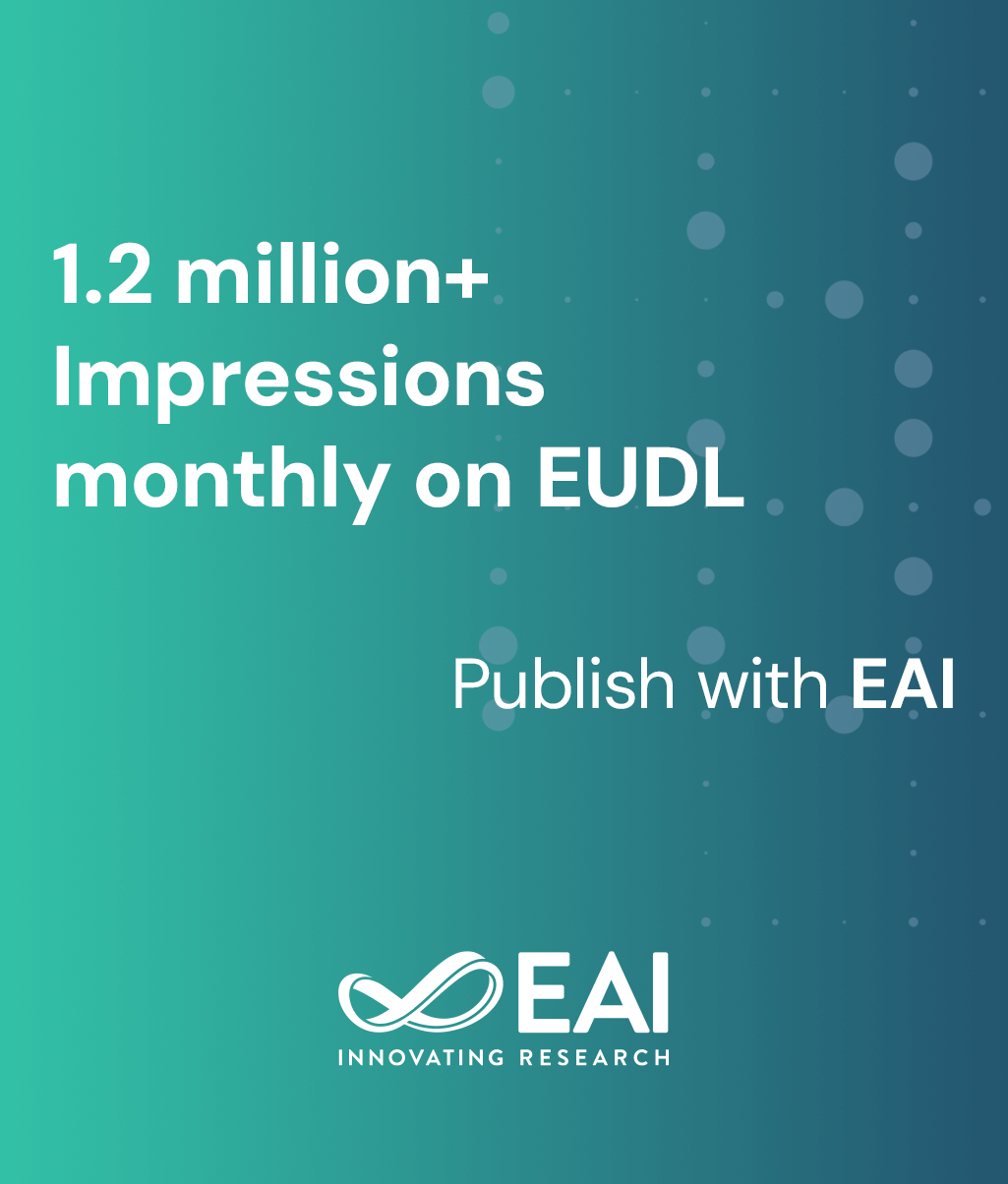
Research Article
Compression and Transmission of Big AI Model Based on Deep Learning
@ARTICLE{10.4108/eetsis.3803, author={Zhengping Lin and Yuzhong Zhou and Yuliang Yang and Jiahao Shi and Jie Lin}, title={Compression and Transmission of Big AI Model Based on Deep Learning}, journal={EAI Endorsed Transactions on Scalable Information Systems}, volume={11}, number={2}, publisher={EAI}, journal_a={SIS}, year={2023}, month={12}, keywords={Big AI model, compression and transmission, deep learning, convolutional networks}, doi={10.4108/eetsis.3803} }
- Zhengping Lin
Yuzhong Zhou
Yuliang Yang
Jiahao Shi
Jie Lin
Year: 2023
Compression and Transmission of Big AI Model Based on Deep Learning
SIS
EAI
DOI: 10.4108/eetsis.3803
Abstract
In recent years, big AI models have demonstrated remarkable performance in various artificial intelligence (AI) tasks. However, their widespread use has introduced significant challenges in terms of model transmission and training. This paper addresses these challenges by proposing a solution that involves the compression and transmission of large models using deep learning techniques, thereby ensuring the efficiency of model training. To achieve this objective, we leverage deep convolutional networks to design a novel approach for compressing and transmitting large models. Specifically, deep convolutional networks are employed for model compression, providing an effective means to reduce the size of large models without compromising their representational capacity. The proposed framework also includes carefully devised encoding and decoding strategies to guarantee the restoration of model integrity after transmission. Furthermore, a tailored loss function is designed for model training, facilitating the optimization of both the transmission and training performance within the system. Through experimental evaluation, we demonstrate the efficacy of the proposed approach in addressing the challenges associated with large model transmission and training. The results showcase the successful compression and subsequent accurate reconstruction of large models, while maintaining their performance across various AI tasks. This work contributes to the ongoing research in enhancing the practicality and efficiency of deploying large models in real-world AI applications.
Copyright © 2023 Y. Yang et al., licensed to EAI. This is an open access article distributed under the terms of the Creative Commons Attribution license, which permits unlimited use, distribution and reproduction in any medium so long as the original work is properly cited.