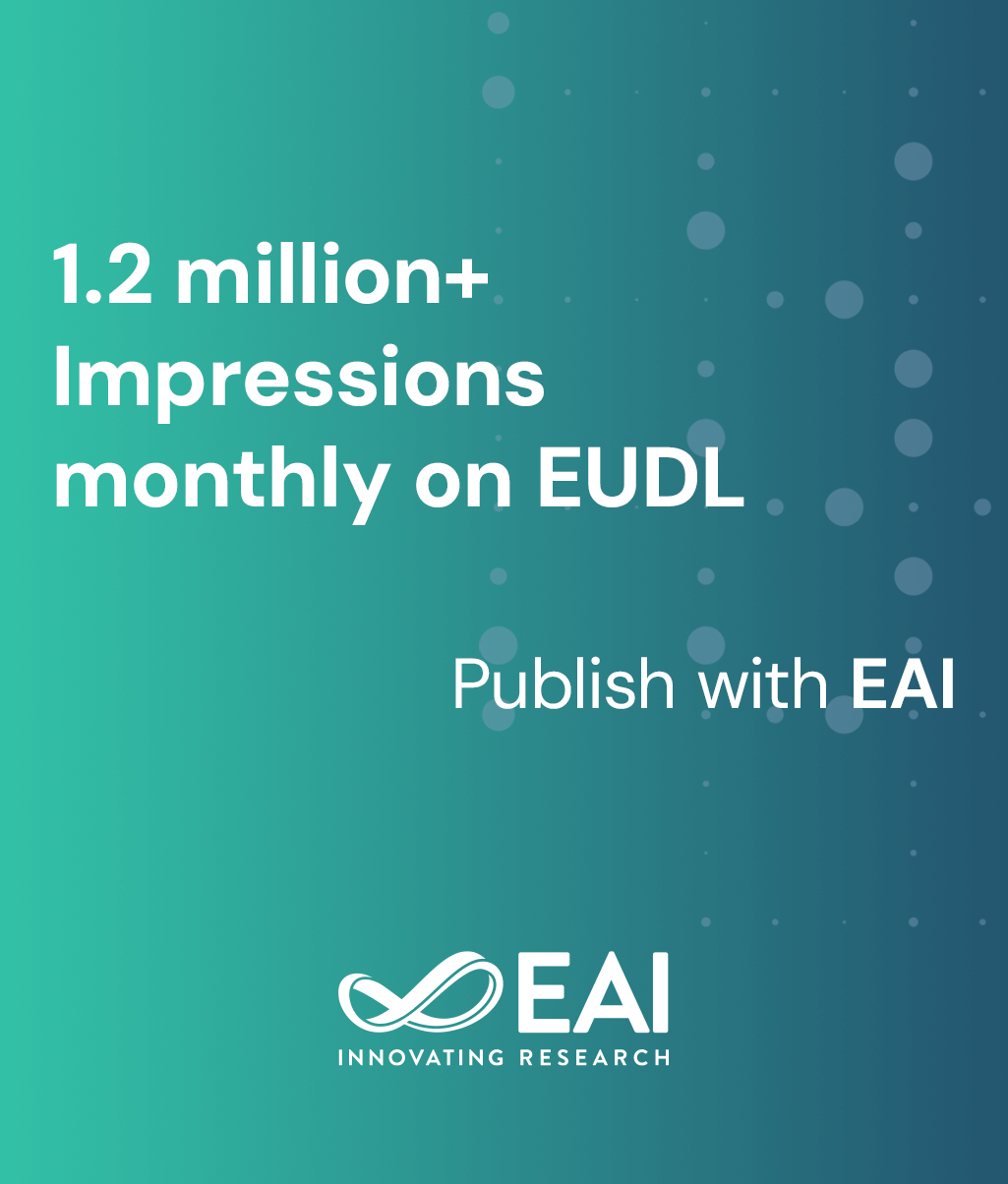
Research Article
A Self-Supervised GCN Model for Link Scheduling in Downlink NOMA Networks
@ARTICLE{10.4108/eetiot.6039, author={Caiya Zhang and Fang Fang and Congsong Zhang}, title={A Self-Supervised GCN Model for Link Scheduling in Downlink NOMA Networks}, journal={EAI Endorsed Transactions on Internet of Things}, volume={10}, number={1}, publisher={EAI}, journal_a={IOT}, year={2024}, month={12}, keywords={GNNs, graph convolutional neural networks, Non-orthogonal multiple access (NOMA), link scheduling.}, doi={10.4108/eetiot.6039} }
- Caiya Zhang
Fang Fang
Congsong Zhang
Year: 2024
A Self-Supervised GCN Model for Link Scheduling in Downlink NOMA Networks
IOT
EAI
DOI: 10.4108/eetiot.6039
Abstract
INTRODUCTION: Downlink Non-Orthogonal Multiple Access (NOMA) networks pose challenges in optimizing power allocation efficiency due to their complex design. OBJECTIVES: This paper aims to propose a novel scheme utilizing Graph Neural Networks to address the optimization challenges in downlink NOMA networks. METHODS: We transform the optimization problem into an optimal link scheduling problem by modeling the network as a bipartite graph. Leveraging Graph Convolutional Networks, we employ self-supervised learning to learn the optimal link scheduling strategy. RESULTS: Simulation results showcase a significant enhancement in power allocation efficiency in downlink NOMA networks, evidenced by notable improvements in both average accuracy and generalization ability. CONCLUSION: Our proposed scheme demonstrates promising potential in substantially augmenting power allocation efficiency within downlink NOMA networks, offering a promising avenue for further research and application in wireless communications.
Copyright © 2024 C. Zhang et al., licensed to EAI. This is an open access article distributed under the terms of the CC BY-NC-SA 4.0, which permits copying, redistributing, remixing, transformation, and building upon the material in any medium so long as the original work is properly cited.