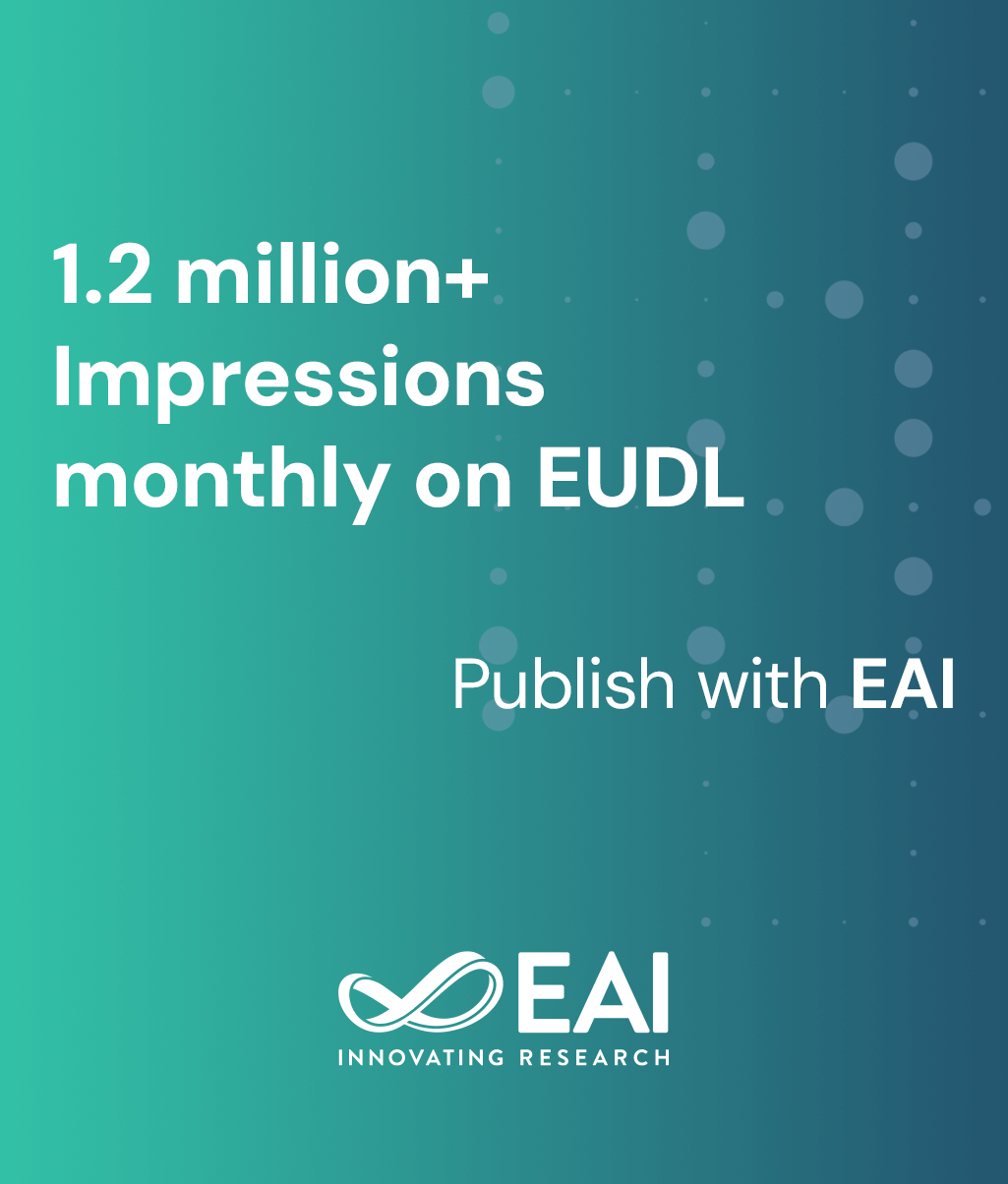
Research Article
Comparative Study on Anomaly based Intrusion Detection using Deep Learning Techniques
@ARTICLE{10.4108/eetiot.7178, author={Sabeena S and Chitra S}, title={Comparative Study on Anomaly based Intrusion Detection using Deep Learning Techniques}, journal={EAI Endorsed Transactions on Internet of Things}, volume={11}, number={1}, publisher={EAI}, journal_a={IOT}, year={2024}, month={11}, keywords={Wireless Sensor Network, Malicious Attacks, Intrusion Detection Systems, Machine Learning, Deep Learning}, doi={10.4108/eetiot.7178} }
- Sabeena S
Chitra S
Year: 2024
Comparative Study on Anomaly based Intrusion Detection using Deep Learning Techniques
IOT
EAI
DOI: 10.4108/eetiot.7178
Abstract
With an array of applications, Wireless Sensor Networks (WSNs) have the potential to transform the world into a smart planet. WSNs consist of a collection of resource-constrained sensors that gather data, which is then utilized for decision-making and analysis, leading to improvements in quality of service, management, and efficiency. However, the open nature of WSNs exposes them to numerous vulnerabilities and threats. Operating in potentially hostile and unattended environments makes these networks attractive targets for adversaries. Therefore, it is essential to detect the presence of malicious attacks within the networks and implement robust security systems to address these challenges. While traditional security mechanisms such as authentication and cryptographic methods are commonly employed, they often fall short in effectively countering the dynamic nature of modern attacks. Hence, IDS (Intrusion Detection System) tends to continuously monitor the network and detect potential threats in real-time scenarios. This method possess the ability of identifying, responding promptly, preventing and thus ensures resilience of the network. Therefore, the present study reviews the various intrusion detection techniques and data collection methods. The main aim of the study is to investigate the design challenges of deploying IDS in a WSN environment. So, the study analysed the AI (Artificial Intelligence) based techniques involved in intrusion detection and how these techniques could be adopted in WSN. In addition, the comparative analysis of several ML (Machine Learning) and DL (Deep Learning) algorithms are also deliberated to portray the different deployment technique with corresponding outcomes. Further, the main challenges faced by each studies with their limitations are specified for supporting future researchers in developing new trends in intrusion detection for WSN.
Copyright © 2024 Sabeena S et al., licensed to EAI. This is an open access article distributed under the terms of the CC BY-NC-SA 4.0, which permits copying, redistributing, remixing, transformation, and building upon the material in any medium so long as the original work is properly cited.