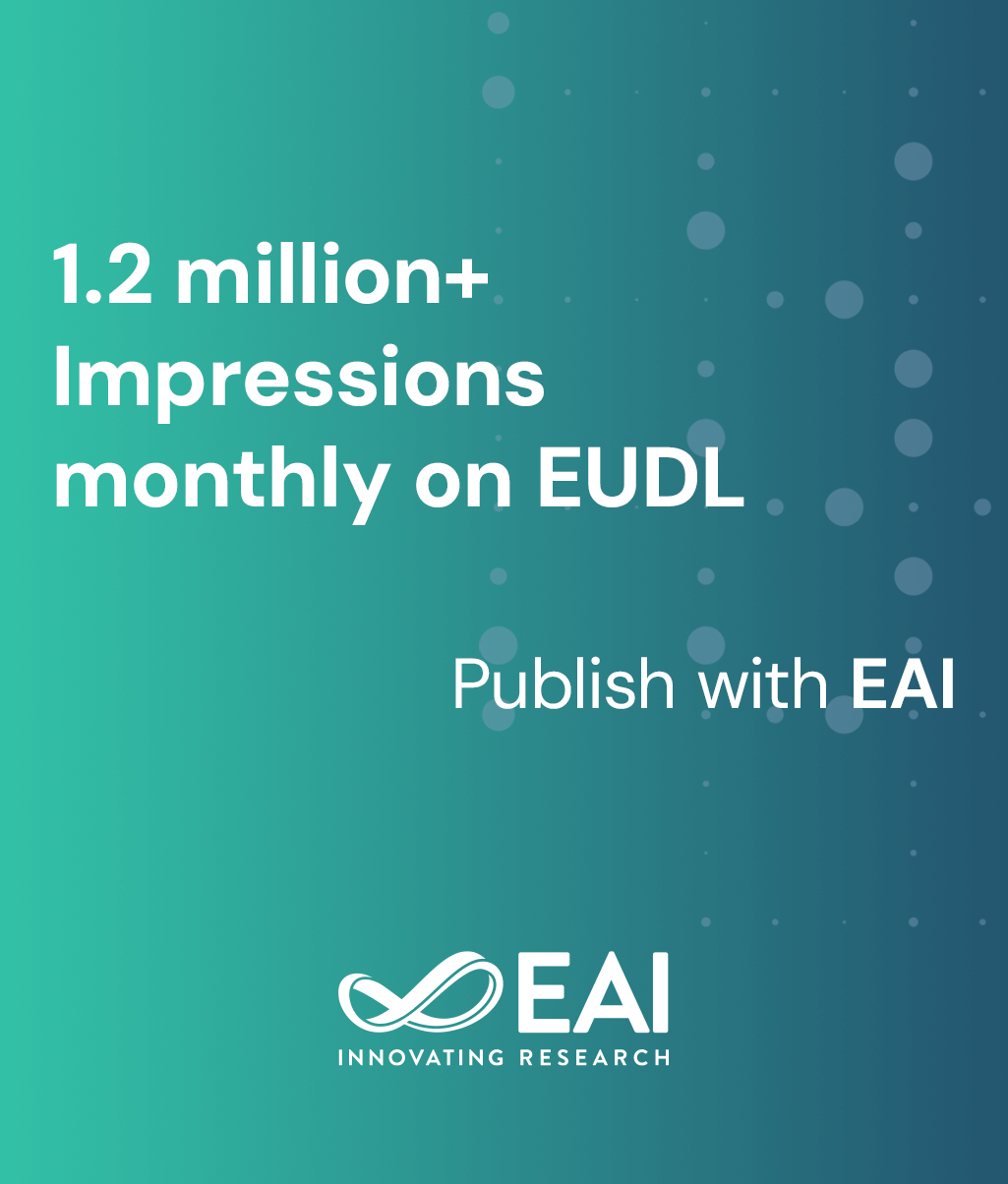
Research Article
Assessment of CatBoost for Diabetes Prevention in Comparison to XGBoost: AI model capable of predicting the onset of diabetes
@ARTICLE{10.4108/eetiot.5880, author={Jagadeesh Selvaraj and G. Gifta Jerith and Karthikeyan R and Senthil K}, title={Assessment of CatBoost for Diabetes Prevention in Comparison to XGBoost: AI model capable of predicting the onset of diabetes}, journal={EAI Endorsed Transactions on Internet of Things}, volume={11}, number={1}, publisher={EAI}, journal_a={IOT}, year={2025}, month={4}, keywords={}, doi={10.4108/eetiot.5880} }
- Jagadeesh Selvaraj
G. Gifta Jerith
Karthikeyan R
Senthil K
Year: 2025
Assessment of CatBoost for Diabetes Prevention in Comparison to XGBoost: AI model capable of predicting the onset of diabetes
IOT
EAI
DOI: 10.4108/eetiot.5880
Abstract
The metabolic disease known as diabetes is defined by consistently elevated blood sugar levels. An increase in hunger, thirst, and urine production are symptoms of high blood sugar. Untreated diabetes may lead to a variety of complications. Acute complications of diabetes include hyperosmolarity, hyperglycemia, diabetic ketoacidosis, and perhaps death. The most devastating long-term effects are cardiovascular disease, cerebrovascular accident, chronic kidney disease, foot ulcers, and vision loss. The World Diabetes Organization estimates that 463 million people were diagnosed with diabetes in 2019. This population will increase by 578 million by 2030 and by 700 million by 2045, if forecasts pan out. The ability to quickly and accurately diagnose sickness is one of its current medical uses. Therefore, we might potentially reduce death rates via the use of machine learning by creating an AI model that can anticipate when diabetes will start. We will compare the CatBoost and XGBoost algorithms to find the one that is most suited for this purpose. Finally, using a number of health markers from the dataset, the study contrasted XGBoost and CatBoost, two models that may predict diabetes. We train and build our recommended system using Python on a real-world dataset taken from Kaggle. We evaluate our algorithms using precision, recall, F1score, and accuracy metrics, among other performance evaluation parameters. While XGBoost achieved an F1 Score of 91.75, an accuracy rate of 93.33%, a precision of 90.38%, and a recall of 90.63%. The accuracy, precision, recall, and F1 score for CatBoost are 96.09%, 93.38%, 91.38% and 92.13%, respectively. It's the most effective ensemble method, according to CatBoost.
Copyright © 2025 J. Selvaraj et al., licensed to EAI. This is an open access article distributed under the terms of the CC BY-NC-SA 4.0, which permits copying, redistributing, remixing, transformation, and building upon the material in any medium so long as the original work is properly cited.