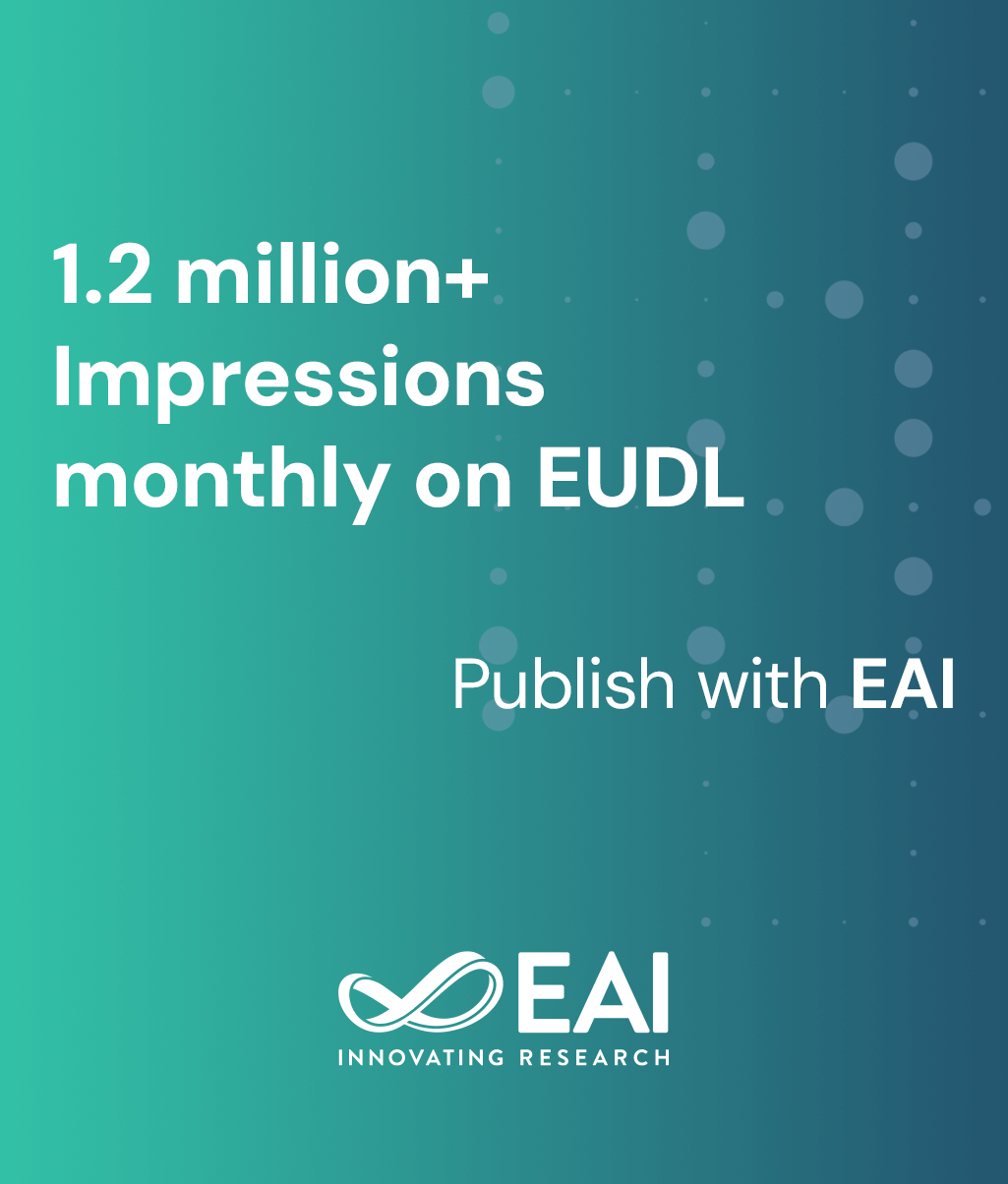
Research Article
Robustness of NMF Algorithms Under Different Noises
@ARTICLE{10.4108/eetiot.v9i1.3271, author={Mengyao Kang and Jiawei Zhao and Zheng Han}, title={Robustness of NMF Algorithms Under Different Noises}, journal={EAI Endorsed Transactions on Internet of Things}, volume={9}, number={1}, publisher={EAI}, journal_a={IOT}, year={2023}, month={6}, keywords={Machine Learning, Nonnegative matrix factorization, Robustness of algorithm, NMF}, doi={10.4108/eetiot.v9i1.3271} }
- Mengyao Kang
Jiawei Zhao
Zheng Han
Year: 2023
Robustness of NMF Algorithms Under Different Noises
IOT
EAI
DOI: 10.4108/eetiot.v9i1.3271
Abstract
In machine learning, datasets are often disturbed by different noises. The Nonnegative Matrix Factorization (NMF) algorithm provides a robust method to deal with noise, which will significantly improve the efficiency of machine learning. In this investigation, the standard NMF algorithm and L2,1-Norm Based NMF algorithm are studied by designing experiments on different noise types, noise levels, and datasets. Furthermore, Relative Reconstruction Errors (RRE), accuracy, and Normalized Mutual Information (NMI) are used to evaluate the robustness of the two algorithms. In this experiment, there is no significant difference in performance between the two algorithms, while L2,1-Norm Based NMF algorithm shows relatively small advantages.
Copyright © 2023 Mengyao Kang et al., licensed to EAI. This is an open access article distributed under the terms of the CC BY-NCSA 4.0, which permits copying, redistributing, remixing, transformation, and building upon the material in any medium so long as the original work is properly cited.