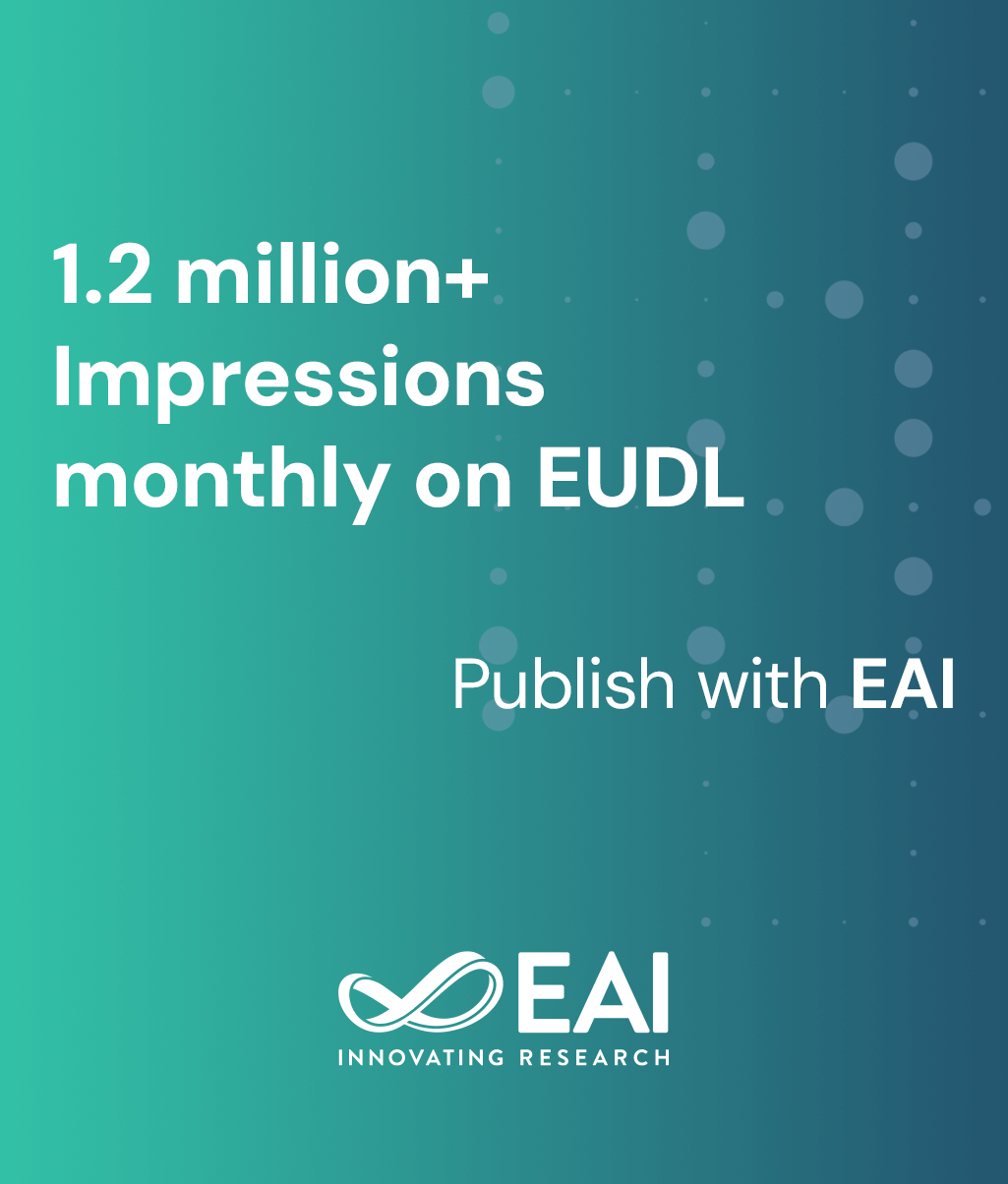
Editorial
Machine Learning Based Stroke Predictor Application
@ARTICLE{10.4108/eetiot.5384, author={R Kishore Kanna and Ch. Venkata Rami Reddy and Bhawani Sankar Panigrahi and Naliniprava Behera and Sarita Mohanty}, title={Machine Learning Based Stroke Predictor Application}, journal={EAI Endorsed Transactions on Internet of Things}, volume={10}, number={1}, publisher={EAI}, journal_a={IOT}, year={2024}, month={3}, keywords={Stroke, SVM, Healthcare, Machine Learning, ML}, doi={10.4108/eetiot.5384} }
- R Kishore Kanna
Ch. Venkata Rami Reddy
Bhawani Sankar Panigrahi
Naliniprava Behera
Sarita Mohanty
Year: 2024
Machine Learning Based Stroke Predictor Application
IOT
EAI
DOI: 10.4108/eetiot.5384
Abstract
When blood flow to the brain stops or slows down, brain cells die because they don't get enough oxygen and nutrients. This condition is known as an ischemic stroke. It is now the biggest cause of death in the whole planet. Examining the afflicted people has shown a number of risk variables that are thought to be connected to the stroke's origin. Numerous studies have been conducted to predict the illnesses associated with stroke using these risk variables. The prompt identification of various warning symptoms associated with stroke has the potential to mitigate the severity of the stroke. The utilization of machine learning techniques yields prompt and precise predictive outcomes. Although its uses in healthcare are expanding, certain research domains have a stronger need for more study. We think that machine learning algorithms may aid in a deeper comprehension of illnesses and make an excellent healthcare partner. The textual dataset of numerous patients, which includes many medical variables, is gathered for this study. The missing values in the dataset are located and dealt with during processing. The dataset is used to train machine learning algorithms including Random Forest, Decision Tree classifier, and SVM. The method that delivers the greatest accuracy for our dataset is then selected once the accuracy of the algorithms has been determined. This aids patients in determining the likelihood of a brain stroke and ensuring they get the right medical attention.
Copyright © 2024 R. Kishore Kanna et al., licensed to EAI. This is an open access article distributed under the terms of the CC BY-NCSA 4.0, which permits copying, redistributing, remixing, transformation, and building upon the material in any medium so long as the original work is properly cited.