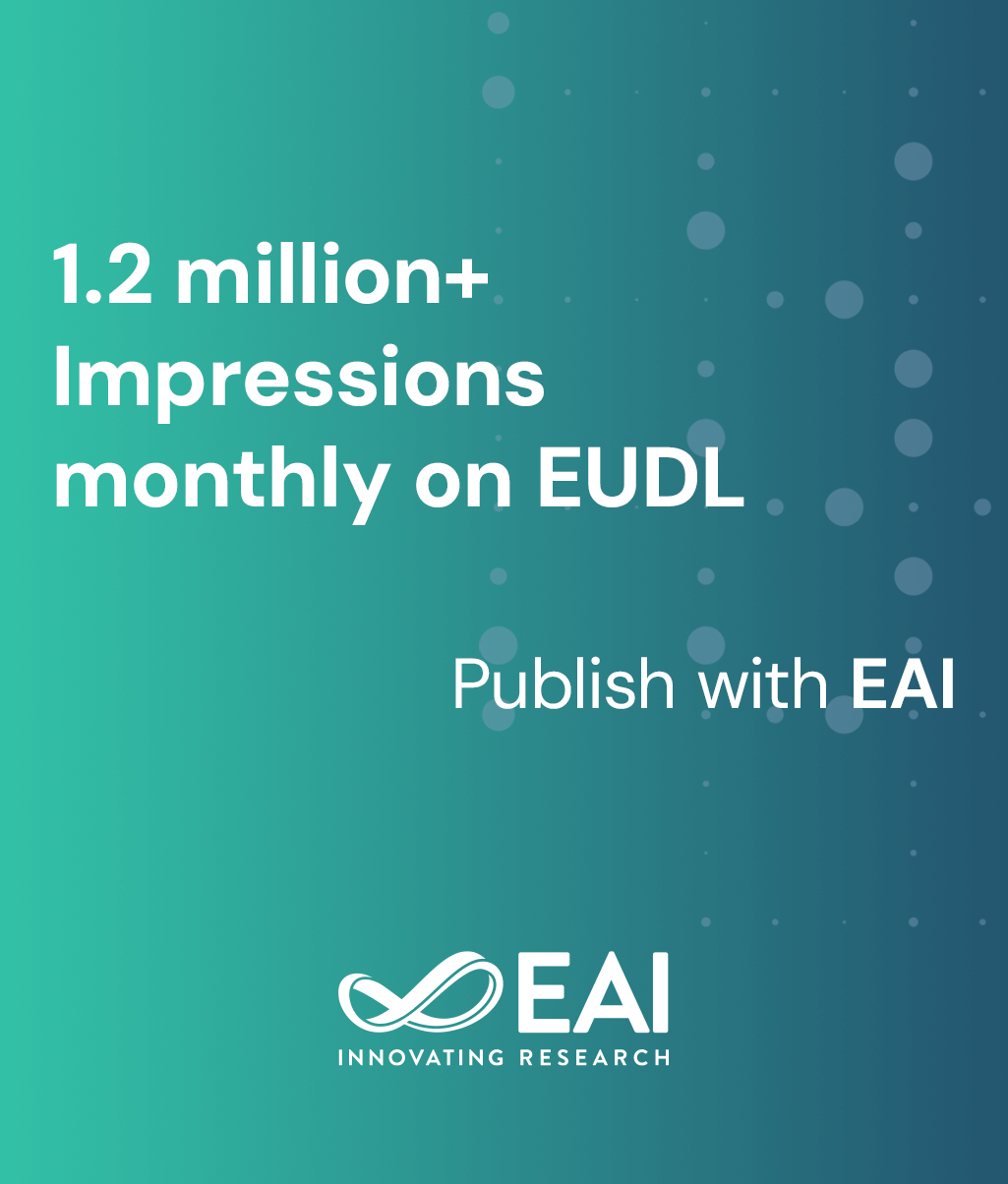
Editorial
A Probabilistic Descent Ensemble for Malware Prediction Using Deep Learning
@ARTICLE{10.4108/eetiot.6774, author={R. Vinoth Kumar and R. Suguna}, title={A Probabilistic Descent Ensemble for Malware Prediction Using Deep Learning}, journal={EAI Endorsed Transactions on Internet of Things}, volume={10}, number={1}, publisher={EAI}, journal_a={IOT}, year={2024}, month={12}, keywords={Gaussian Naive Bayes, Stochastic Gradient Descent, Maximum Likelihood Estimation, Hyperparameters, Mini-Batch Gradient Descent}, doi={10.4108/eetiot.6774} }
- R. Vinoth Kumar
R. Suguna
Year: 2024
A Probabilistic Descent Ensemble for Malware Prediction Using Deep Learning
IOT
EAI
DOI: 10.4108/eetiot.6774
Abstract
INTRODUCTION: Introducing a Probabilistic Descent Ensemble (PDE) approach for enhancing malware prediction through deep learning leverages the power of multiple neural network models with distinct architectures and training strategies to achieve superior accuracy while minimizing false positives. OBJECTIVES: Combining Stochastic Gradient Descent (SGD) with early stopping is a potent approach to optimising deep learning model training. Early stopping, a vital component, monitors a validation metric and halts training if it stops improving or degrades, guarding against overfitting. METHODS: This synergy between SGD and early stopping creates a dynamic framework for achieving optimal model performance adaptable to diverse tasks and datasets, with potential benefits including reduced training time and enhanced generalization capabilities. RESULTS: The proposed work involves training a Gaussian NB classifier with SGD as the optimization algorithm. Gaussian NB is a probabilistic classifier that assumes the features follow a Gaussian (normal) distribution. SGD is an optimization algorithm that iteratively updates model parameters to minimize a loss function. CONCLUSION: The proposed work gives an accuracy of 99% in malware prediction and is free from overfitting and local minima.
Copyright © 2024 R. Vinoth Kumar et al., licensed to EAI. This is an open access article distributed under the terms of the CC BYNC-SA 4.0, which permits copying, redistributing, remixing, transformation, and building upon the material in any medium so long as the original work is properly cited.