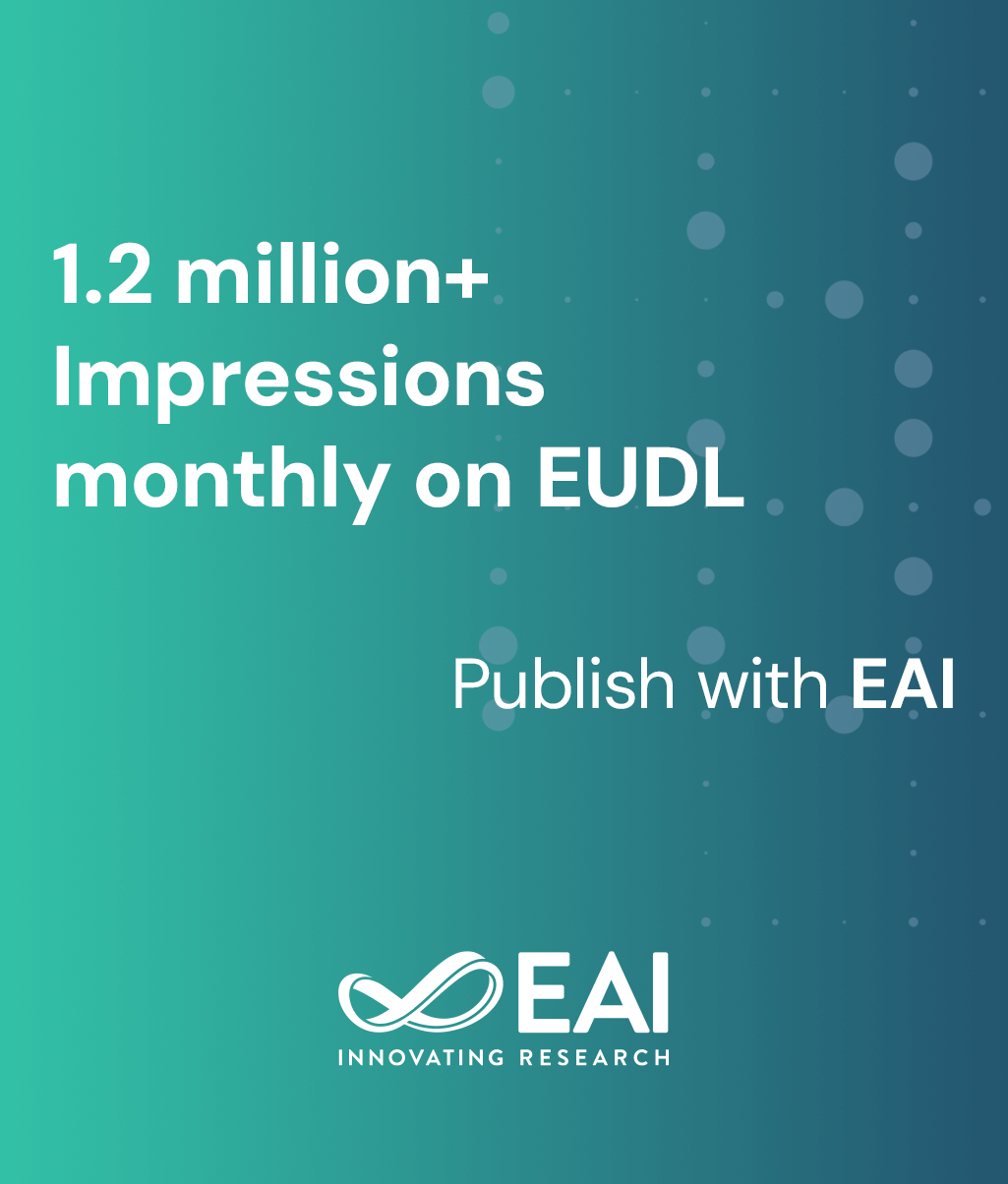
Research Article
Data prediction system in malaria control based on physio-chemical parameters of anopheles breeding sites
@ARTICLE{10.4108/eetiot.v8i4.2936, author={Kodzo M. Parkoo and Bamba Gueye and Cheikh Sarr and Ibrahima Dia}, title={Data prediction system in malaria control based on physio-chemical parameters of anopheles breeding sites}, journal={EAI Endorsed Transactions on Internet of Things}, volume={8}, number={4}, publisher={EAI}, journal_a={IOT}, year={2022}, month={12}, keywords={algorithms classification, larvae control, data analysis, data predictions, malaria, python}, doi={10.4108/eetiot.v8i4.2936} }
- Kodzo M. Parkoo
Bamba Gueye
Cheikh Sarr
Ibrahima Dia
Year: 2022
Data prediction system in malaria control based on physio-chemical parameters of anopheles breeding sites
IOT
EAI
DOI: 10.4108/eetiot.v8i4.2936
Abstract
Malaria is a public health problem in Senegal. As a result, a real program focused on prevention and treatment has been put in place to fight it. Despite the efforts made, the prevalence rate of malaria is still worrying. To have a prediction system that, once certain physicochemical information, will inform if we can or not attend to the development of anopheles larvae. Our work consisted of collecting data on mosquito breeding sites, processing, and analyzing them in order to predict the physicochemical conditions for the development of Anopheles larvae. Larval control is an alternative to reduce the prevalence rate of malaria. We retain logistic regression as an algorithm and water electrical conductivity, water turbidity, temperature, and dissolved oxygen as determinant parameters. The learning and prediction system set up on the basis of the determining parameters and logistic regression worked. The predictions will be improved by further training our system with field data.
Copyright © 2022 Kodzo M. Parkoo et al., licensed to EAI. This is an open access article distributed under the terms of the CC BYNC-SA 4.0, which permits copying, redistributing, remixing, transformation, and building upon the material in any medium so long as the original work is properly cited.