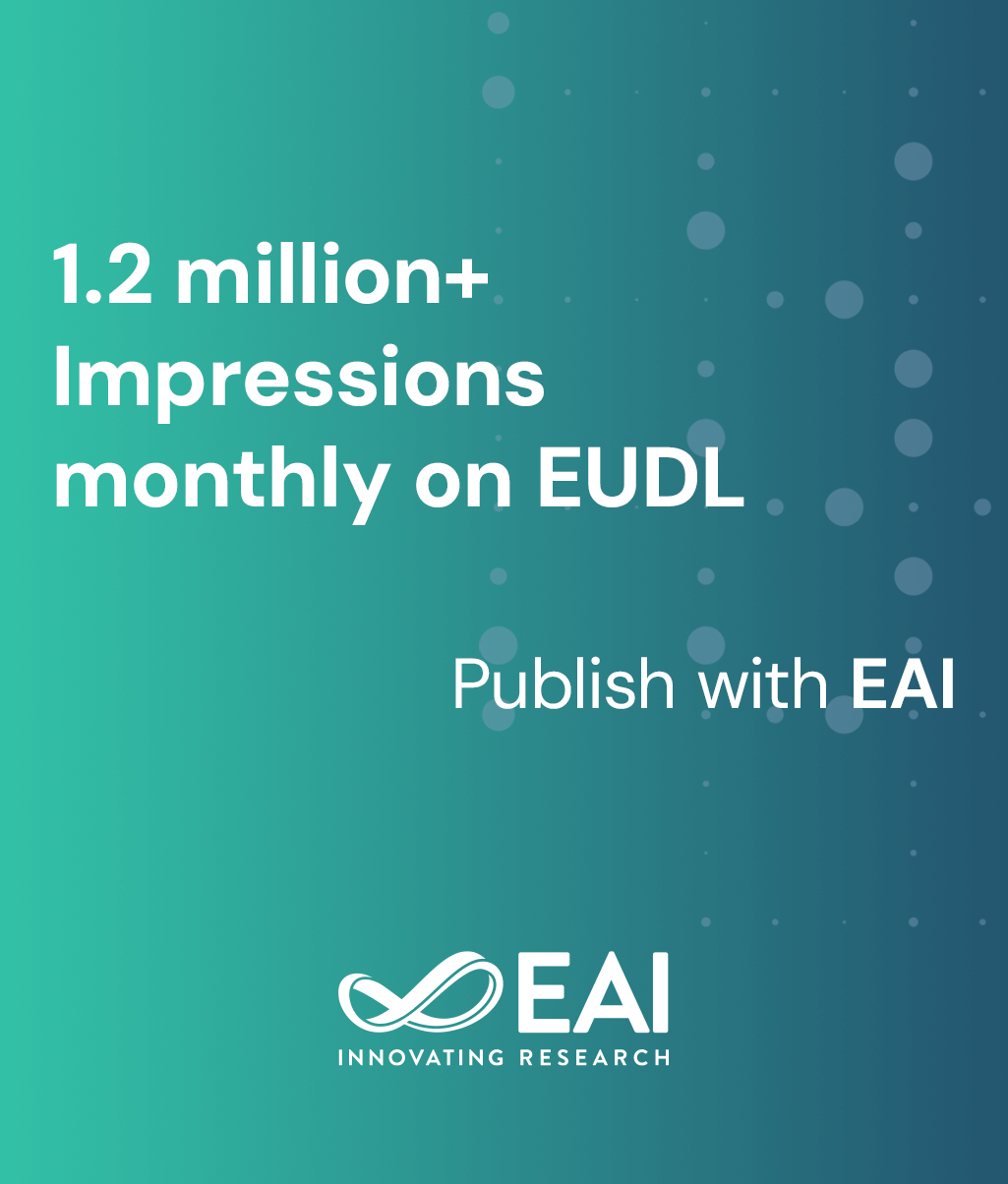
Research Article
Fortified MapReduce Layer: Elevating Security and Privacy in Big Data
@ARTICLE{10.4108/eetsis.3859, author={Manish Gupta and Rajendra Kumar Dwivedi}, title={Fortified MapReduce Layer: Elevating Security and Privacy in Big Data}, journal={EAI Endorsed Transactions on Scalable Information Systems}, volume={10}, number={6}, publisher={EAI}, journal_a={SIS}, year={2023}, month={10}, keywords={Big Data, Privacy, Security, HDFS, Map Reduce}, doi={10.4108/eetsis.3859} }
- Manish Gupta
Rajendra Kumar Dwivedi
Year: 2023
Fortified MapReduce Layer: Elevating Security and Privacy in Big Data
SIS
EAI
DOI: 10.4108/eetsis.3859
Abstract
In today's digital landscape, the widespread sharing and utilization of raw data are integral in social, medical, agricultural, and academic domains. The surge of open platforms has led to exponential growth in data, transforming it into what we now call Big Data (BD). However, the traditional BD model lacks a specific mechanism for capturing the sensitivity of data, leaving it vulnerable to potential breaches. To address this, a privacy and security layer is crucial. This paper propose a novel solution called the Fortified Secured Map Reduce (FSMR) Layer, which serves as an intermediary between the HDFS (Hadoop Distributed File System) and MR (Map Reduce) Layer. The FSMR model is designed to foster data sharing for knowledge mining while ensuring robust privacy and security guarantees. It effectively resolves scalability issues concerning privacy and strikes a balance between privacy and utility for data miners. By implementing the FSMR model, we achieve remarkable improvements in running time and information loss compared to existing approaches. Furthermore, storage and CPU utilization are minimized, enhancing the overall efficiency and effectiveness of the data processing pipeline. The outcome of our work lies in promoting data sharing while safeguarding sensitive information, making it a significant step towards secure and privacy-conscious BD processing.
Copyright © 2023 M. K. Gupta et al., licensed to EAI. This is an open access article distributed under the terms of theCC BY-NC-SA 4.0, which permits copying, redistributing, remixing, transformation, and building upon the material in any medium so long as the original work is properly cited.