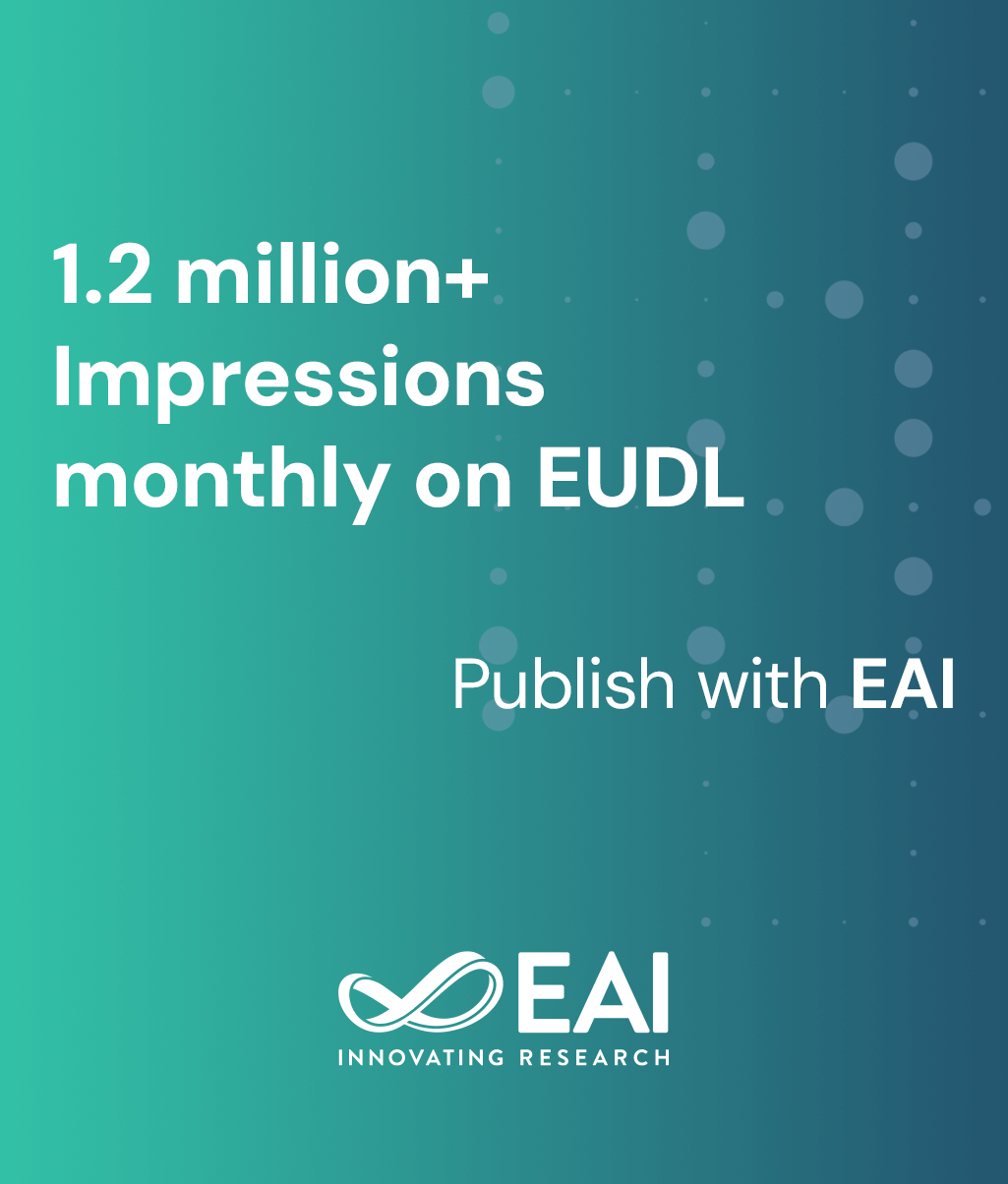
Research Article
A Machine Learning-Based Method for Predicting the Classification of Aircraft Damage
@ARTICLE{10.4108/eetiot.6936, author={Imron Rosadi and Freddy Franciscus and Muhammad Hadi Widanto}, title={A Machine Learning-Based Method for Predicting the Classification of Aircraft Damage}, journal={EAI Endorsed Transactions on Internet of Things}, volume={10}, number={1}, publisher={EAI}, journal_a={IOT}, year={2024}, month={12}, keywords={Aircraft Damage Classification, Machine Learning, Predictive Maintenance, Structural Assessment, Aviation Safety, Maintenance Planning}, doi={10.4108/eetiot.6936} }
- Imron Rosadi
Freddy Franciscus
Muhammad Hadi Widanto
Year: 2024
A Machine Learning-Based Method for Predicting the Classification of Aircraft Damage
IOT
EAI
DOI: 10.4108/eetiot.6936
Abstract
Efficient and accurate classification of aircraft damage is paramount in ensuring the safety and reliability of air transportation. This research uses a machine learning-based approach tailored to predict the classification of aircraft damage with high precision and reliability to achieve data-driven insights as input for the improvement of safety standards. Leveraging a diverse dataset encompassing various types and severities of damage instances, our methodology harnesses the power of machine learning algorithms to discern patterns and correlations within the data. The approach involves using extensive datasets consisting of various structural attributes, flight data, and environmental conditions. The Random Forest algorithm, Support Vector Machine, and Neural Networks methods used in the research are more accurate than traditional methods, providing detailed information on the factors contributing to damage severity. By using machine learning, maintenance schedules can be optimized and flight safety can be improved. This research is a significant step toward predictive maintenance, which is poised to improve safety standards in the aerospace industry.
Copyright © 2024 Rosadi et al., licensed to EAI. This is an open access article distributed under the terms of the CC BY-NC-SA 4.0, which permits copying, redistributing, remixing, transformation, and building upon the material in any medium so long as the original work is properly cited.