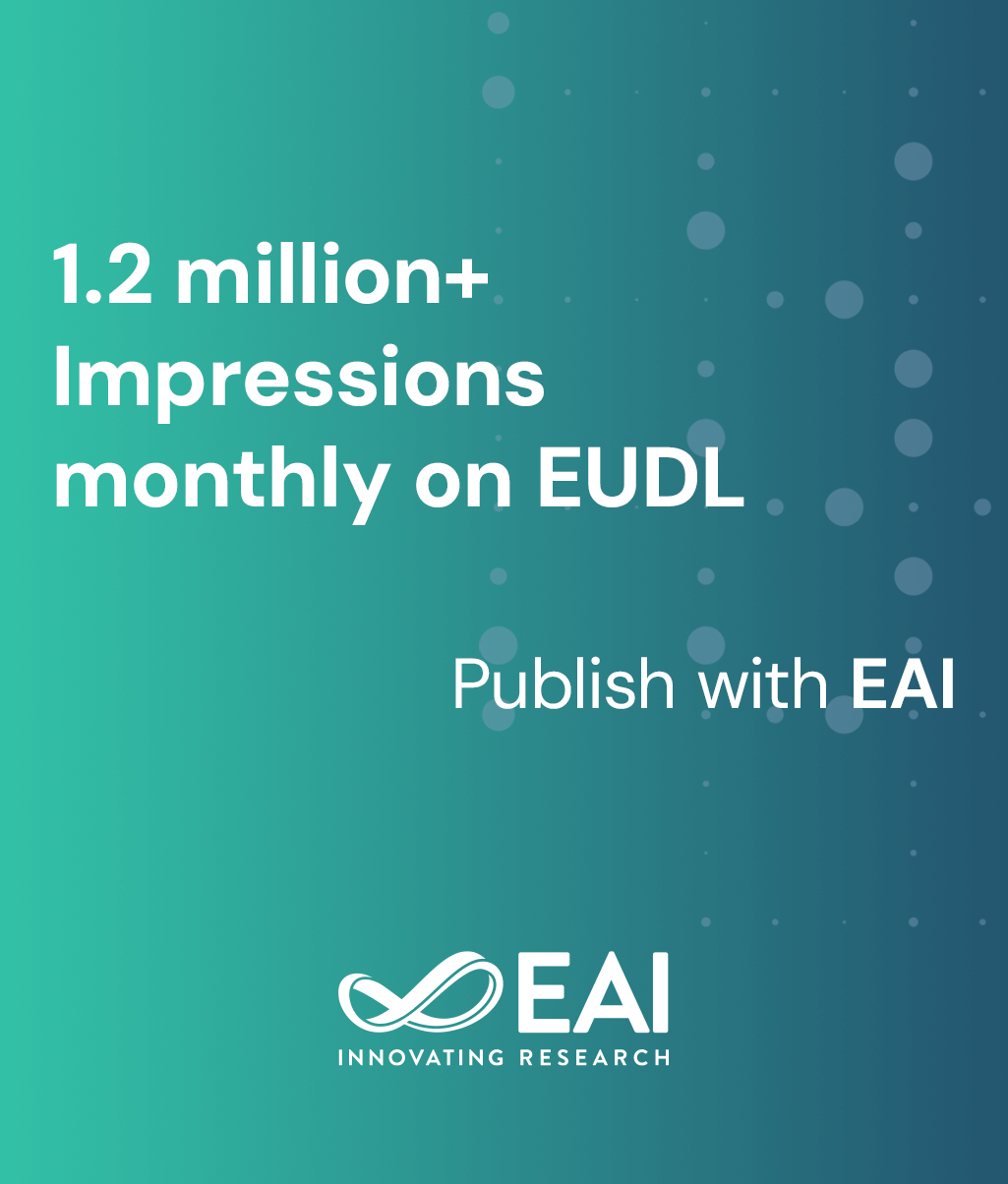
Research Article
Smartagb: Aboveground Biomass Estimation of Sorghum Based on Spatial Resolution, Machine Learning and Vegetation Index
@ARTICLE{10.4108/eetiot.v9i1.2904, author={Qi Liu and Yaxin Wang and Jie Yang and Wuping Zhang and Huanchen Wang and Fuzhong Li and Guofang Wang and Yuansen Huo and Jiwan Han}, title={Smartagb: Aboveground Biomass Estimation of Sorghum Based on Spatial Resolution, Machine Learning and Vegetation Index}, journal={EAI Endorsed Transactions on Internet of Things}, volume={9}, number={1}, publisher={EAI}, journal_a={IOT}, year={2023}, month={3}, keywords={Sorghum, Aboveground Biomass, UAV, Multispectral Image, Spatial Resolution}, doi={10.4108/eetiot.v9i1.2904} }
- Qi Liu
Yaxin Wang
Jie Yang
Wuping Zhang
Huanchen Wang
Fuzhong Li
Guofang Wang
Yuansen Huo
Jiwan Han
Year: 2023
Smartagb: Aboveground Biomass Estimation of Sorghum Based on Spatial Resolution, Machine Learning and Vegetation Index
IOT
EAI
DOI: 10.4108/eetiot.v9i1.2904
Abstract
This work aims to explore the feasibility of predicting and estimating the aboveground biomass (AGB) of sorghum using multispectral images captured by UAVs, and clarify the quantitative relationship between vegetation index and sorghum AGB based on different spatial resolutions, and build an AGB estimation model based on UAV multispectral images and vegetation index under different spatial resolutions. Combining spatial resolution, vegetation index, and machine learning, a training set is used to train the model, and a verification set is used to verify the model to select the best prediction model corresponding to different spatial resolutions. The three best prediction models under three spatial resolutions are classic machine learning models. 1) when the spatial resolution is 0.017m, the model precision obtained from the random forest is R2=0.8961, MAE=26.4340, and RMSE=32.2459. 2) when the spatial resolution is 0.024m, the model accuracy obtained by the Lasso algorithm is R2=0.8826, MAE=31.106, and RMSE=40.2937; 3) when the spatial resolution is 0.030m, the model accuracy obtained by the decision tree algorithm is R2=0.8568, MAE=30.3373, and RMSE=40.8082; and 4) the model's accuracy decreases with the decrease of spatial resolution. The results show that the combination of spatial resolution, vegetation index, and machine learning algorithm is an effective, fast, and accurate prediction method.
Copyright © 2023 Qi Liu et al., licensed to EAI. This is an open access article distributed under the terms of the CC BY-NC-SA 4.0, which permits copying, redistributing, remixing, transformation, and building upon the material in any medium so long as the original work is properly cited.