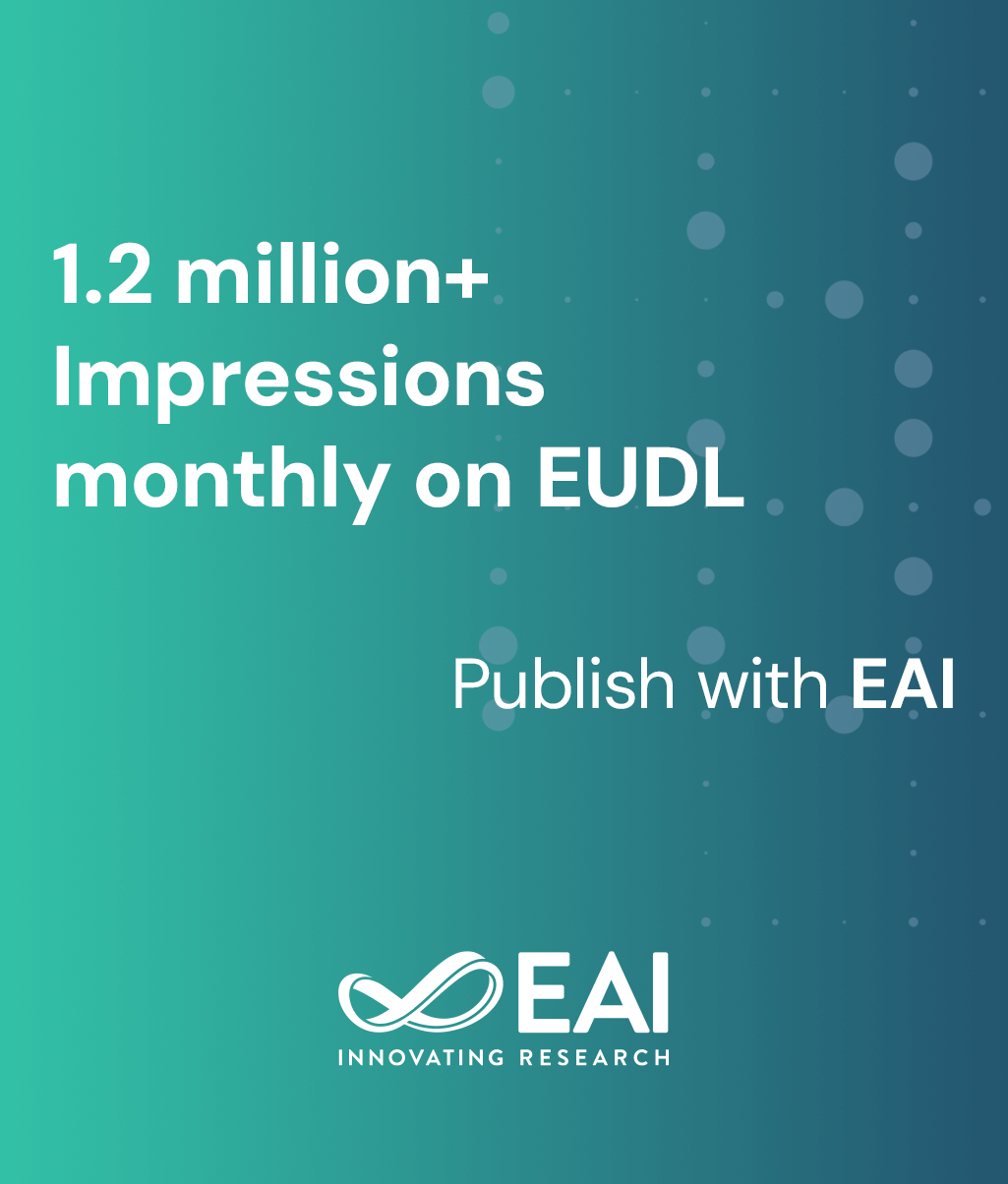
Research Article
Enhancing Document Clustering with Hybrid Recurrent Neural Networks and Autoencoders: A Robust Approach for Effective Semantic Organization of Large Textual Datasets
@ARTICLE{10.4108/eetismla.4564, author={Ratnam Dodda and Suresh Babu Alladi}, title={Enhancing Document Clustering with Hybrid Recurrent Neural Networks and Autoencoders: A Robust Approach for Effective Semantic Organization of Large Textual Datasets}, journal={EAI Endorsed Transactions on Intelligent Systems and Machine Learning}, volume={1}, number={1}, publisher={EAI}, journal_a={ISMLA}, year={2024}, month={3}, keywords={Document Clustering, Recurrent Neural Network, Autoencoders, Hybrid model, Diverse Datasets}, doi={10.4108/eetismla.4564} }
- Ratnam Dodda
Suresh Babu Alladi
Year: 2024
Enhancing Document Clustering with Hybrid Recurrent Neural Networks and Autoencoders: A Robust Approach for Effective Semantic Organization of Large Textual Datasets
ISMLA
EAI
DOI: 10.4108/eetismla.4564
Abstract
This research presents an innovative document clustering method that uses recurrent neural networks (RNNs) and autoencoders. RNNs capture sequential dependencies while autoencoders improve feature representation. The hybrid model, tested on different datasets (20-Newsgroup, Reuters, BBC Sports), outperforms traditional clustering, revealing semantic relationships and robustness to noise. Preprocessing includes denoising techniques (stemming, lemmatization, tokenization, stopword removal) to ensure a refined data set. Evaluation metrics (adjusted randomness evaluation, normalized mutual information evaluation, completeness evaluation, homogeneity evaluation, V-measure, accuracy) validate the effectiveness of the model and provide a powerful solution for organizing and understanding large text datasets.
Copyright © 2024 R. Dodda et al., licensed to EAI. This is an open access article distributed under the terms of the CC BY-NC-SA 4.0, which permits copying, redistributing, remixing, transformation, and building upon the material in any medium so long as the original work is properly cited.