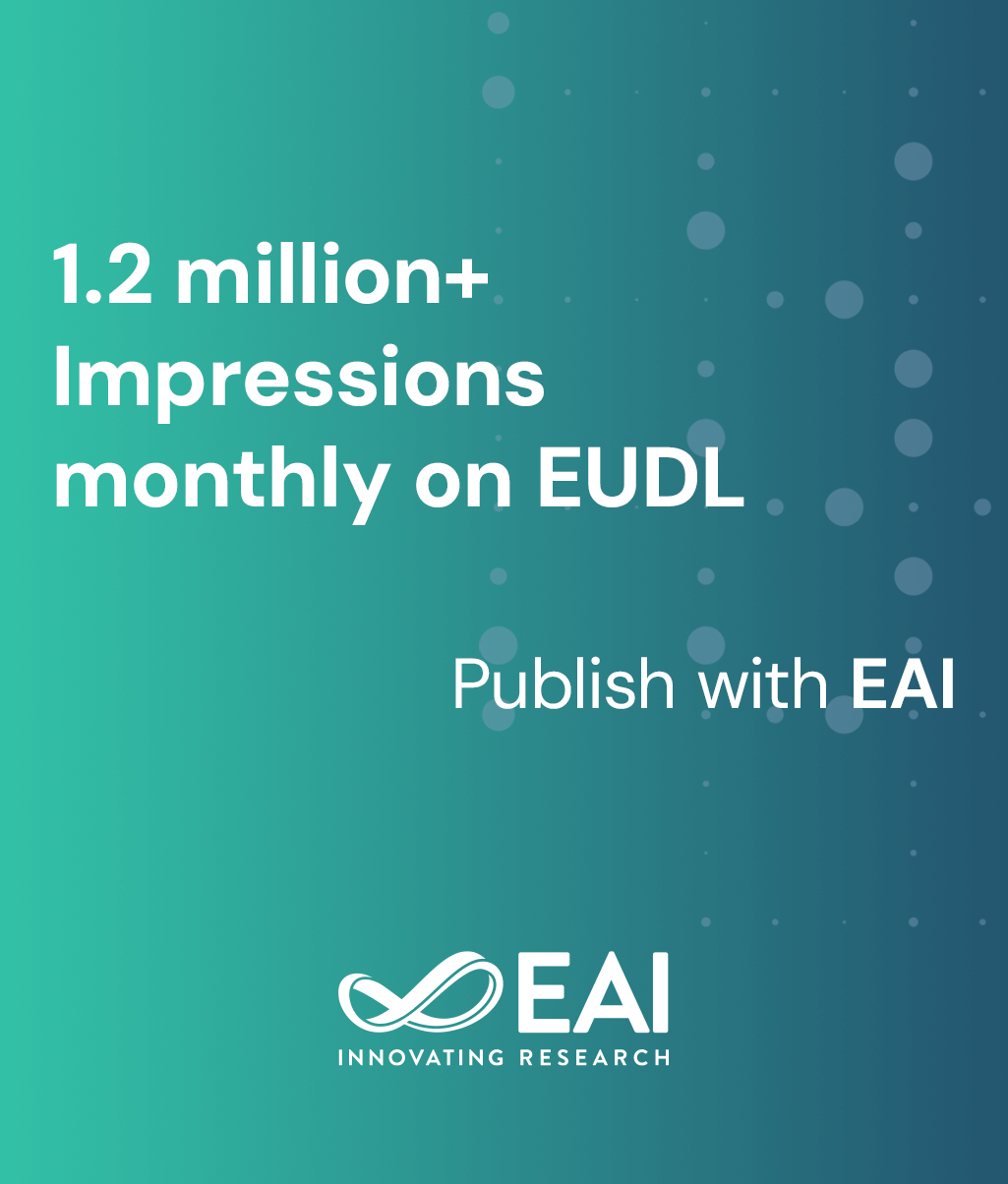
Research Article
A Hybrid Deep Learning GRU based Approach for Text Classification using Word Embedding
@ARTICLE{10.4108/eetiot.4590, author={Poluru Eswaraiah and Hussain Syed}, title={A Hybrid Deep Learning GRU based Approach for Text Classification using Word Embedding}, journal={EAI Endorsed Transactions on Internet of Things}, volume={10}, number={1}, publisher={EAI}, journal_a={IOT}, year={2023}, month={12}, keywords={Text Classification, Natural Language Processing, RNN, GRU, LSTM}, doi={10.4108/eetiot.4590} }
- Poluru Eswaraiah
Hussain Syed
Year: 2023
A Hybrid Deep Learning GRU based Approach for Text Classification using Word Embedding
IOT
EAI
DOI: 10.4108/eetiot.4590
Abstract
Text categorization has become an increasingly important issue for businesses that handle massive volumes of data generated online, and it has found substantial use in the field of NLP. The capacity to group texts into separate categories is crucial for users to effectively retain and utilize important information. Our goal is to improve upon existing recurrent neural network (RNN) techniques for text classification by creating a deep learning strategy through our study. Raising the quality of the classifications made is the main difficulty in text classification, nevertheless, as the overall efficacy of text classification is often hampered by the data semantics' inadequate context sensitivity. Our study presents a unified approach to examine the effects of word embedding and the GRU on text classification to address this difficulty. In this study, we use the TREC standard dataset. RCNN has four convolution layers, four LSTM levels, and two GRU layers. RNN, on the other hand, has four GRU layers and four LSTM levels. One kind of recurrent neural network (RNN) that is well-known for its comprehension of sequential data is the gated recurrent unit (GRU). We found in our tests that words with comparable meanings are typically found near each other in embedding spaces. The trials' findings demonstrate that our hybrid GRU model is capable of efficiently picking up word usage patterns from the provided training set. Remember that the depth and breadth of the training data greatly influence the model's effectiveness. Our suggested method performs remarkably well when compared to other well-known recurrent algorithms such as RNN, MV-RNN, and LSTM on a single benchmark dataset. In comparison to the hybrid GRU's F-measure 0.952, the proposed model's F-measure is 0.982%. We compared the performance of the proposed method to that of the three most popular recurrent neural network designs at the moment RNNs, MV-RNNs, and LSTMs, and found that the new method achieved better results on two benchmark datasets, both in terms of accuracy and error rate.
Copyright © 2023 P. Eswaraiah et al., licensed to EAI. This is an open access article distributed under the terms of the CC BY-NCSA 4.0, which permits copying, redistributing, remixing, transformation, and building upon the material in any medium so long as the original work is properly cited.