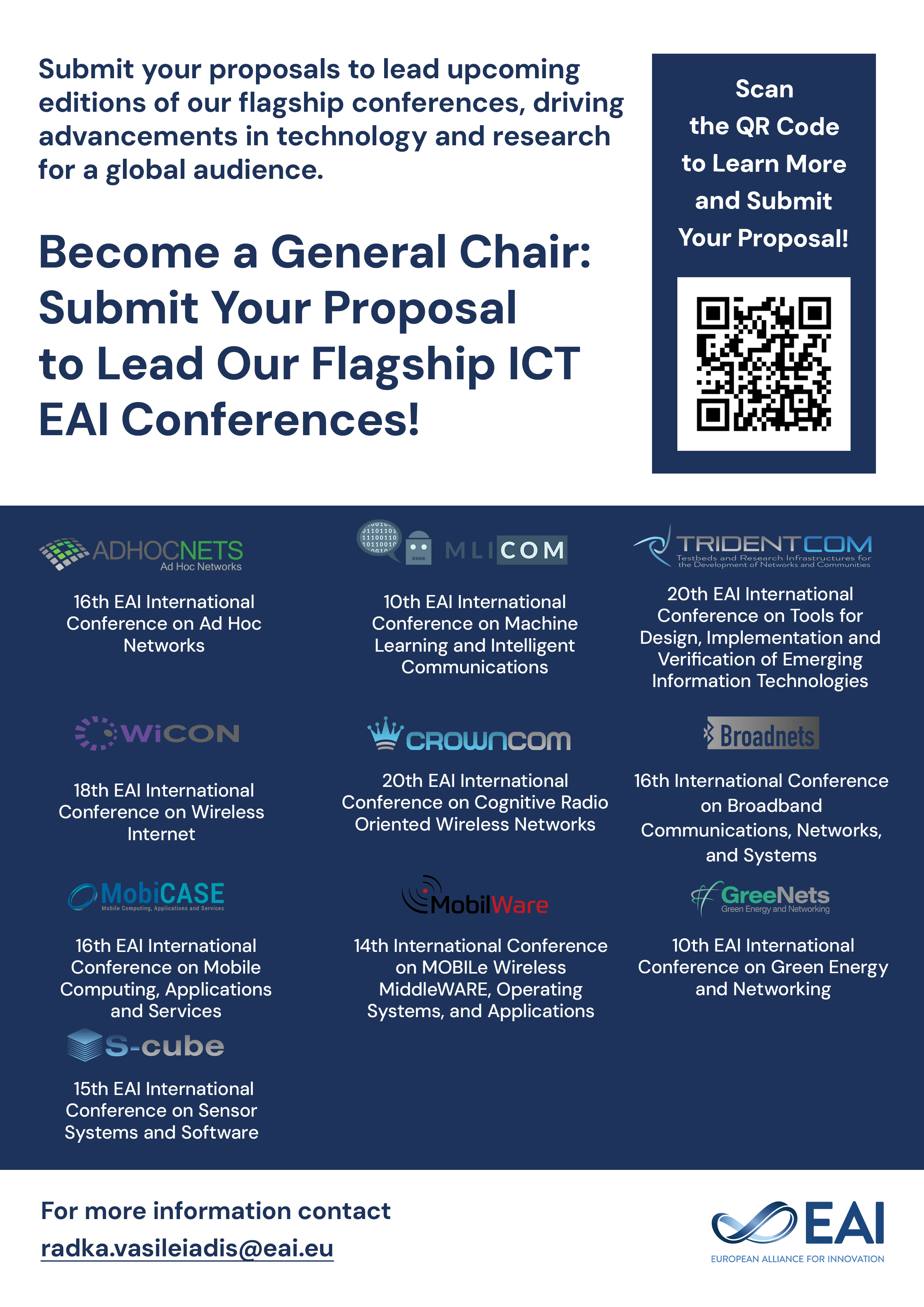
Research Article
Detection of Sleep Paralysis by using IoT Based Device and Its Relationship Between Sleep Paralysis And Sleep Quality
@ARTICLE{10.4108/eetiot.v8i30.2688, author={Muhammad Shoaib Akhtar and Tao Feng}, title={Detection of Sleep Paralysis by using IoT Based Device and Its Relationship Between Sleep Paralysis And Sleep Quality}, journal={EAI Endorsed Transactions on Internet of Things}, volume={8}, number={30}, publisher={EAI}, journal_a={IOT}, year={2022}, month={9}, keywords={Sleep paralysis, Machine Learning, Deep Learning}, doi={10.4108/eetiot.v8i30.2688} }
- Muhammad Shoaib Akhtar
Tao Feng
Year: 2022
Detection of Sleep Paralysis by using IoT Based Device and Its Relationship Between Sleep Paralysis And Sleep Quality
IOT
EAI
DOI: 10.4108/eetiot.v8i30.2688
Abstract
When a person wakes up in the middle of the night, they are paralyzed. Despite the fact that most episodes are associated with extreme terror and some might cause clinically significant suffering, little is understood about the experience. This study will analyze existing research on the relationship between sleep paralyses and sleep in general. Many studies have connected poor sleep quality to an increased risk of sleep paralysis. Awake yet unable to act, sleep paralysis occurs. This might happen between awake and sleeping. The problem is approached in three steps: Data collection, data storage, calculation and machine learning prediction of sleep paralysis. The data came from the Smart Device. The dataset has several (independent) and dependent variables (Outcome). This device has been put to the test. Each exam has its own set of features and predicted outcomes. To assess the system's validity, we executed a posture recognition accuracy test. The device was hidden on top of the bed. The controller is in charge of measurement and data collection. Experiments were conducted by collecting pressure data from a patient lying down. The person acted out his sleeping positions on a mat for a while. Machine learning has been used to predict sleep paralysis. By comparing sleep postures to the outcome, we were able to show the link between sleep qualities and sleep paralysis. Machine learning approaches have been used to predict sleep paralysis. Comparing sleeping positions with the results showed the link between sleep quality and sleep paralysis. Sleep paralysis correlates with poor sleep quality. The Random Forest model has the highest accuracy of 91.9 percent in predicting sleep paralysis in the given dataset. SVM with Linear Kernel was 80.49 percent accurate, RBF was 42.68 percent, and Polynomial was 47.56 percent. The accuracy of logistic regression was 76.83 percent. KNN had a dismal performance of 60.98%. Decision Trees and Gradient Boosting both fared well at 85.37 percent.
Copyright © 2022 Muhammad Shoaib Akhtar et al., licensed to EAI. This is an open access article distributed under the terms of the CC BY-NC-SA 4.0, which permits copying, redistributing, remixing, transformation, and building upon the material in any medium so long as the original work is properly cited.