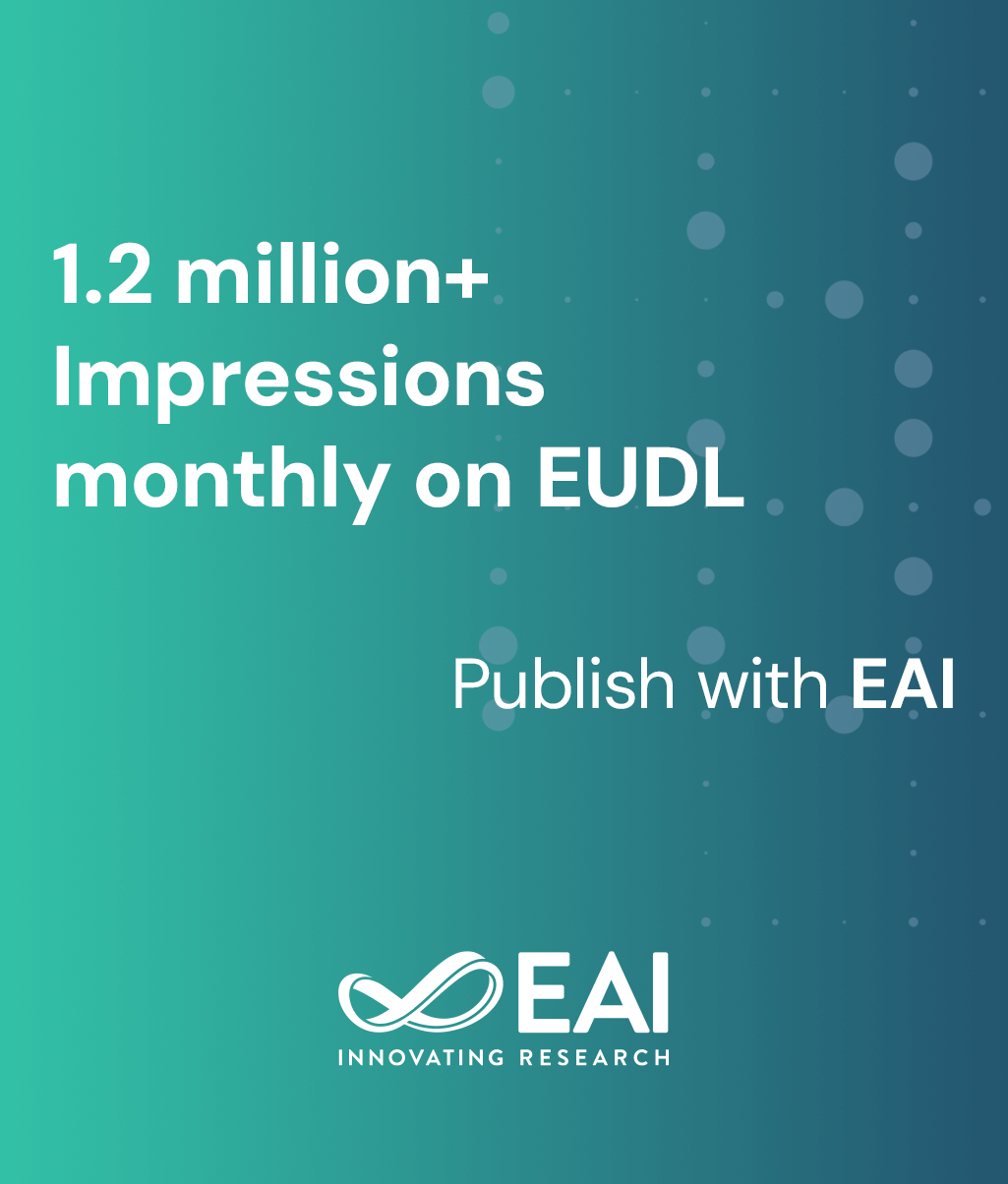
Research Article
Advancing Vehicle Security: Deep Learning based Solution for Defending CAN Networks in the Internet of Vehicles
@ARTICLE{10.4108/eetiot.6523, author={Kiran Aswal and Heman Pathak}, title={Advancing Vehicle Security: Deep Learning based Solution for Defending CAN Networks in the Internet of Vehicles}, journal={EAI Endorsed Transactions on Internet of Things}, volume={10}, number={1}, publisher={EAI}, journal_a={IOT}, year={2024}, month={12}, keywords={Autonomous vehicle, Internet of Vehicles (IoV), Controller Area Network (CAN), cybersecurity, deep learning, vehicular communication systems}, doi={10.4108/eetiot.6523} }
- Kiran Aswal
Heman Pathak
Year: 2024
Advancing Vehicle Security: Deep Learning based Solution for Defending CAN Networks in the Internet of Vehicles
IOT
EAI
DOI: 10.4108/eetiot.6523
Abstract
The Internet of Vehicle (IoV) is revolutionizing the automobile sector by allowing vehicles to interact with one another and with roadside infrastructure. The Controller Area Network (CAN) is a vital component of such smart vehicles, allowing communication between various Electronic Control Units (ECUs). However, the CAN protocol's intrinsic lack of security renders it open to a variety of cyber-attacks, posing substantial hazards to both safety and privacy. This research investigates the use of deep learning with multi-layer perceptron to improve the security of CAN networks inside the IoV framework. We discuss current threats to CAN networks, including spoofing, replay, and denial-of-service attacks, and how deep learning may be used to identify and mitigate these threats efficiently. We propose a unique deep learning-based defense mechanism for real-time threat detection. The suggested method is highly effective in identifying and mitigating potential risks, as evidenced by extensive testing on real-world CAN datasets. Based on our findings, the proposed solution has the potential to considerably enhance the security of CAN networks in the Internet of Vehicles, making car communication systems more secure and reliable.
Copyright © Aswal et al., licensed to EAI. This is an open access article distributed under the terms of the CC BY-NC-SA 4.0, which permits copying, redistributing, remixing, transformation, and building upon the material in any medium so long as the original work is properly cited.