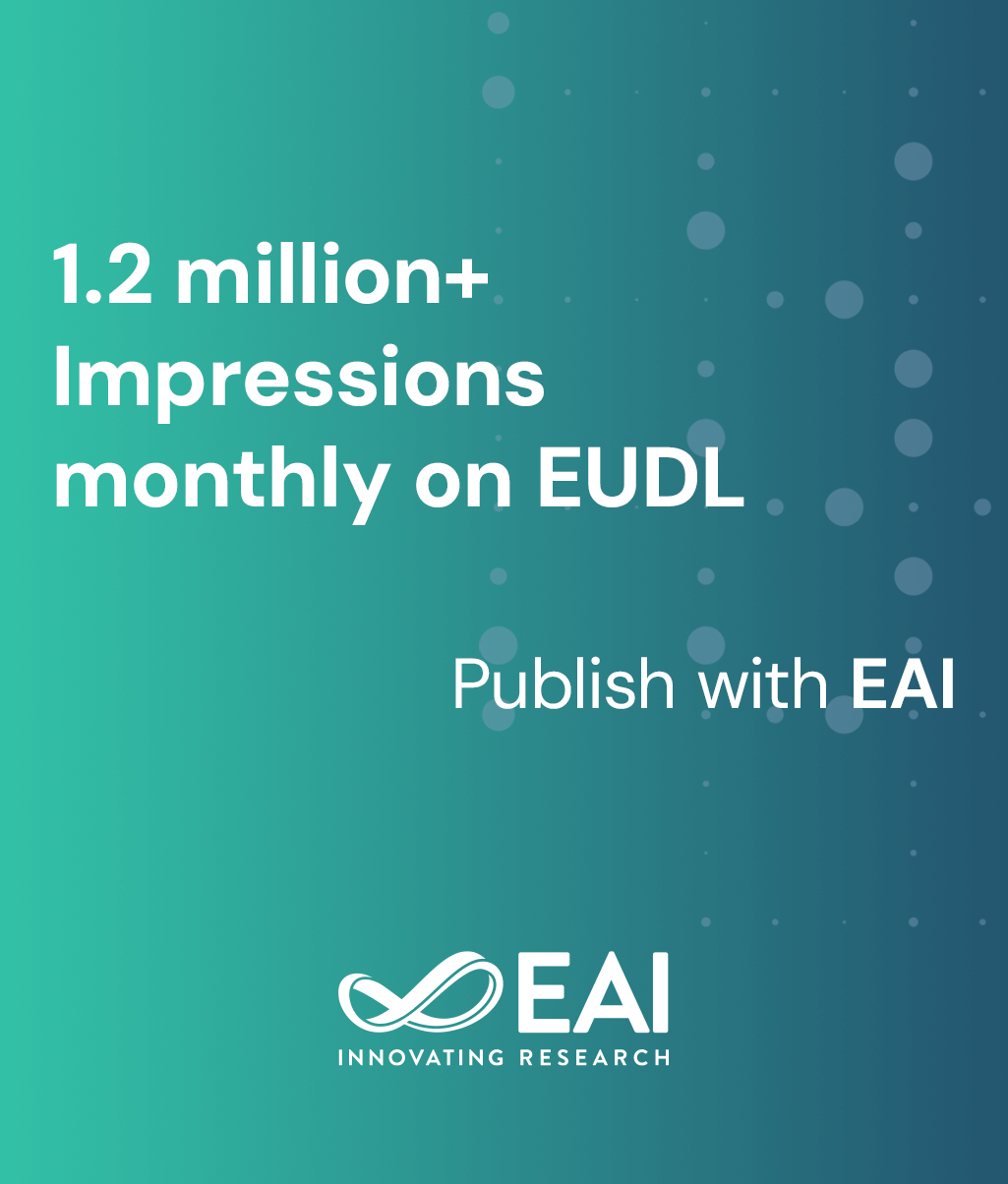
Research Article
Raspberry Pi-based Intelligent Cyber Defense Systems for SMEs and Smart-homes: An Exploratory Study
@ARTICLE{10.4108/eetsc.v6i18.2345, author={Sreenivas Sremath Tirumala and Narayan Nepal and Sayan Kumar Ray}, title={Raspberry Pi-based Intelligent Cyber Defense Systems for SMEs and Smart-homes: An Exploratory Study}, journal={EAI Endorsed Transactions on Smart Cities}, volume={6}, number={18}, publisher={EAI}, journal_a={SC}, year={2022}, month={8}, keywords={Cyber defense, Raspberry-Pi, intelligent cyber-defense system}, doi={10.4108/eetsc.v6i18.2345} }
- Sreenivas Sremath Tirumala
Narayan Nepal
Sayan Kumar Ray
Year: 2022
Raspberry Pi-based Intelligent Cyber Defense Systems for SMEs and Smart-homes: An Exploratory Study
SC
EAI
DOI: 10.4108/eetsc.v6i18.2345
Abstract
Ongoing ransomware attacks have forced business to think about security of their resources. Recently, small- to-medium enterprises (SMEs) and Smart-homes have become easy targets for attackers since they don’t have cyber defense mechanism in place other than simple firewall systems which are quite vulnerable. Cyber defense systems are costly and often not within the budget of SMEs or families which inspired to think about low cost yet highly efficient cyber defense solutions. Regular individuals and families who use internet for day to day use often end-up becoming a possible resource for using them as Trojan or bitcoin nodes. This research explores the prospects of implementing a Raspberry Pi (Raspberry Pi)-based intelligent cyber- defense system (iCDS) for SME networks and Smart-homes to filter malicious contents from incoming traffic and detect malware using artificial intelligence. Primarily, the work presented in this paper tries to evaluate the hardware capability of network interfaces (both internal, and attached) of Raspberry Pi for handle high volumes of incoming traffic. For this, we measure the network performance of the Raspberry Pi using the speed test software and try to explore the possibility of a light weight machine learning (ML) based malware detection. The results show that the built in Ethernet interface outperforms the built in WiFi and external attached USB to Ethernet Adapter in terms of latency, download and upload throughput. Also, a new DNA based ML approach was successfully able to produce over 19.5% better accuracy rates of over classifier trained with hash-sequence. The experiment results further emphasise on the importance of generating complex malware signatures with variety to face existing threats which has taken a new form due to increase in malware based attacks, particularly for ransomware. The complexity of the generated malware is based on generic yet strong encryption principles which produced good results which is quite encouraging at this stage.
Copyright © 2022 S S Tirumala et al., licensed to EAI. This is an open access article distributed under the terms of the Creative Commons Attribution license (http://creativecommons.org/licenses/by/3.0/), which permits unlimited use, distribution and reproduction in any medium so long as the original work is properly cited.