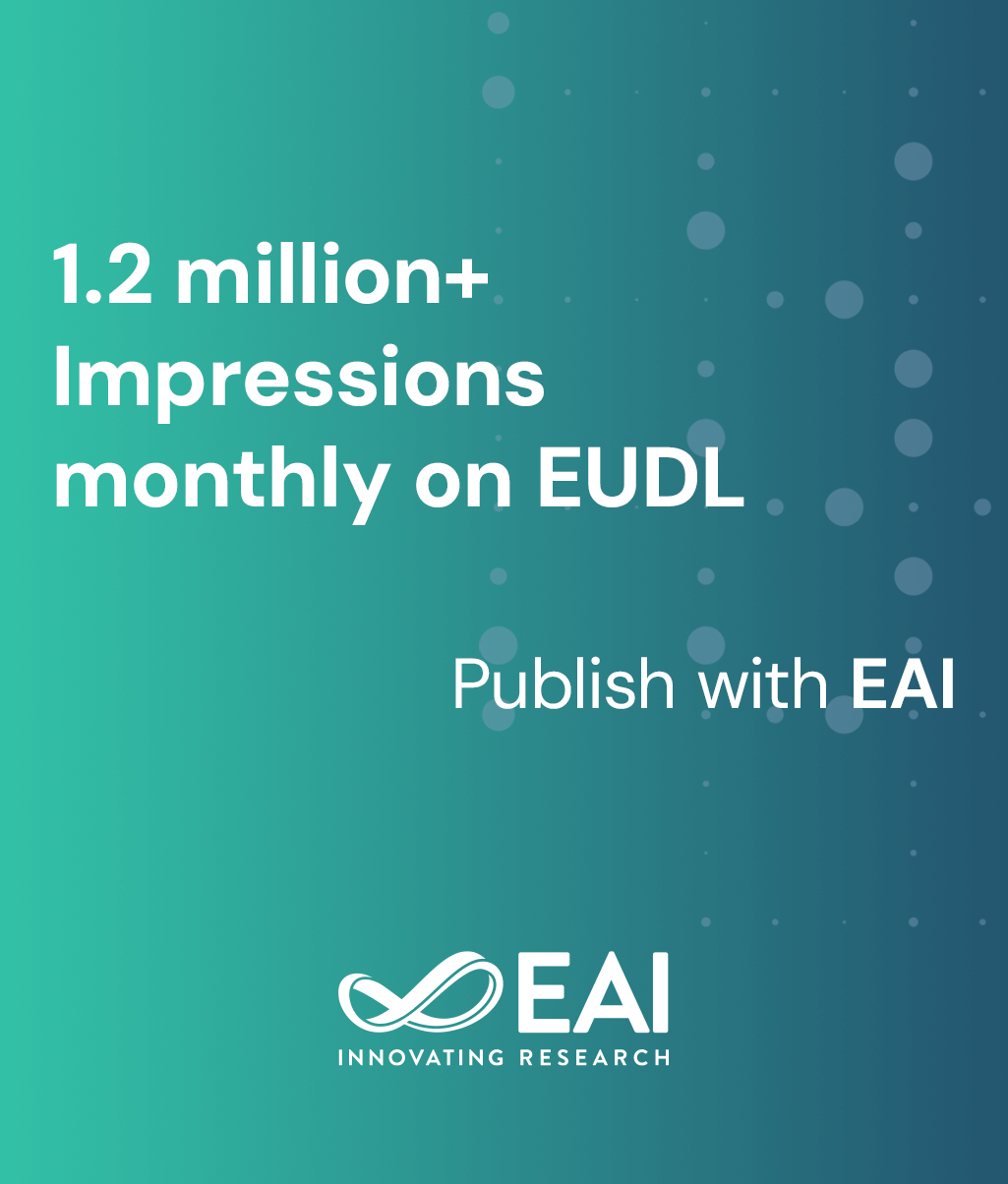
Research Article
Scalable Image Clustering to screen for self-produced CSAM
@ARTICLE{10.4108/eetiot.6631, author={Samantha Klier and Harald Baier}, title={Scalable Image Clustering to screen for self-produced CSAM}, journal={EAI Endorsed Transactions on Internet of Things}, volume={10}, number={1}, publisher={EAI}, journal_a={IOT}, year={2024}, month={7}, keywords={CSAM, clustering, metadata, EXIF, digital image forensics, data science, anti-forensic, source camera identification}, doi={10.4108/eetiot.6631} }
- Samantha Klier
Harald Baier
Year: 2024
Scalable Image Clustering to screen for self-produced CSAM
IOT
EAI
DOI: 10.4108/eetiot.6631
Abstract
The number of cases involving Child Sexual Abuse Material (CSAM) has increased dramatically in recent years, resulting in significant backlogs. To protect children in the suspect’s sphere of influence, immediate identification of self-produced CSAM among acquired CSAM is paramount. Currently, investigators often rely on an approach based on a simple metadata search. However, this approach faces scalability limitations for large cases and is ineffective against anti-forensic measures. Therefore, to address these problems, we bridge the gap between digital forensics and state-of-the-art data science clustering approaches. Our approach enables clustering of more than 130,000 images, which is eight times larger than previous achievements, using commodity hardware and within an hour with the ability to scale even further. In addition, we evaluate the effectiveness of our approach on seven publicly available forensic image databases, taking into account factors such as anti-forensic measures and social media post-processing. Our results show an excellent median clustering-precision (Homogeinity) of 0.92 on native images and a median clustering-recall (Completeness) of over 0.92 for each test set. Importantly, we provide full reproducibility using only publicly available algorithms, implementations, and image databases.
Copyright © 2024 S. Klier et al., licensed to EAI. This is an open access article distributed under the terms of the Creative Commons Attribution license (http://creativecommons.org/licenses/by/3.0/), which permits unlimited use, distribution and reproduction in any medium so long as the original work is properly cited.