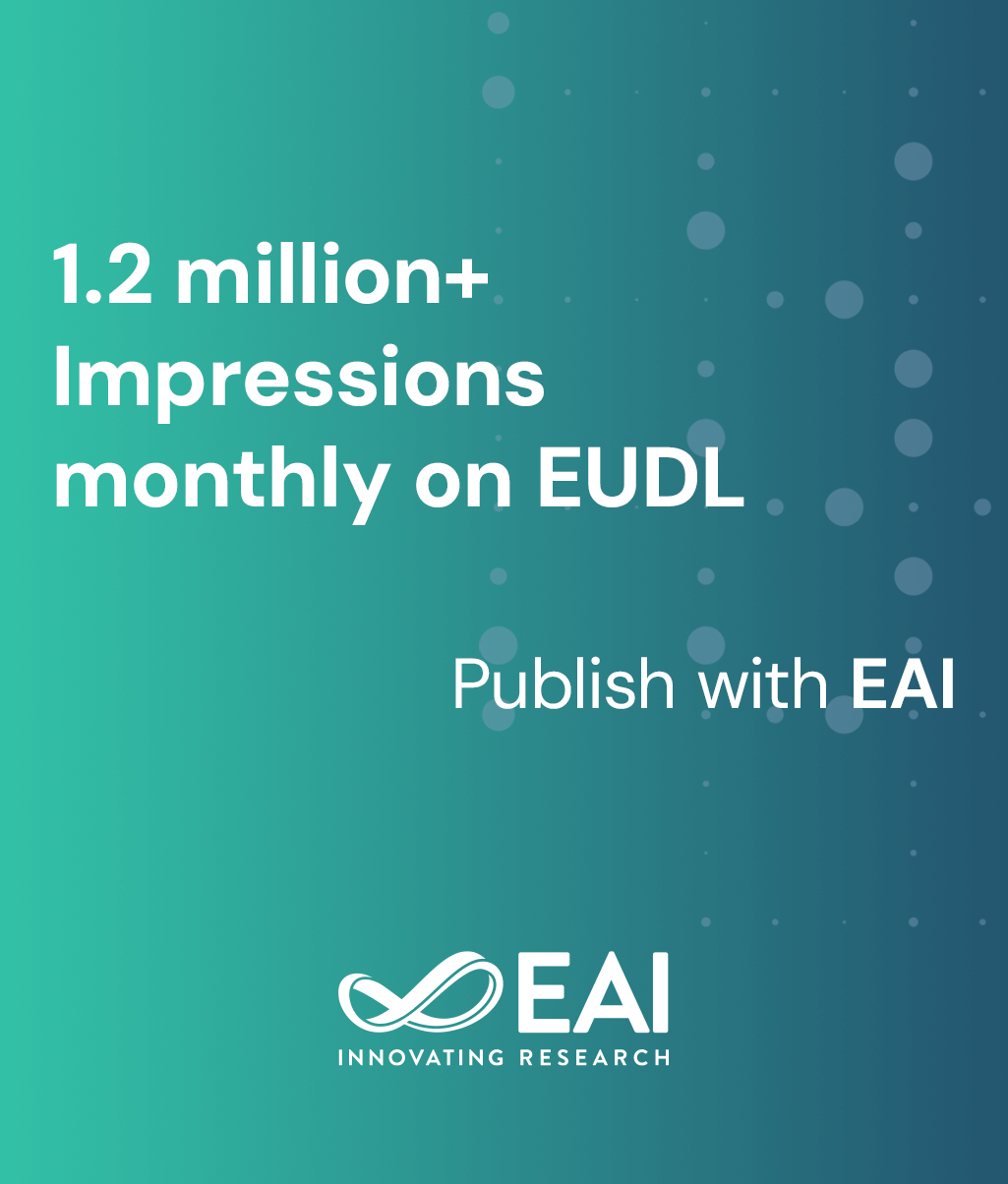
Research Article
Smart Gardening: A Solution to Your Gardening Issues
@ARTICLE{10.4108/eetiot.v8i30.384, author={Niloy Chakraborty and Adrika Mukherjee and Mayuri Bhadra}, title={Smart Gardening: A Solution to Your Gardening Issues}, journal={EAI Endorsed Transactions on Internet of Things}, volume={8}, number={30}, publisher={EAI}, journal_a={IOT}, year={2022}, month={8}, keywords={smart garden, AI planning, plant identification, CNN, smart lighting, Internet of Things, smart city}, doi={10.4108/eetiot.v8i30.384} }
- Niloy Chakraborty
Adrika Mukherjee
Mayuri Bhadra
Year: 2022
Smart Gardening: A Solution to Your Gardening Issues
IOT
EAI
DOI: 10.4108/eetiot.v8i30.384
Abstract
The technology which could make our lives prosper within the four walls could also help to create our own corner of nature nourish. In this paper, we propose a smart gardening system that utilizes the concept of the Internet of Things (IoT) [1]. The major goal of this project is to reduce water consumption when gardening and to maintain the garden remotely. Important plant data, like temperature, relative humidity, and soil moisture, are continuously stored in a relational database in this gardening system. Artificial Intelligence (AI) based planning [2] is used for watering the plants at regular intervals and providing appropriate illumination in the garden area for aesthetics and overall plant growth. Our proposed system reduces the effort due to manual intervention by around 59.3%. The real-time sensor status can be monitored which in turn allows the end-users of the garden to control the surrounding conditions optimal for plant growth, using the Telegram application. A plant recognition model has been introduced in this system, where a Convolutional Neural Network (CNN) [3] based deep learning algorithm classifies the plant categories with 95% accuracy. Moreover, an 98% accurate, deep learning-based, plant health identification model integrated with this gardening system also informs the end-user about the health of the plant.
Copyright © 2022 Niloy Chakraborty et al., licensed to EAI. This is an open access article distributed under the terms of the Creative Commons Attribution license, which permits unlimited use, distribution and reproduction in any medium so long as the original work is properly cited.