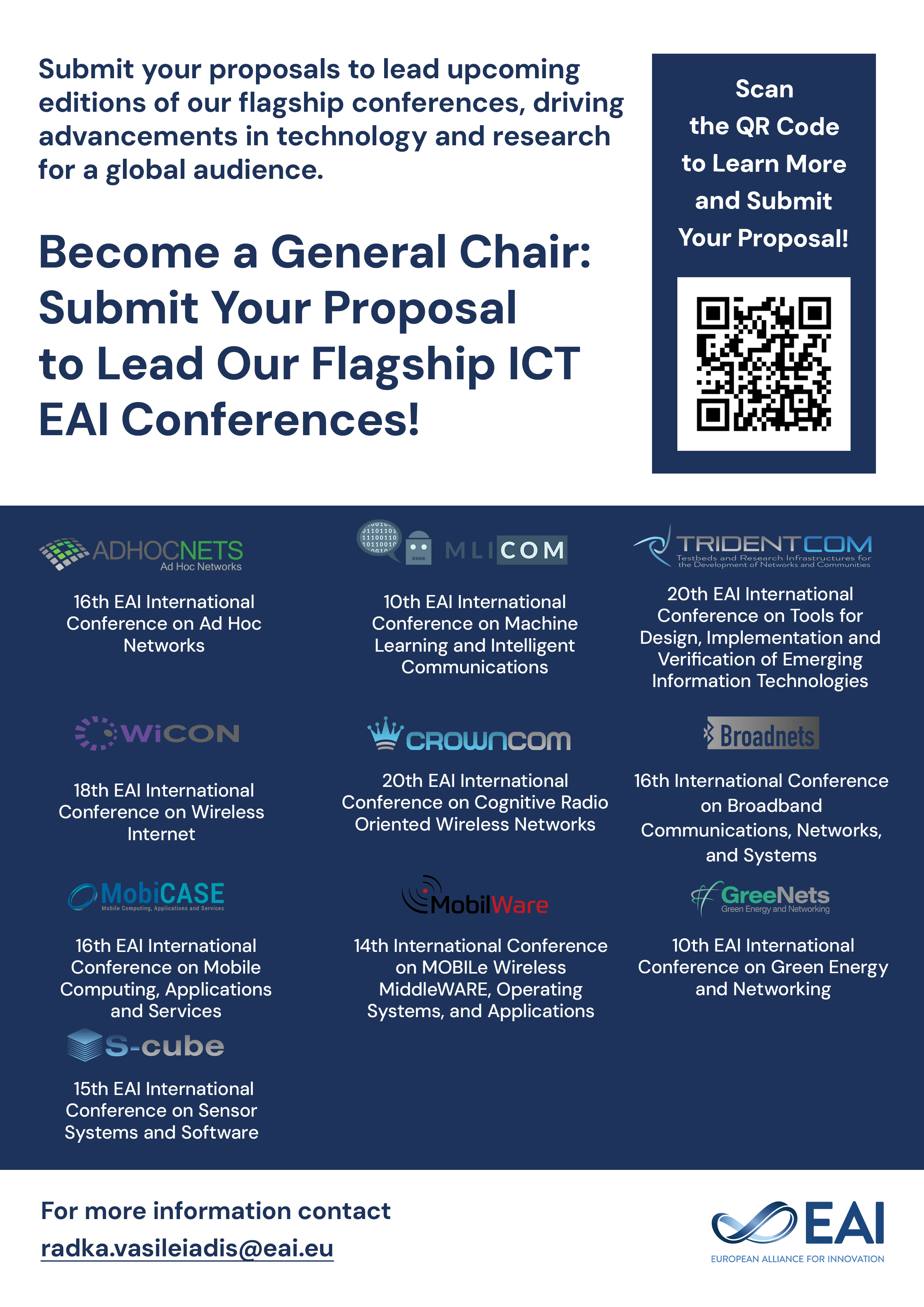
Research Article
Performance Analysis of Big Model Transmission under Double Rayleigh Fading
@ARTICLE{10.4108/eetsis.3776, author={Ying Sun and Jiajia Huang and Fusheng Wei}, title={Performance Analysis of Big Model Transmission under Double Rayleigh Fading}, journal={EAI Endorsed Transactions on Scalable Information Systems}, volume={11}, number={4}, publisher={EAI}, journal_a={SIS}, year={2023}, month={11}, keywords={Big model, data transmission, double Rayleigh fading, performance analysis}, doi={10.4108/eetsis.3776} }
- Ying Sun
Jiajia Huang
Fusheng Wei
Year: 2023
Performance Analysis of Big Model Transmission under Double Rayleigh Fading
SIS
EAI
DOI: 10.4108/eetsis.3776
Abstract
The recent big model such as GPT-3.5 possesses an extensive understanding of natural language, and it can perform a wide range of tasks, making it a significant advancement in the field of artificial intelligence (AI). A critical challenge in the design and implementation of big model is that it imposes a heavy load on the wireless transmission due to a huge size of the network parameters, especially for the distributed implementation. To tackle this challenge, we investigate big model transmission under practical double Rayleigh fading environments, where the big model is simultaneously distributed to multiple training nodes. To evaluate the system performance, we study the system outage probability (OP) based on the transmission latency, where an analytical expression is derived for the OP. Finally, we present some simulations under double Rayleigh fading environments, in order to show the validity of the proposed big model transmission.
Copyright © 2023 Ying Sun et al., licensed to EAI. This is an open access article distributed under the terms of the https://creativecommons.org/licenses/by/4.0/Creative Commons Attribution license, which permits unlimited use, distribution and reproduction in any medium so long as the original work is properly cited.