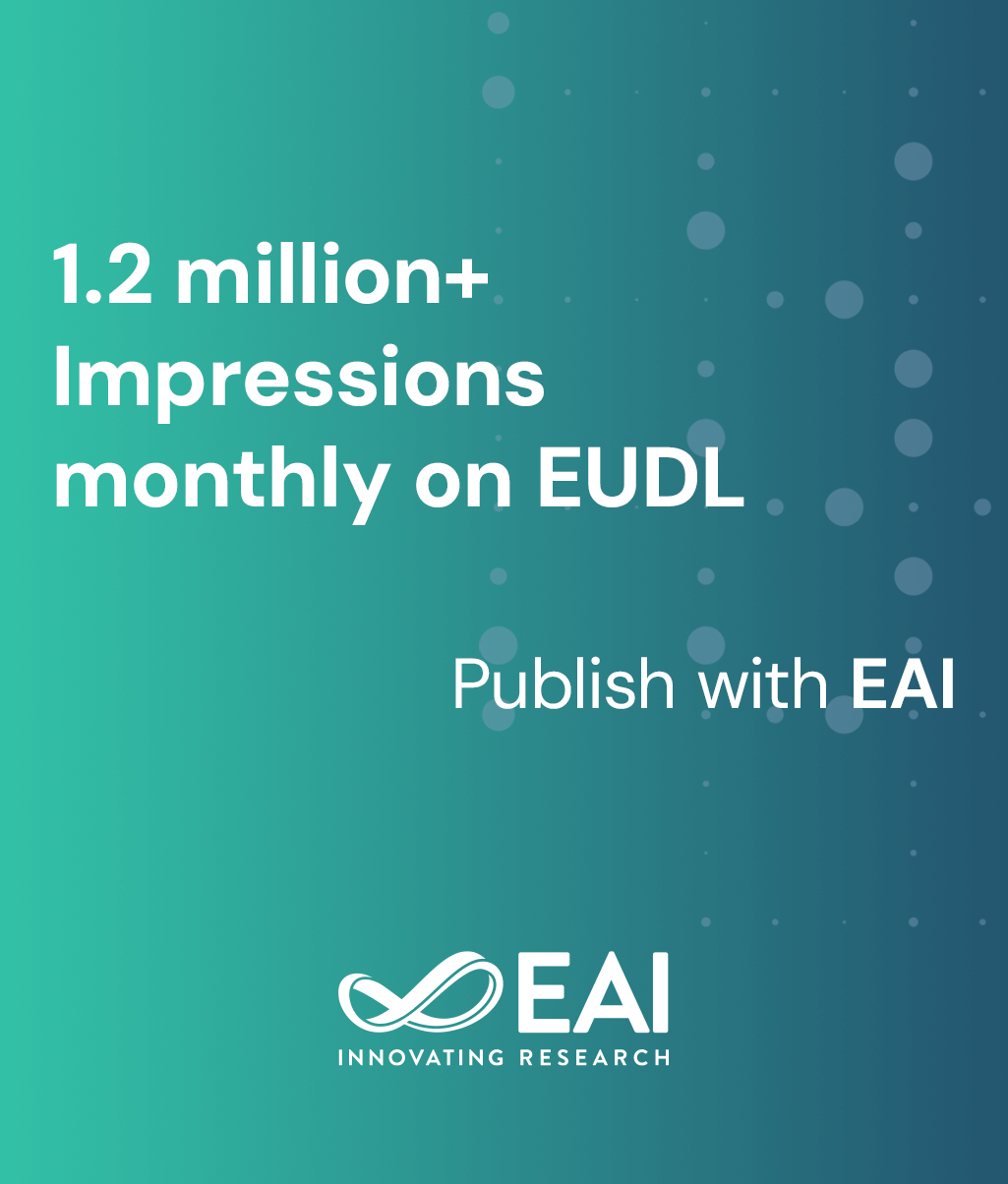
Research Article
Machine Learning based Disease and Pest detection in Agricultural Crops
@ARTICLE{10.4108/eetiot.5049, author={Balasubramaniam S and Sandra Grace Nelson and Arishma M and Anjali S Rajan and Satheesh Kumar K}, title={Machine Learning based Disease and Pest detection in Agricultural Crops}, journal={EAI Endorsed Transactions on Internet of Things}, volume={10}, number={1}, publisher={EAI}, journal_a={IOT}, year={2024}, month={2}, keywords={Machine Learning, Smart Agriculture, Disease Diagnosis, Pest Detection, Artificial Intelligence}, doi={10.4108/eetiot.5049} }
- Balasubramaniam S
Sandra Grace Nelson
Arishma M
Anjali S Rajan
Satheesh Kumar K
Year: 2024
Machine Learning based Disease and Pest detection in Agricultural Crops
IOT
EAI
DOI: 10.4108/eetiot.5049
Abstract
INTRODUCTION: Most Indians rely on agricultural work as their primary means of support, making it an essential part of the country’s economy. Disasters and the expected loss of farmland by 2050 as a result of global population expansion raise concerns about food security in that year and beyond. The Internet of Things (IoT), Big Data and Analytics are all examples of smart agricultural technologies that can help the farmers enhance their operation and make better decisions. OBJECTIVES: In this paper, machine learning based system has been developed for solving the problem of crop disease and pest prediction, focussing on the chilli crop as a case study. METHODS: The performance of the suggested system has been assessed by employing performance metrics like accuracy, Mean Squared Error (MSE), Mean Absolute Error (MAE) and Root Mean Squared Error (RMSE). RESULTS: The experimental results reveals that the proposed method obtained accuracy of 0.90, MSE of 0.37, MAE of 0.15, RMSE of 0.61 CONCLUSION: This model will predict pests and diseases and notify farmers using a combination of the Random Forest Classifier, the Ada Boost Classifier, the K Nearest Neighbour, and Logistic Regression. Random Forest is the most accurate model.
Copyright © 2024 Balasubramanium S. et al., licensed to EAI. This is an open access article distributed under the terms of the CC BYNC-SA 4.0, which permits copying, redistributing, remixing, transformation, and building upon the material in any medium so long as the original work is properly cited.