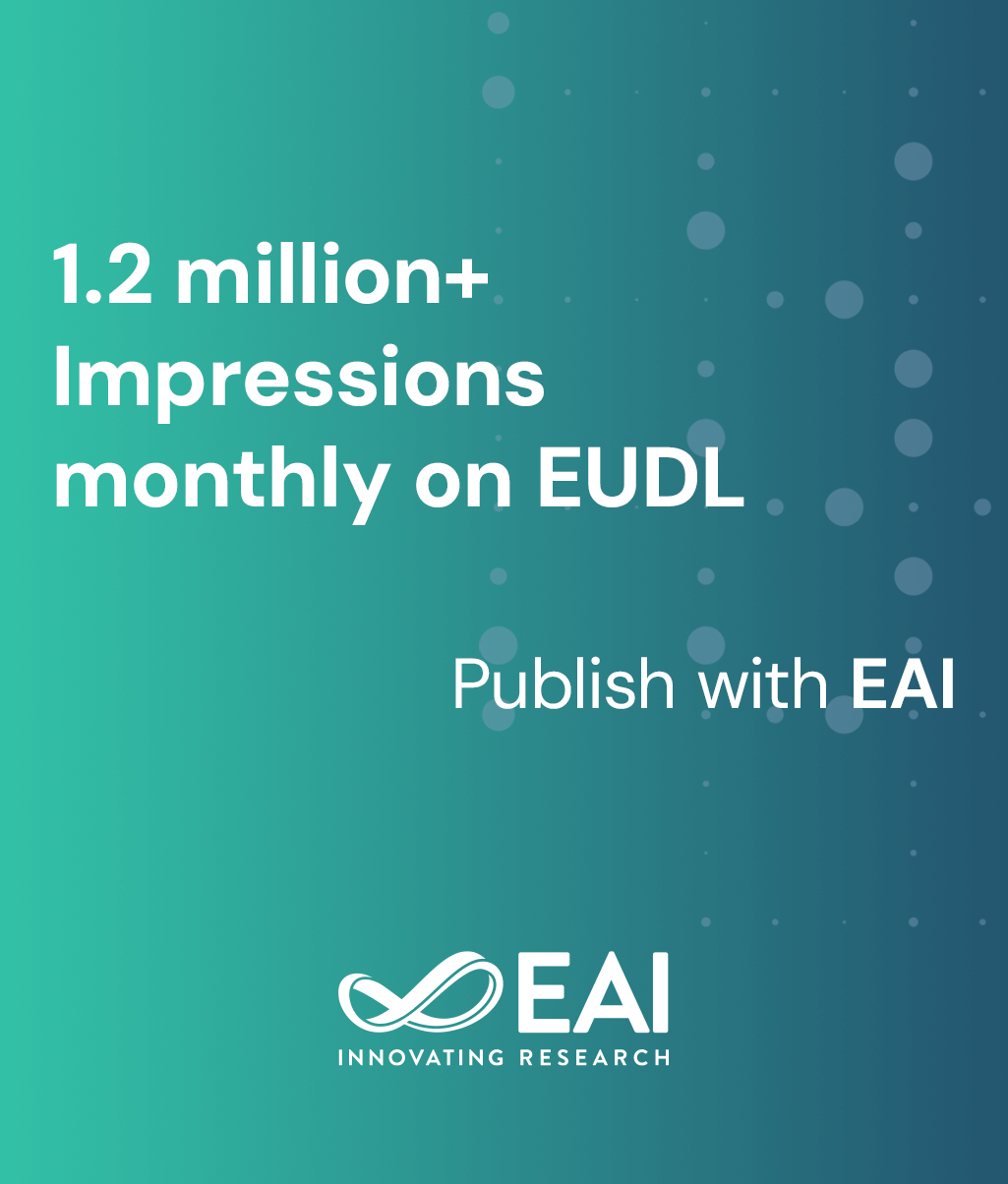
Research Article
Textual Information Processing Based on Multi-Dimensional Indicator Weights
@ARTICLE{10.4108/eetsis.3805, author={Yuliang Yang and Zhengping Lin and Yuzhong Zhou and Jiahao Shi and Jie Lin}, title={Textual Information Processing Based on Multi-Dimensional Indicator Weights}, journal={EAI Endorsed Transactions on Scalable Information Systems}, volume={11}, number={2}, publisher={EAI}, journal_a={SIS}, year={2023}, month={12}, keywords={Textual information processing, semantic information, multi-dimensional indicator weights, artificial intelligence}, doi={10.4108/eetsis.3805} }
- Yuliang Yang
Zhengping Lin
Yuzhong Zhou
Jiahao Shi
Jie Lin
Year: 2023
Textual Information Processing Based on Multi-Dimensional Indicator Weights
SIS
EAI
DOI: 10.4108/eetsis.3805
Abstract
With the rapid advancement of artificial intelligence and wireless communication technologies, the abundance of textual information has grown significantly, accompanied by a plethora of multidimensional metrics such as innovation, application prospects, key technologies, and expected outcomes. Extracting valuable insights from these multifaceted indicators and establishing an effective composite evaluation weighting framework poses a pivotal challenge in text information processing. In response, we propose a novel approach in this paper to textual information processing, leveraging multi-dimensional indicator weights (MDIWs). Our method involves extracting semantic information from text and inputting it into an LSTM-based textual information processor (TIP) to generate MDIWs. These MDIWs are then processed to create a judgment matrix following by eigenvalue decomposition and normalization, capturing intricate semantic relationships. Our framework enhances the comprehension of multi-dimensional aspects within textual data, offering potential benefits in various applications such as sentiment analysis, information retrieval, and content summarization. Experimental results underscore the effectiveness of our approach in refining and utilizing MDIWs for improved understanding and decision-making. This work contributes to the enhancement of text information processing by offering a structured approach to address the complexity of multidimensional metric evaluation, thus enabling more accurate and informed decision-making in various domains.
Copyright © 2023 Y. Yang et al., licensed to EAI. This is an open access article distributed under the terms of the Creative Commons Attribution license, which permits unlimited use, distribution and reproduction in any medium so long as the original work is properly cited.