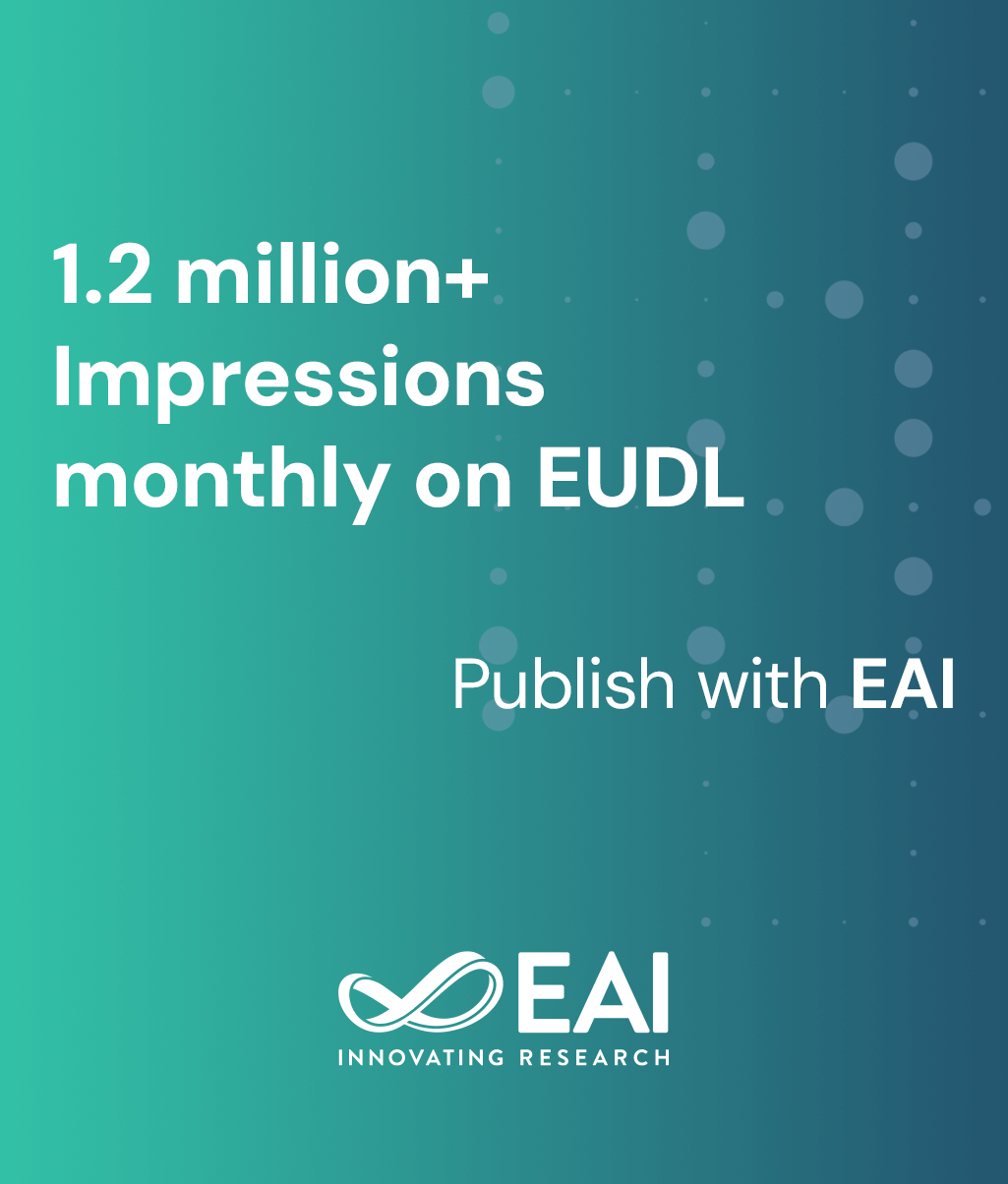
Research Article
Identification and Categorization of Yellow Rust Infection in Wheat through Deep Learning Techniques
@ARTICLE{10.4108/eetiot.4603, author={Mamatha Mandava and Surendra Reddy Vinta and Hritwik Ghosh and Irfan Sadiq Rahat}, title={Identification and Categorization of Yellow Rust Infection in Wheat through Deep Learning Techniques}, journal={EAI Endorsed Transactions on Internet of Things}, volume={10}, number={1}, publisher={EAI}, journal_a={IOT}, year={2023}, month={12}, keywords={Puccinna striiformis, wheat production, yield loss, detection, classification, deep learning, ML, Convolutional neural networks, ResNet50, DenseNet121, VGG19, wheat leaf images, data augmentation, transfer learning, precision agriculture, disease management}, doi={10.4108/eetiot.4603} }
- Mamatha Mandava
Surendra Reddy Vinta
Hritwik Ghosh
Irfan Sadiq Rahat
Year: 2023
Identification and Categorization of Yellow Rust Infection in Wheat through Deep Learning Techniques
IOT
EAI
DOI: 10.4108/eetiot.4603
Abstract
The global wheat industry faces significant challenges due to yellow rust disease, This is induced by fungus Puccinia striiformis, as it leads to substantial crop losses and economic impacts. Timely detection and classification of the disease are essential for its effective management and control. In this study, we investigate the potential of DL and ML techniques for detecting and classifying yellow rust disease in wheat. We utilize three state-of-the-art CNN models, namely ResNet50, DenseNet121, and VGG19, to analyze wheat leaf images and extract relevant features. These models were developed and refined using a large dataset of annotated wheat photos. Encompassing both healthy plants and those affected by yellow rust disease. Furthermore, we examine the effectiveness of data augmentation and transfer learning in enhancing classification performance. Our findings reveal that the DL-based CNN models surpass traditional machine learning techniques in detecting and classifying yellow rust disease in wheat. Among the tested CNN models, EfficientNetB3 demonstrates the best performance, emphasizing its suitability for large-scale and real-time monitoring of wheat crops. This research contributes to the development of precision agriculture tools, laying the groundwork for prompt intervention and management of yellow rust disease, ultimately minimizing yield loss and economic impact on wheat production.
Copyright © 2023 Mamatha Mandava et al., licensed to EAI. This is an open access article distributed under the terms of the CC BYNC-SA 4.0, which permits copying, redistributing, remixing, transformation, and building upon the material in any medium so long as the originalwork is properly cited.