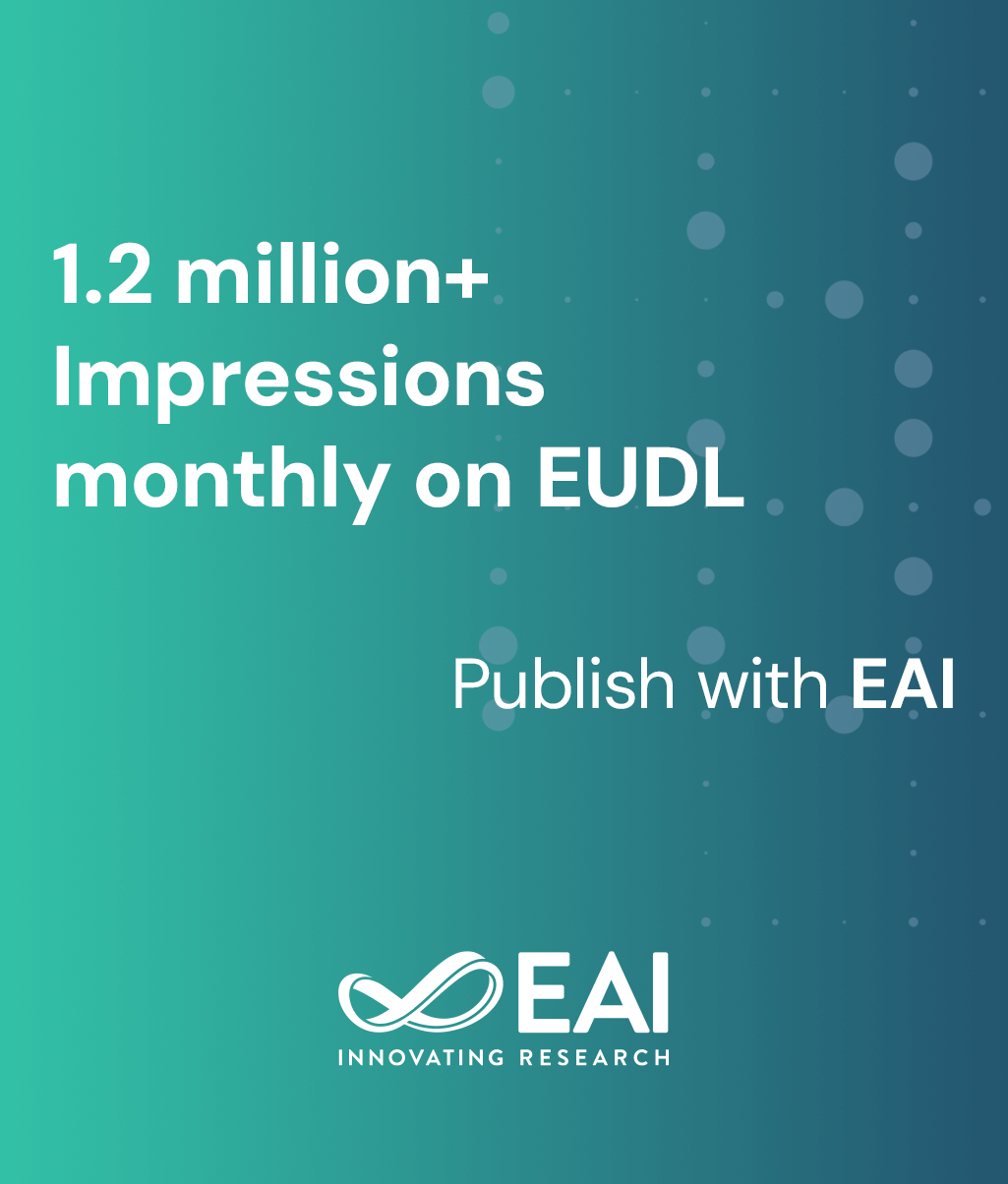
Editorial
Enhancing Diabetes Prediction with Data Preprocessing and various Machine Learning Algorithms
@ARTICLE{10.4108/eetiot.5348, author={Gudluri Saranya and Sagar Dhanraj Pande}, title={Enhancing Diabetes Prediction with Data Preprocessing and various Machine Learning Algorithms}, journal={EAI Endorsed Transactions on Internet of Things}, volume={10}, number={1}, publisher={EAI}, journal_a={IOT}, year={2024}, month={3}, keywords={Accuracy, Diabetes, Machine Learning, Naive Bayes, Random Forest}, doi={10.4108/eetiot.5348} }
- Gudluri Saranya
Sagar Dhanraj Pande
Year: 2024
Enhancing Diabetes Prediction with Data Preprocessing and various Machine Learning Algorithms
IOT
EAI
DOI: 10.4108/eetiot.5348
Abstract
Diabetes mellitus, usually called diabetes, is a serious public health issue that is spreading like an epidemic around the world. It is a condition that results in elevated glucose levels in the blood. India is often referred to as the 'Diabetes Capital of the World', due to the country's 17% share of the global diabetes population. It is estimated that 77 million Indians over the age of 18 have diabetes (i.e., everyone in eleven) and there are also an estimated 25 million pre-diabetics. One of the solutions to control diabetes growth is to detect it at an early stage which can lead to improved treatment. So, in this project, we are using a few machine learning algorithms like SVM, Decision Tree Classifier, Random Forest, KNN, Linear regression, Logistic regression, Naive Bayes to effectively predict the diabetes. Pima Indians Diabetes Database has been used in this project. According to the experimental findings, Random Forest produced an accuracy of 91.10% which is higher among the different algorithms used.
Copyright © 2024 Saranya et al., licensed to EAI. This is an open access article distributed under the terms of the CC BY-NC-SA 4.0, which permits copying, redistributing, remixing, transformation, and building upon the material in any medium so long as the original work is properly cited.