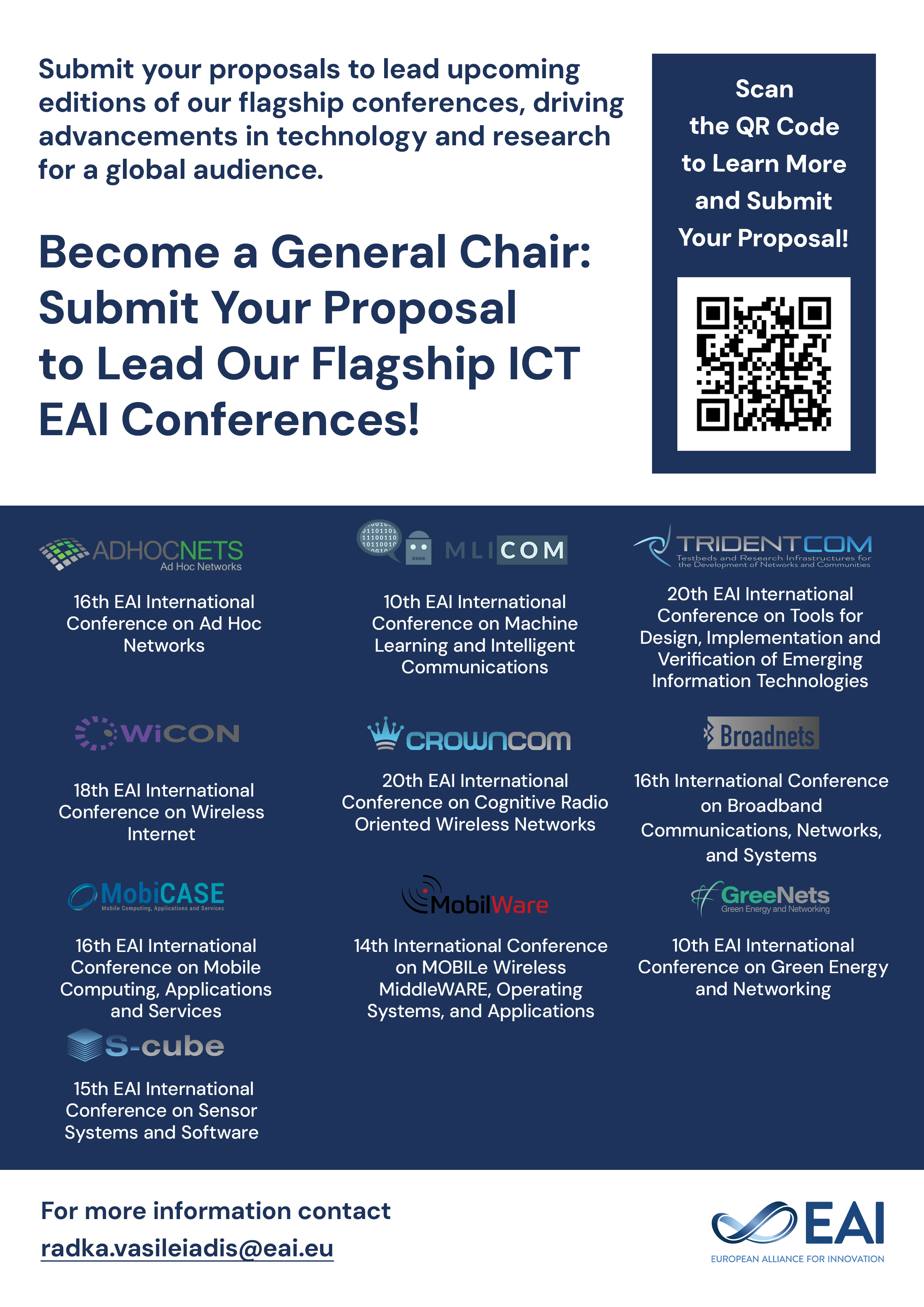
Editorial
Enhancing IoT Security through an Artificial Neural Network Approach
@ARTICLE{10.4108/eetiot.5045, author={Ahmad Sanmorino and Amirah and Rendra Gustriansyah and Shinta Puspasari}, title={Enhancing IoT Security through an Artificial Neural Network Approach}, journal={EAI Endorsed Transactions on Internet of Things}, volume={10}, number={1}, publisher={EAI}, journal_a={IOT}, year={2024}, month={10}, keywords={Internet of Things (IoT) Security, Artificial Neural Networks (ANNs), Long Short-Term Memory (LSTM)}, doi={10.4108/eetiot.5045} }
- Ahmad Sanmorino
Amirah
Rendra Gustriansyah
Shinta Puspasari
Year: 2024
Enhancing IoT Security through an Artificial Neural Network Approach
IOT
EAI
DOI: 10.4108/eetiot.5045
Abstract
This study aims to fortify Internet of Things (IoT) security through the strategic implementation of Artificial Neural Networks (ANNs). With the rapid expansion of IoT devices, traditional security measures have struggled to cope with the dynamic and complex nature of these environments. ANNs, known for their adaptability, are explored as a promising solution to enhance security. The central objective is to significantly improve the accuracy of IoT security measures by optimizing ANN architectures. Using a curated dataset with key environmental parameters, the study evaluates three ANN models—Backpropagation Neural Network (BPNN), Multilayer Perceptron (MLP), and Long Short-Term Memory (LSTM). The evaluation metrics include accuracy, precision, recall, and F1-score across different train-test splits. Results show that LSTM consistently outperforms BPNN and MLP, demonstrating superior accuracy and the ability to capture temporal dependencies within IoT security data. Implications stress the importance of aligning model selection with specific application goals, considering factors like computational efficiency. In conclusion, this research contributes valuable insights into the practical implementation of ANNs for IoT security, guiding future optimization efforts and addressing real-world deployment challenges to safeguard sensitive data and ensure system resilience in the evolving IoT landscape.
Copyright © 2024 Sanmorino et al., licensed to EAI. This is an open access article distributed under the terms of the CC BY-NC-SA 4.0, which permits copying, redistributing, remixing, transformation, and building upon the material in any medium so long as the original work is properly cited.