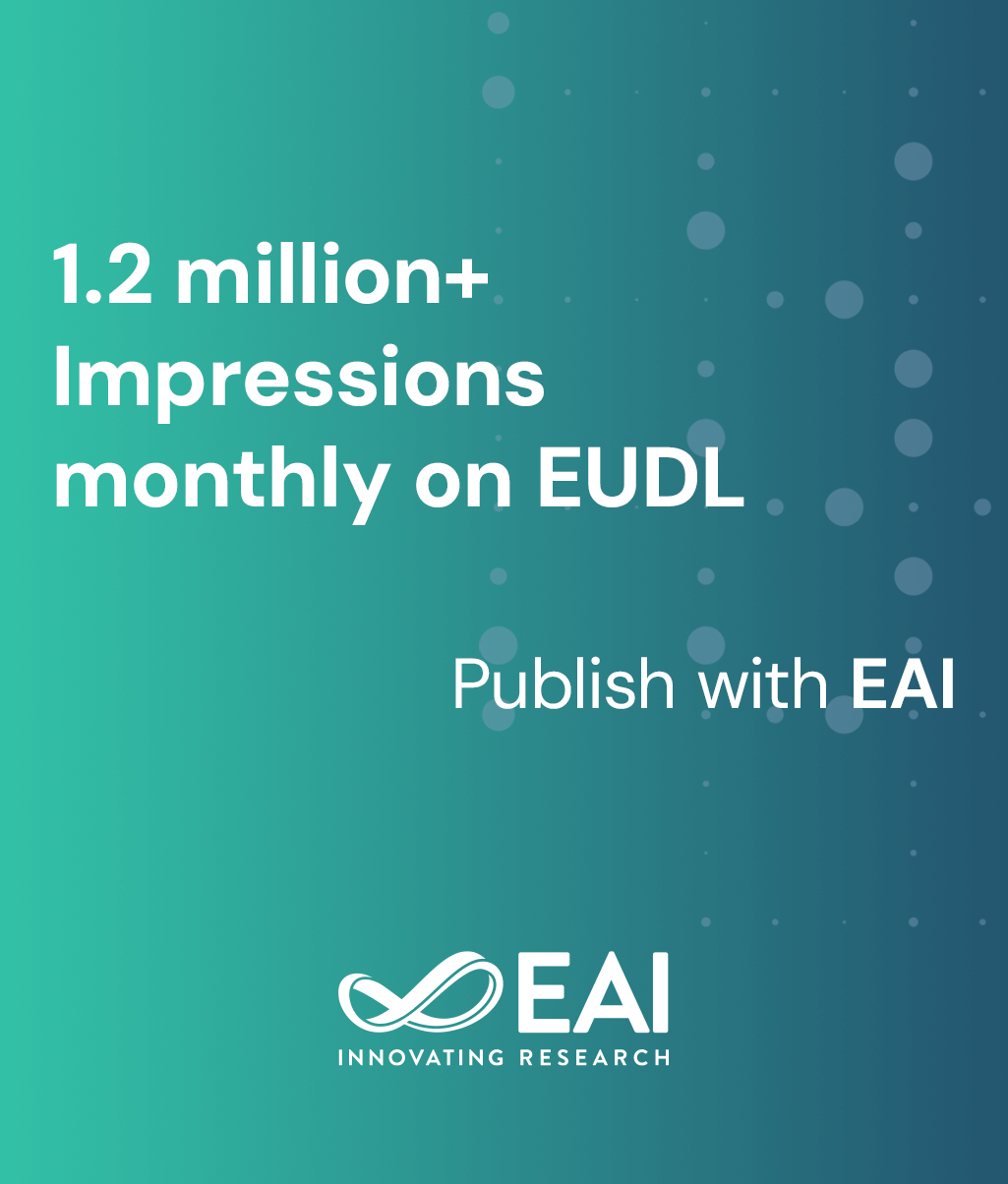
Research Article
Enhancing Agricultural Sustainability with Deep Learning: A Case Study of Cauliflower Disease Classification
@ARTICLE{10.4108/eetiot.4834, author={Nihar Ranjan Pradhan and Hritwik Ghosh and Irfan Sadiq Rahat and Janjhyam Venkata Naga Ramesh and Mannava Yesubabu}, title={Enhancing Agricultural Sustainability with Deep Learning: A Case Study of Cauliflower Disease Classification}, journal={EAI Endorsed Transactions on Internet of Things}, volume={10}, number={1}, publisher={EAI}, journal_a={IOT}, year={2024}, month={1}, keywords={Biotechnology, classification, clinical microbiology, food production, Amoeba, Euglena, Hydra, Paramecium, Rod bacteria, Spherical bacteria, Yeast, SVM, Random Forest, KNN, CNN}, doi={10.4108/eetiot.4834} }
- Nihar Ranjan Pradhan
Hritwik Ghosh
Irfan Sadiq Rahat
Janjhyam Venkata Naga Ramesh
Mannava Yesubabu
Year: 2024
Enhancing Agricultural Sustainability with Deep Learning: A Case Study of Cauliflower Disease Classification
IOT
EAI
DOI: 10.4108/eetiot.4834
Abstract
The pivotal role of sustainable agriculture in ensuring food security and nurturing healthy farming communities is undeniable. Among the numerous challenges encountered in this domain, one key hurdle is the early detection and effective treatment of diseases impacting crops, specifically cauliflower.This research provides an in-depth exploration of the use of advanced DL algorithms to perform efficient identification and classification of cauliflower diseases. The study employed and scrutinized four leading DL models: EfficientNetB3, DenseNet121, VGG19 CNN, and ResNet50, assessing their capabilities based on the accuracy of disease detection.The investigation revealed a standout performer, the EfficientNetB3 model, which demonstrated an exceptional accuracy rate of 98%. The remaining models also displayed commendable performance, with DenseNet121 and VGG19 CNN attaining accuracy rates of 81% and 84%, respectively, while ResNet50 trailed at 78%. The noteworthy performance of the EfficientNetB3 model is indicative of its vast potential to contribute to agricultural sustainability. Its ability to detect and classify cauliflower diseases accurately and promptly allows for early interventions, reducing the risk of extensive crop damage.This study contributes valuable insights to the expanding field of DL applications in agriculture. These findings are expected to guide the development of advanced agricultural monitoring systems and decision-support tools, ultimately fostering a more sustainable and productive agricultural landscape.
Copyright © 2024 N. R. Pradhan et al., licensed to EAI. This is an open access article distributed under the terms of the CC BY-NCSA 4.0, which permits copying, redistributing, remixing, transformation, and building upon the material in any medium so long as the original work is properly cited.