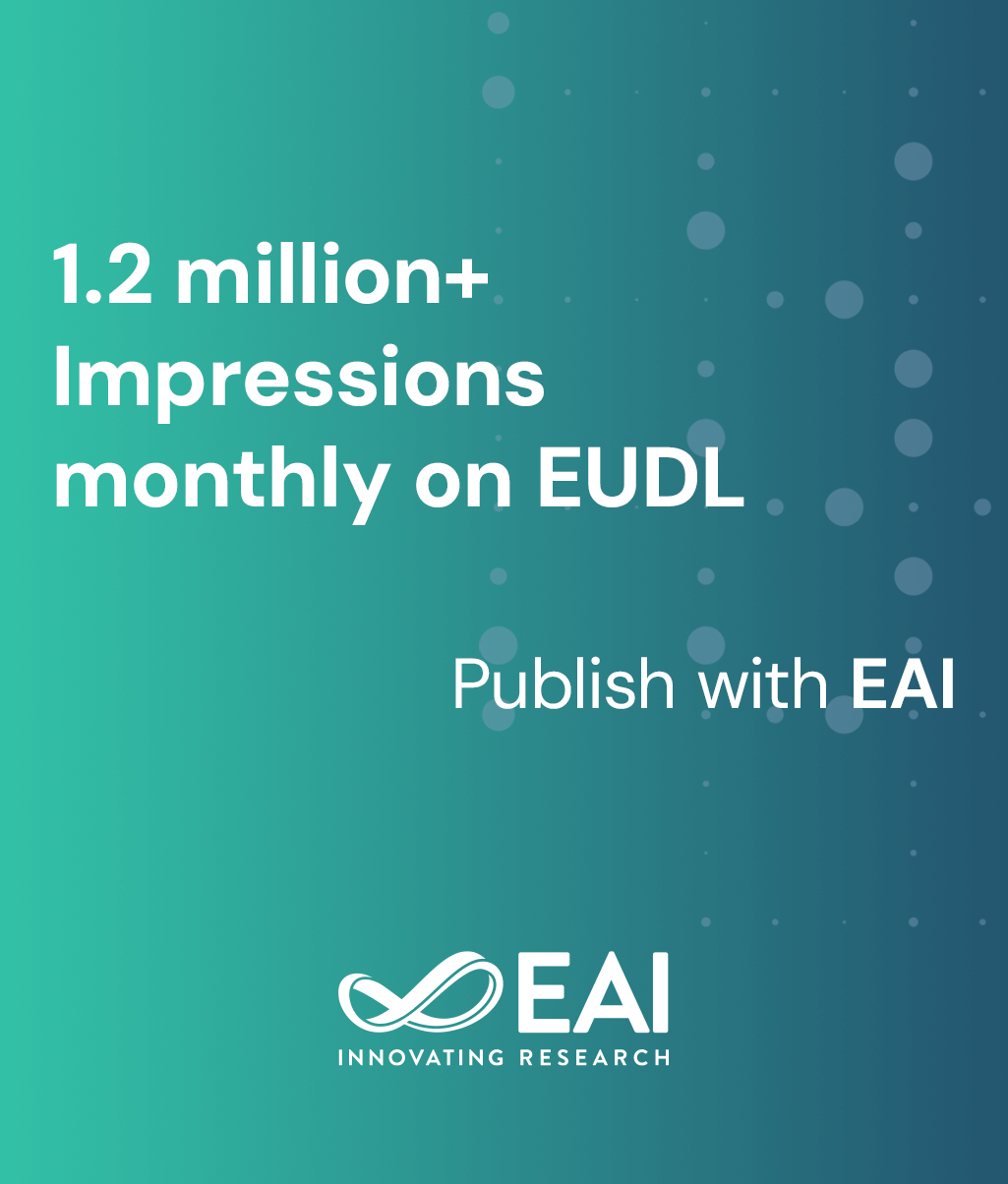
Editorial
An Efficient Crop Yield Prediction System Using Machine Learning
@ARTICLE{10.4108/eetiot.5333, author={Debabrata Swain and Sachin Lakum and Samrat Patel and Pramoda Patro and Jatin }, title={An Efficient Crop Yield Prediction System Using Machine Learning}, journal={EAI Endorsed Transactions on Internet of Things}, volume={10}, number={1}, publisher={EAI}, journal_a={IOT}, year={2024}, month={3}, keywords={Farming, Regression, Crop Prediction, Mean Absolute Error, MAE, Root mean square Error, RMSE, R2 Score}, doi={10.4108/eetiot.5333} }
- Debabrata Swain
Sachin Lakum
Samrat Patel
Pramoda Patro
Jatin
Year: 2024
An Efficient Crop Yield Prediction System Using Machine Learning
IOT
EAI
DOI: 10.4108/eetiot.5333
Abstract
Farming is considered the biggest factor in strengthening the economy of any country. It also has significant effects on GDP growth. However, due to a lack of information and consultation, farmers suffer from significant crop losses every year. Typically, farmers consult agricultural officers for detecting crop diseases. However, the accuracy of predictions made by agricultural officers based on their experience is not always reliable. If the exact issues are not identified at right time then it results in a heavy crop loss. To address this issue, Computational Intelligence, also known as Machine Learning, can be applied based on historical data. In this study, an intelligent crop yield prediction algorithm is developed using various types of regression-based algorithms. The Crop Yield Prediction Dataset from the Kaggle repository is used for model training and evaluation. Among all different regression methods Random Forest has shown the better performance in terms of R2 score and other errors.
Copyright © 2024 D. Swain et al., licensed to EAI. This is an open access article distributed under the terms of the CC BY-NC-SA 4.0, which permits copying, redistributing, remixing, transformation, and building upon the material in any medium so long as the original work is properly cited.