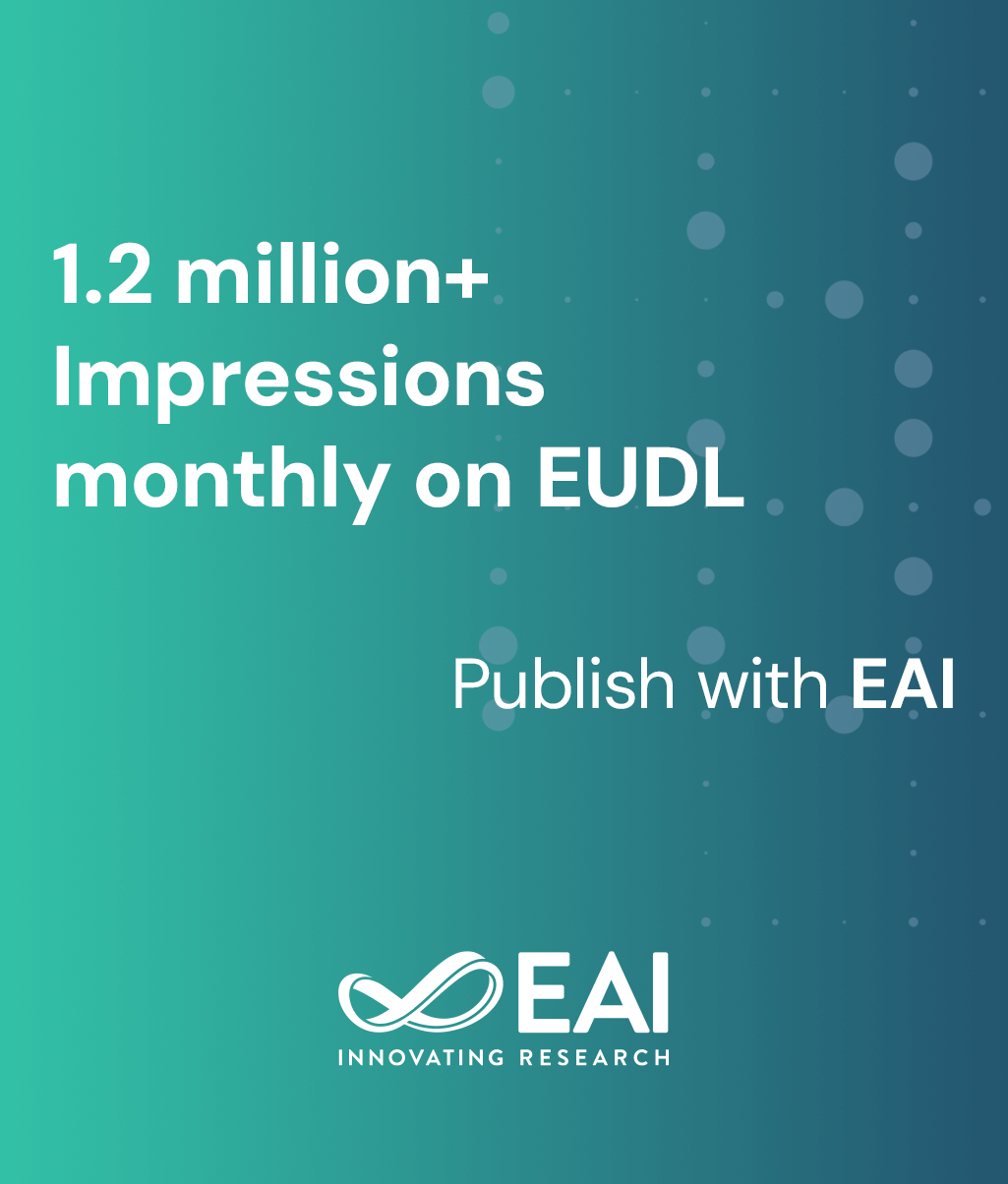
Editorial
Analyse and Predict the Detection of the Cyber - Attack Process by Using a Machine-Learning Approach
@ARTICLE{10.4108/eetiot.5345, author={Charanjeet Singh and Ravinjit Singh and Shivaputra and Mohit Tiwari and Bramah Hazela}, title={Analyse and Predict the Detection of the Cyber - Attack Process by Using a Machine-Learning Approach}, journal={EAI Endorsed Transactions on Internet of Things}, volume={10}, number={1}, publisher={EAI}, journal_a={IOT}, year={2024}, month={3}, keywords={Machine Learning, Cyber-attacks, Cybercrime, SVM}, doi={10.4108/eetiot.5345} }
- Charanjeet Singh
Ravinjit Singh
Shivaputra
Mohit Tiwari
Bramah Hazela
Year: 2024
Analyse and Predict the Detection of the Cyber - Attack Process by Using a Machine-Learning Approach
IOT
EAI
DOI: 10.4108/eetiot.5345
Abstract
Crimes committed online rank among the most critical global concerns. Daily, they cause country and citizen economies to suffer massive financial losses. With the proliferation of cyber-attacks, cybercrime has also been on the rise. To effectively combat cybercrime, it is essential to identify its perpetrators and understand their methods. Identifying and preventing cyber-attacks are difficult tasks. To combat these concerns, however, new research has produced safety models and forecast tools grounded on artificial intelligence. Numerous methods for predicting criminal behaviour are available in the literature. While they may not be perfect, they may help in cybercrime and cyber-attack tactic prediction. To find out whether an attack happened and, if so, who was responsible, one way to look at this problem is by using real-world data. There is data about the crime, the perpetrator's demographics, the amount of property damaged, and the entry points for the assault. Potentially, by submitting applications to forensics teams, victims of cyber-attacks may get information. This study uses ML methods to analyse cyber-crime consuming two patterns and to forecast how the specified characteristics will furnish to the detection of the cyber-attack methodology and perpetrator. Based on the comparison of eight distinct machine-learning methods, one can say that their accuracy was quite comparable. The Support Vector Machine (SVM) Linear outperformed all other cyber-attack tactics in terms of accuracy. The initial model gave us a decent notion of the assaults that the victims would face. The most successful technique for detecting malevolent actors was logistic regression, according to the success rate. To anticipate who the perpetrator and victim would be, the second model compared their traits. A person’s chances of being a victim of a cyber-attack decrease as their income and level of education rise. The proposed idea is expected to be used by departments dealing with cybercrime. Cyber-attack identification will also be made easier, and the fight against them will be more efficient.
Copyright © 2024 C. Singh et al., licensed to EAI. This is an open access article distributed under the terms of the CC BY-NC-SA 4.0, which permits copying, redistributing, remixing, transformation, and building upon the material in any medium so long as the original work is properly cited.