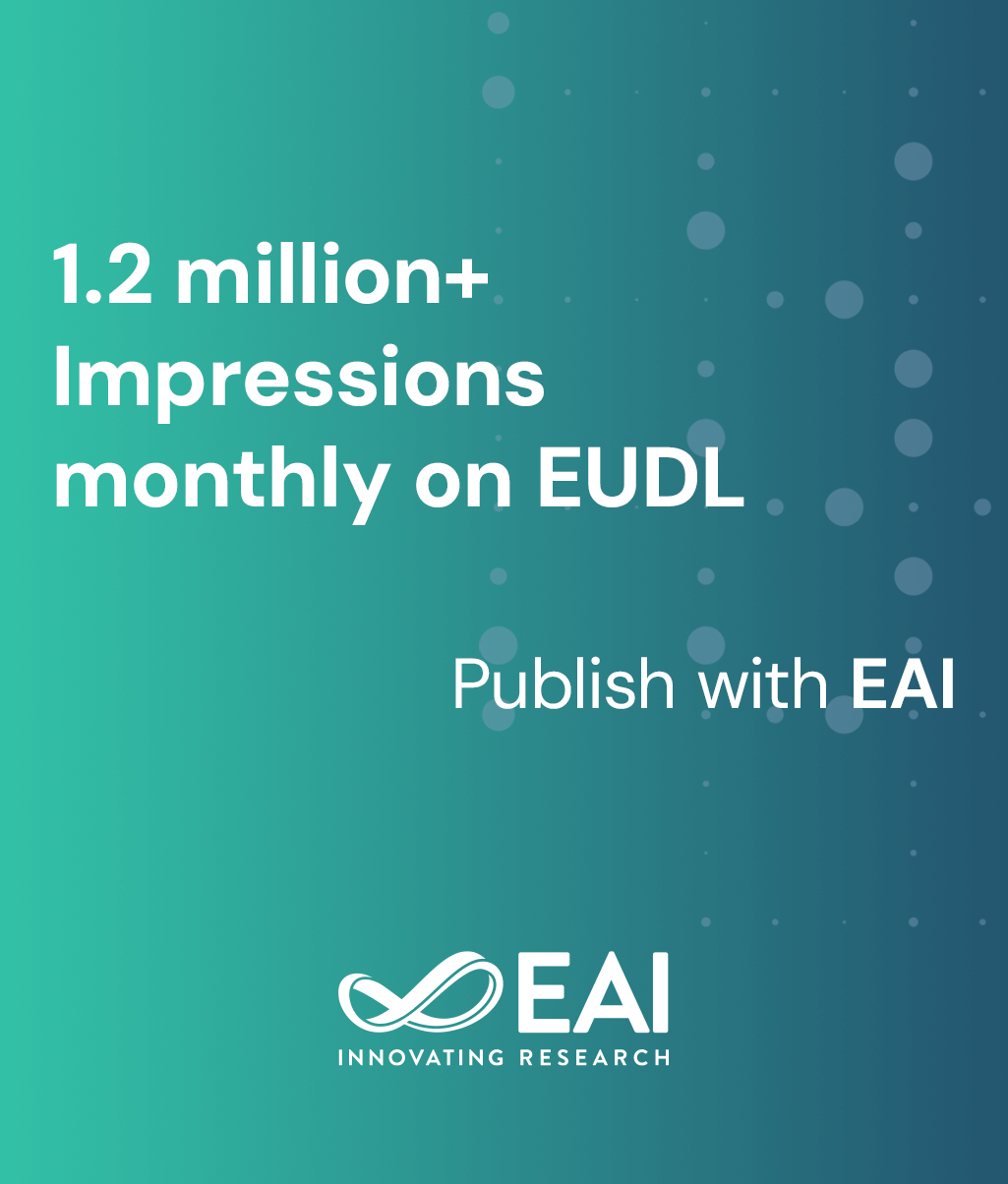
Research Article
Improving Student Grade Prediction Using Hybrid Stacking Machine Learning Model
@ARTICLE{10.4108/eetiot.5369, author={Saloni Reddy and Sagar Dhanraj Pande}, title={Improving Student Grade Prediction Using Hybrid Stacking Machine Learning Model}, journal={EAI Endorsed Transactions on Internet of Things}, volume={10}, number={1}, publisher={EAI}, journal_a={IOT}, year={2024}, month={3}, keywords={Hybrid Model, Grade Prediction, Stack Model}, doi={10.4108/eetiot.5369} }
- Saloni Reddy
Sagar Dhanraj Pande
Year: 2024
Improving Student Grade Prediction Using Hybrid Stacking Machine Learning Model
IOT
EAI
DOI: 10.4108/eetiot.5369
Abstract
With increasing technical procedures, academic institutions are adapting to a data-driven decision-making approach of which grade prediction is an integral part. The purpose of this study is to propose a hybrid model based on a stacking approach and compare its accuracy with those of the individual base models. The model hybridizes K-nearest neighbours, Random forests, XGBoost and multi-layer perceptron networks to improve the accuracy of grade prediction by enabling a combination of strengths of different algorithms for the creation of a more robust and accurate model. The proposed model achieved an average overall accuracy of around 90.9% for 10 epochs, which is significantly higher than that achieved by any of the individual algorithms of the stack. The results demonstrate the improvement of prediction results but using a stacking approach. This study has significant implications for academic institutions which can help them make informed grade predictions for the improvement of student outcomes.
Copyright © 2024 S. Reddy et al., licensed to EAI. This is an open access article distributed under the terms of the CC BY-NC-SA 4.0, which permits copying, redistributing, remixing, transformation, and building upon the material in any medium so long as the original work is properly cited.