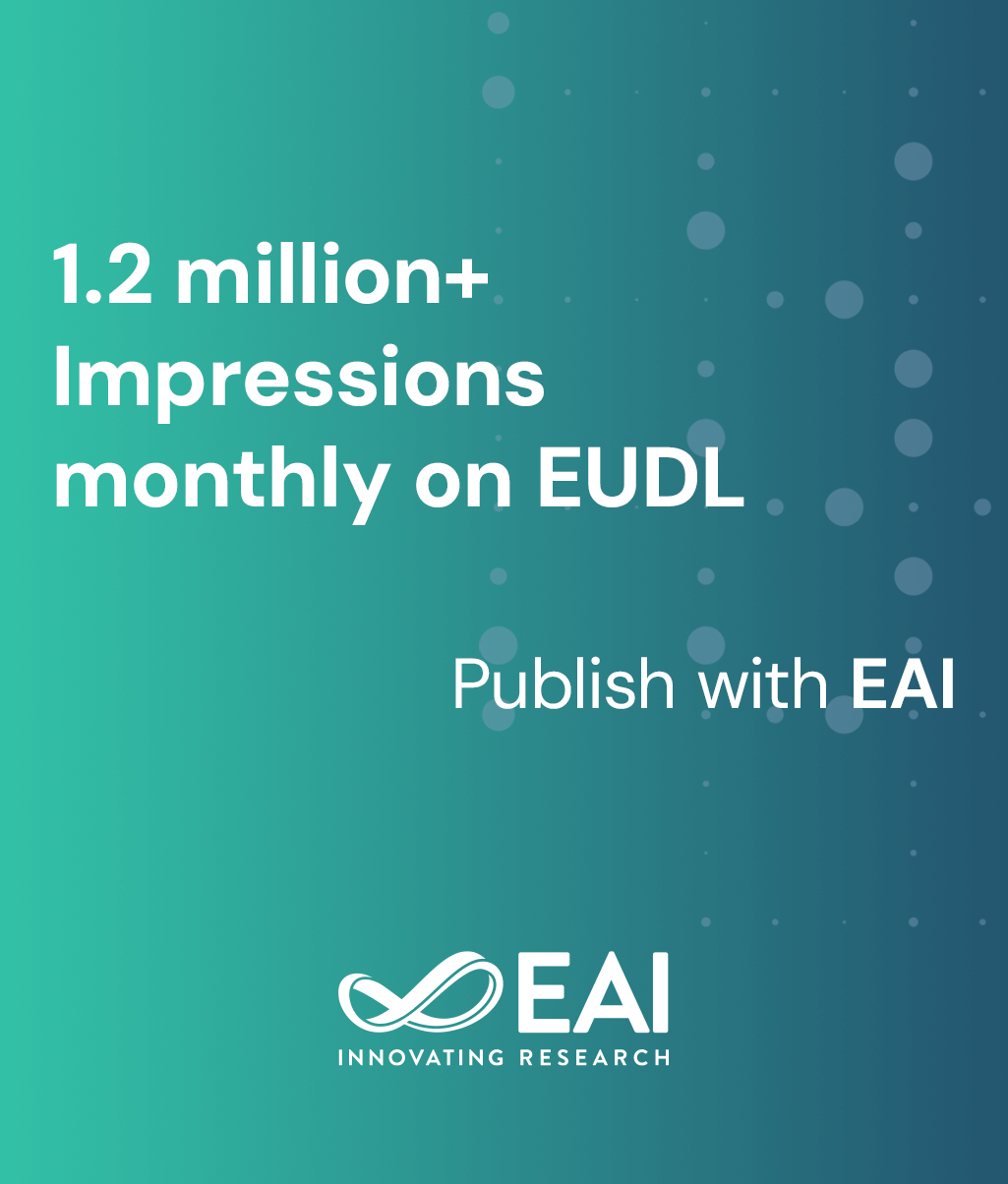
Research Article
Applied Deep learning approaches on canker effected leaves to enhance the detection of the disease using Image Embedding and Machine learning Techniques
@ARTICLE{10.4108/eetiot.5346, author={K Badri Narayanan and Devatha Krishna Sai and Korrapati Akhil Chowdary and Srinivasa Reddy K}, title={Applied Deep learning approaches on canker effected leaves to enhance the detection of the disease using Image Embedding and Machine learning Techniques}, journal={EAI Endorsed Transactions on Internet of Things}, volume={10}, number={1}, publisher={EAI}, journal_a={IOT}, year={2024}, month={3}, keywords={Canker, Convolutional Neural Network, Machine Learning, Image Embedding, InceptionV3}, doi={10.4108/eetiot.5346} }
- K Badri Narayanan
Devatha Krishna Sai
Korrapati Akhil Chowdary
Srinivasa Reddy K
Year: 2024
Applied Deep learning approaches on canker effected leaves to enhance the detection of the disease using Image Embedding and Machine learning Techniques
IOT
EAI
DOI: 10.4108/eetiot.5346
Abstract
Canker, a disease that causes considerable financial losses in the agricultural business, is a small deep lesion that is visible on the leaves of many plants, especially citrus/apple trees. Canker detection is critical for limiting its spread and minimizing harm. To address this issue, we describe a computer vision-based technique that detects Canker in citrus leaves using image embedding and machine learning (ML) algorithms. The major steps in our proposed model include image embedding, and machine learning model training and testing. We started with preprocessing and then used image embedding techniques like Inception V3 and VGG 16 to turn the ROIs into feature vectors that retained the relevant information associated with Canker leaf disease, using the feature vectors acquired from the embedding stage, we then train and evaluate various ML models such as support vector machines (SVM), Gradient Boosting, neural network, and K Nearest Neighbor. Our experimental results utilizing a citrus leaf picture dataset show that the proposed strategy works. With Inception V3 as the image embedder and neural network machine learning model we have obtained an accuracy of 95.6% which suggests that our approach is effective in canker identification. Our method skips traditional image processing techniques that rely on by hand features and produces results equivalent to cutting-edge methods that use deep learning models. Finally, our proposed method provides a dependable and efficient method for detecting Canker in leaves. Farmers and agricultural specialists can benefit greatly from early illness diagnosis and quick intervention to avoid disease spread as adoption of such methods can significantly reduce the losses incurred by farmers and improve the quality of agricultural produce.
Copyright © 2024 K Badri Narayman et al., licensed to EAI. This is an open access article distributed under the terms of the CC BYNC-SA 4.0, which permits copying, redistributing, remixing, transformation, and building upon the material in any medium so long as the original work is properly cited.