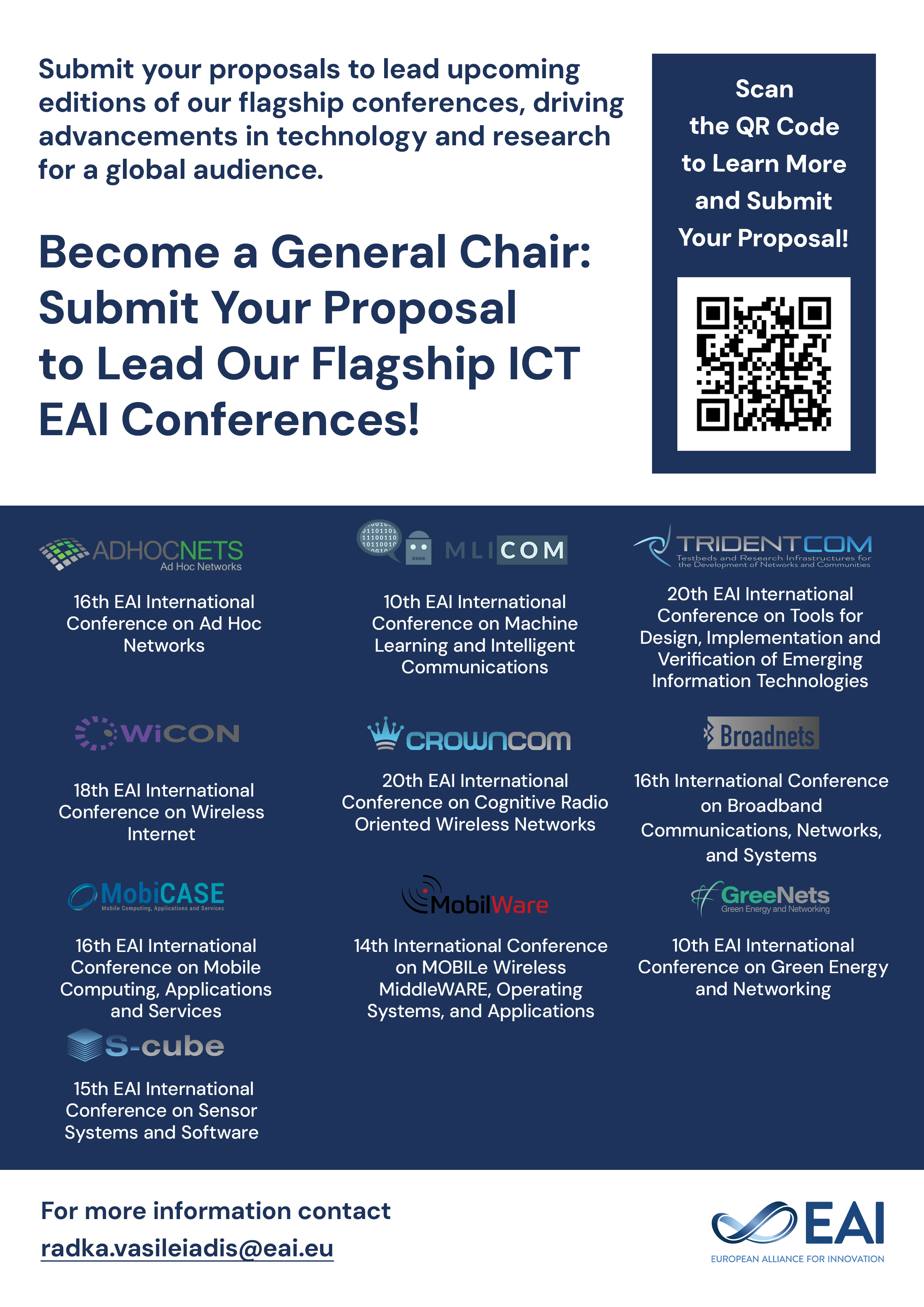
Editorial
Detection of Anomalous Bitcoin Transactions in Blockchain Using ML
@ARTICLE{10.4108/eetiot.7042, author={Soumya Bajpai and Kapil Sharma and Brijesh Kumar Chaurasia}, title={Detection of Anomalous Bitcoin Transactions in Blockchain Using ML}, journal={EAI Endorsed Transactions on Internet of Things}, volume={10}, number={1}, publisher={EAI}, journal_a={IOT}, year={2024}, month={8}, keywords={Machine learning (ML), Regressor Model, Blockchain, Bitcoin Prediction, IoT}, doi={10.4108/eetiot.7042} }
- Soumya Bajpai
Kapil Sharma
Brijesh Kumar Chaurasia
Year: 2024
Detection of Anomalous Bitcoin Transactions in Blockchain Using ML
IOT
EAI
DOI: 10.4108/eetiot.7042
Abstract
An Internet of Things (IoT)-enabled blockchain helps to ensure quick and efficient immutable transactions. Low-power IoT integration with the Bitcoin network has created new opportunities and difficulties for blockchain transactions. Utilising data gathered from IoT-enabled devices, this study investigates the application of ML regression models to analyse and forecast Bitcoin transaction patterns. Several ML regression algorithms, including Lasso Regression, Gradient Boosting, Extreme Boosting, Extra Tree, and Random Forest Regression, are employed to build predictive models. These models are trained using historical Bitcoin transaction data to capture intricate relationships between various transaction parameters. To ensure model robustness and generalisation, cross-validation techniques and hyperparameter tuning are also applied. The empirical results show that the Bitcoin cost prediction of blockchain transactions in terms of time series. Additionally, it highlights the possibility of fusing block- chain analytics with IoT data streams, illuminating how new technologies might work together to enhance financial institutions.
Copyright © 2024 Brijesh Kumar Chaurasiaet al., licensed to EAI. This is an open access article distributed under the terms of the CC BY-NC-SA 4.0, which permits copying, redistributing, remixing, transformation, and building upon the material in any medium so long as the original work is properly cited.