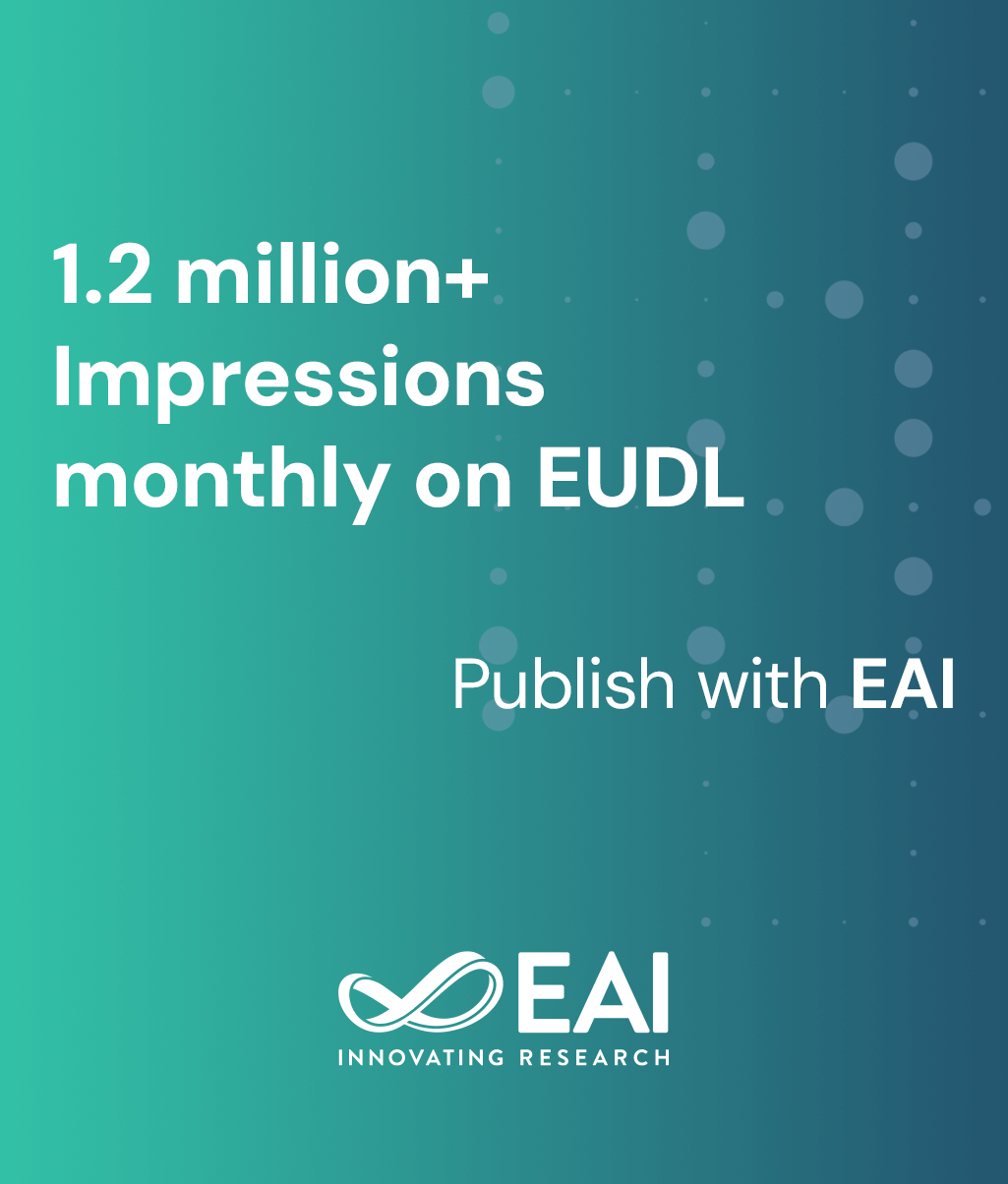
Editorial
Predicting Academic Success: A Comparative Study of Machine Learning and Clustering-Based Subject Recommendation Models
@ARTICLE{10.4108/eetiot.5378, author={Kinjal and Sagar Mousam Parida and Jayesh Suthar and Sagar Dhanraj Pande}, title={Predicting Academic Success: A Comparative Study of Machine Learning and Clustering-Based Subject Recommendation Models}, journal={EAI Endorsed Transactions on Internet of Things}, volume={10}, number={1}, publisher={EAI}, journal_a={IOT}, year={2024}, month={3}, keywords={K-means Clustering, Neural Networks, Academic Performance, Prediction, Recommend}, doi={10.4108/eetiot.5378} }
- Kinjal
Sagar Mousam Parida
Jayesh Suthar
Sagar Dhanraj Pande
Year: 2024
Predicting Academic Success: A Comparative Study of Machine Learning and Clustering-Based Subject Recommendation Models
IOT
EAI
DOI: 10.4108/eetiot.5378
Abstract
The study of students' academic performance is a significant endeavor for higher education schools and universities since it is essential to the design and management of instructional strategies. The efficacy of the current educational system must be monitored by evaluating student achievement. For this research, we used multiple Machine Learning algorithms and Neural Networks to analyze the learning quality. This study investigates the real results of university examinations for B.Tech (Bachelor in Technology) students, a four-year undergraduate programme in Computer Science and Technology. The K-means clustering approach is used to recommend courses, highlighting those that would challenge students and those that will improve their GPA. The Linear Regression method is used to make a prediction of a student’s rank among their batchmates. Academic planners might base operational choices and future planning on the findings of this study.
Copyright © 2024 Kinjal et al., licensed to EAI. This is an open access article distributed under the terms of the CC BY-NC-SA 4.0, which permits copying, redistributing, remixing, transformation, and building upon the material in any medium so long as the original work is properly cited.