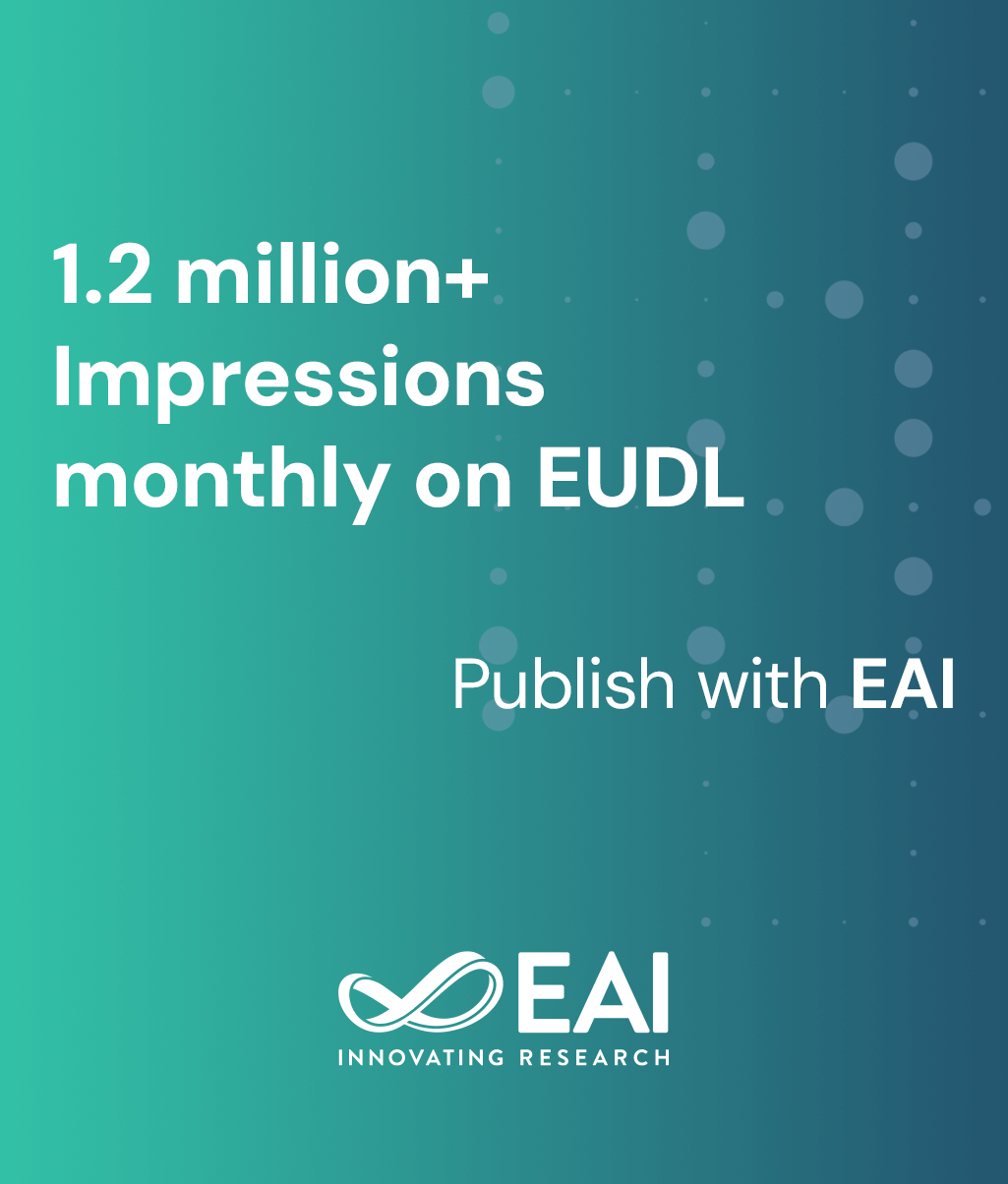
Research Article
Enhancing User Query Comprehension and Contextual Relevance with a Semantic Search Engine using BERT and ElasticSearch
@ARTICLE{10.4108/eetiot.6993, author={Saniya M ladanavar and Ritu Kamble and R.H Goudar and Rohit. B. Kaliwal and Vijayalaxmi Rathod and Santhosh L Deshpande and Dhananjaya G M and Anjanabhargavi Kulkarni}, title={Enhancing User Query Comprehension and Contextual Relevance with a Semantic Search Engine using BERT and ElasticSearch}, journal={EAI Endorsed Transactions on Internet of Things}, volume={10}, number={1}, publisher={EAI}, journal_a={IOT}, year={2024}, month={12}, keywords={Semantic Search Engine, Elastic Search, BERT Model (SBERT)}, doi={10.4108/eetiot.6993} }
- Saniya M ladanavar
Ritu Kamble
R.H Goudar
Rohit. B. Kaliwal
Vijayalaxmi Rathod
Santhosh L Deshpande
Dhananjaya G M
Anjanabhargavi Kulkarni
Year: 2024
Enhancing User Query Comprehension and Contextual Relevance with a Semantic Search Engine using BERT and ElasticSearch
IOT
EAI
DOI: 10.4108/eetiot.6993
Abstract
This research paper explores the development of a semantic search engine designed to enhance user query comprehension and deliver contextually applicable research results. Classic search engines basically struggle to catch the nuanced meaning of user queries, giving to suboptimal results. To address this challenge, we give the merge of advanced natural language processing (NLP) techniques with Elasticsearch, and with a specific focus on Bidirectional Encoder Representations from Transformers (BERT), a state-of-the-art pre-trained language model. Our approach involves leveraging BERT's ability to analyze the contextual meaning of words within documents by sentence transformers as (SBERT) , enabling the search engine to grab the user queries and better under- stand semantics of the content as it is converted into vector embeddings making it understandable in the Elasticsearch server. By utilizing BERT's bidirectional attention mechanism, the search engine can discern the relationships between words, thereby capturing the contextual nuances that are crucial for accurate query interpretation. Through experimental validation and performance assessments, we demonstrate the efficacy of our semantic search engine in providing contextually relevant search results. This research contributes to the advancement of search technology by enhancing the intelligence of search engines, ultimately improving the user experience by giving context based research.
Copyright © 2024 R. H. Goundar et al., licensed to EAI. This is an open access article distributed under the terms of the CC BY-NCSA 4.0, which permits copying, redistributing, remixing, transformation, and building upon the material in any medium so long as the original work is properly cited.