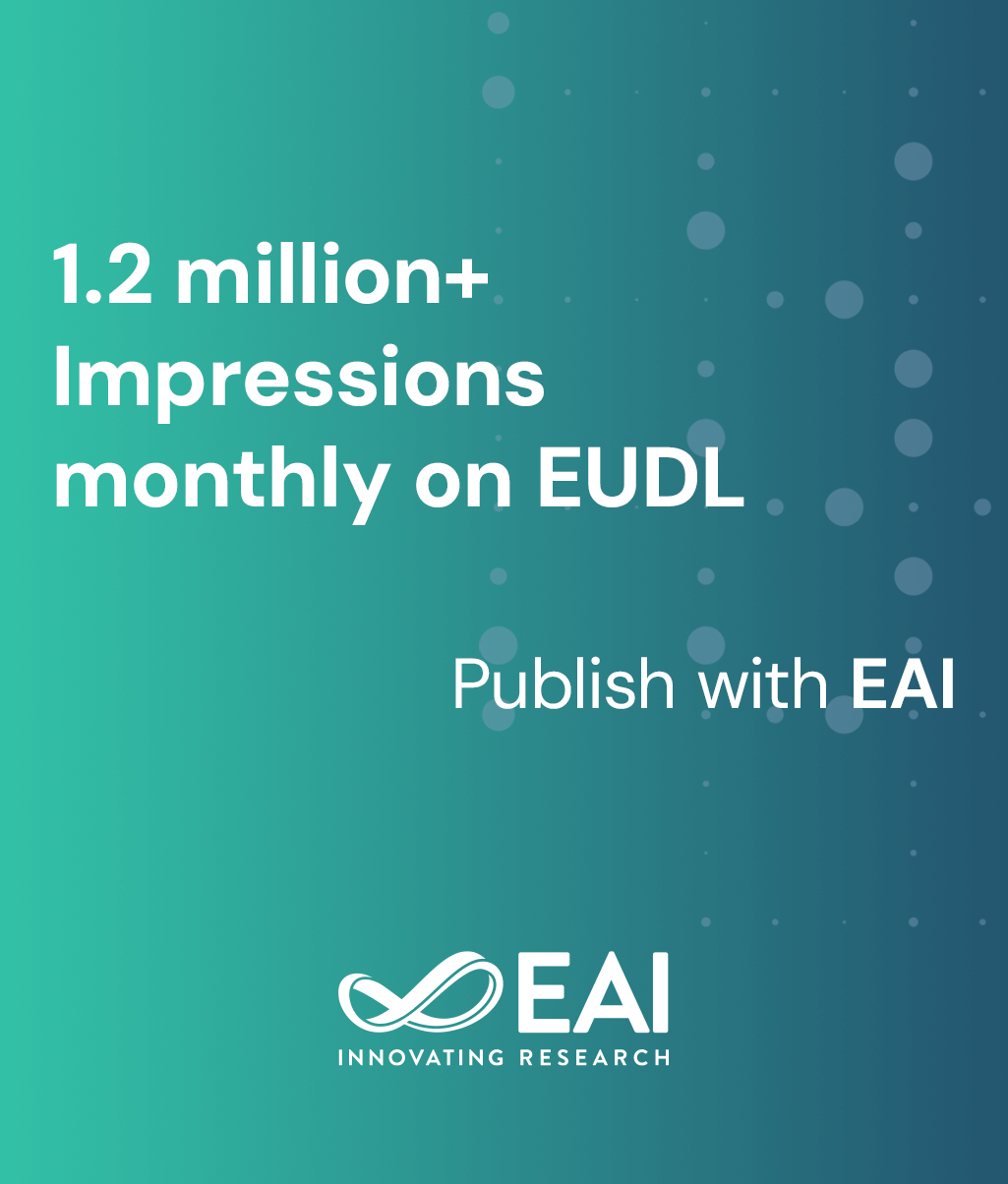
Editorial
Early Detection of Cardiovascular Disease with Different Machine Learning Approaches
@ARTICLE{10.4108/eetiot.5389, author={Eyashita Singh and Vartika Singh and Aryan Rai and Ivan Christopher and Raj Mishra and K S Arikumar}, title={Early Detection of Cardiovascular Disease with Different Machine Learning Approaches}, journal={EAI Endorsed Transactions on Internet of Things}, volume={10}, number={1}, publisher={EAI}, journal_a={IOT}, year={2024}, month={3}, keywords={Cardiovascular disease, Early Detection, Machine Learning, Deep Learning, Healthcare}, doi={10.4108/eetiot.5389} }
- Eyashita Singh
Vartika Singh
Aryan Rai
Ivan Christopher
Raj Mishra
K S Arikumar
Year: 2024
Early Detection of Cardiovascular Disease with Different Machine Learning Approaches
IOT
EAI
DOI: 10.4108/eetiot.5389
Abstract
With the increase in mortality rate around the world in recent years, cardiovascular diseases (CVD) have swiftly become a leading cause of morbidity, and therefore there arises a need for early diagnosis of disease to ensure effective treatment. With machine learning emerging as a promising tool for the detection, this study aims to propose and compare various algorithms for the detection of CVD via several evaluation metrics including accuracy, precision, F1 score, and recall. ML has the ability and potential to improve CVD prediction, detection, and treatment by analysis of patient information and identification of patterns that may be difficult for humans to interpret and detect. Several state-of-the-art ML and DL models such as Decision Tree, XGBoost, KNN, and ANN were employed. The results of these models reflect the potential of Machine Learning in the detection of CVD detection and subsequently highlight the need for their integration into clinical practice along with the suggestion of the development of robust and accurate models to improve the predictions. This integration, however, significantly helps in the reduction of the burden of CVD on healthcare systems.
Copyright © 2024 E. Singh et al., licensed to EAI. This is an open access article distributed under the terms of the CC BY-NC-SA 4.0, which permits copying, redistributing, remixing, transformation, and building upon the material in any medium so long as the original work is properly cited.