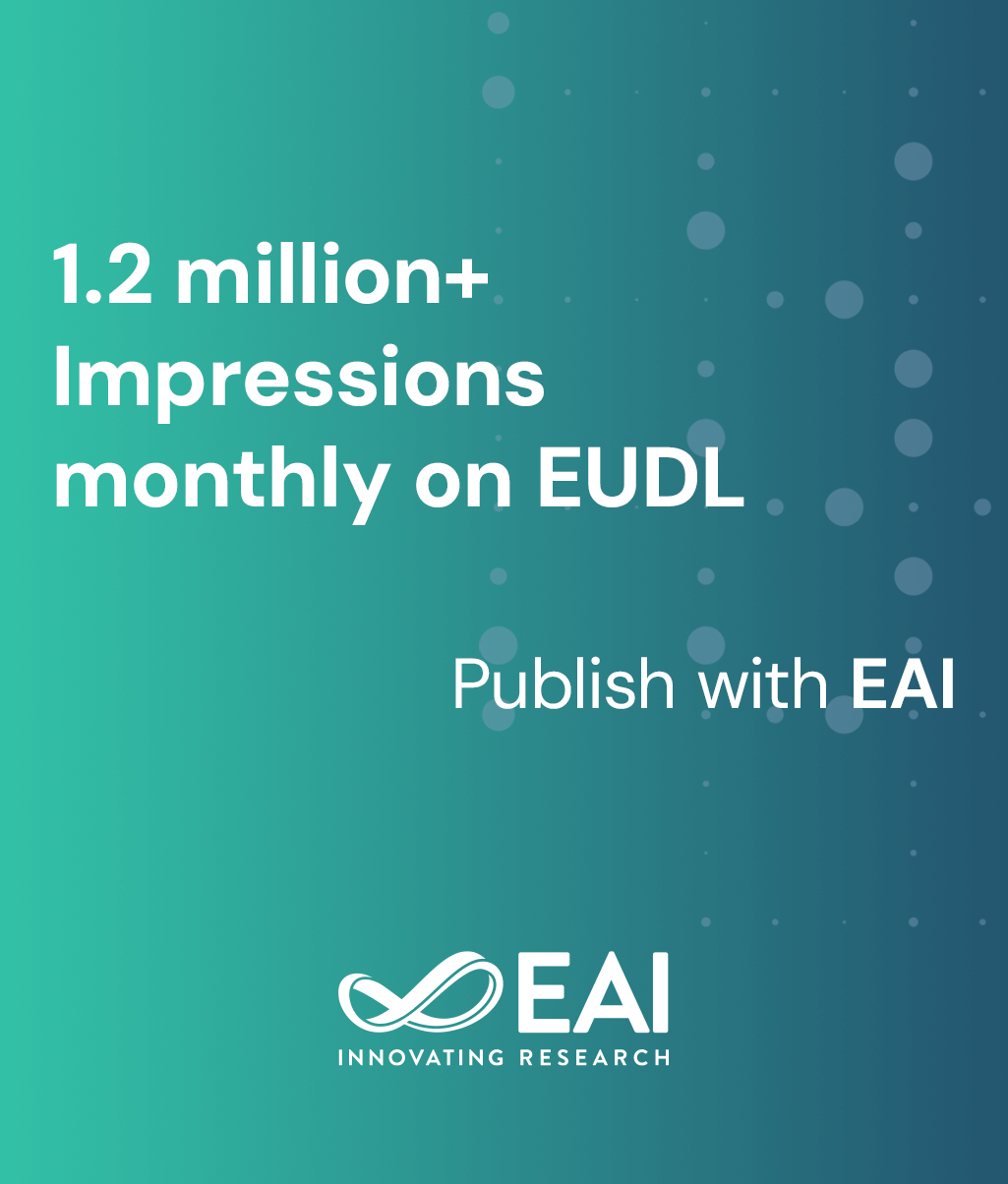
Editorial
Speech Emotion Recognition using Extreme Machine Learning
@ARTICLE{10.4108/eetiot.4485, author={Valli Madhavi Koti and Krishna Murthy and M Suganya and Meduri Sridhar Sarma and Gollakota V S S Seshu Kumar and Balamurugan N}, title={Speech Emotion Recognition using Extreme Machine Learning}, journal={EAI Endorsed Transactions on Internet of Things}, volume={10}, number={1}, publisher={EAI}, journal_a={IOT}, year={2023}, month={11}, keywords={Speech Emotion Recognition, Machine Learning Algorithm, Gaussian Mixture Model, GMM}, doi={10.4108/eetiot.4485} }
- Valli Madhavi Koti
Krishna Murthy
M Suganya
Meduri Sridhar Sarma
Gollakota V S S Seshu Kumar
Balamurugan N
Year: 2023
Speech Emotion Recognition using Extreme Machine Learning
IOT
EAI
DOI: 10.4108/eetiot.4485
Abstract
Detecting Emotion from Spoken Words (SER) is the task of detecting the underlying emotion in spoken language. It is a challenging task, as emotions are subjective and highly contextual. Machine learning algorithms have been widely used for SER, and one such algorithm is the Gaussian Mixture Model (GMM) algorithm. The GMM algorithm is a statistical model that represents the probability distribution of a random variable as a sum of Gaussian distributions. It has been widely used for speech recognition and classification tasks. In this article, we offer a method for SER using Extreme Machine Learning (EML) with the GMM algorithm. EML is a type of machine learning that uses randomization to achieve high accuracy at a low computational cost. It has been effectively utilised in various classification tasks. For the planned approach includes two steps: feature extraction and emotion classification. Cepstral Coefficients of Melody Frequency (MFCCs) are used in order to extract features. MFCCs are commonly used for speech processing and represent the spectral envelope of the speech signal. The GMM algorithm is used for emotion classification. The input features are modelled as a mixture of Gaussians, and the emotion is classified based on the likelihood of the input features belonging to each Gaussian. Measurements were taken of the suggested method on the The Berlin Database of Emotional Speech (EMO-DB) and achieved an accuracy of 74.33%. In conclusion, the proposed approach to SER using EML and the GMM algorithm shows promising results. It is a computationally efficient and effective approach to SER and can be used in various applications, such as speech-based emotion detection for virtual assistants, call centre analytics, and emotional analysis in psychotherapy.
Copyright © 2023 V. M. Koti et al., licensed to EAI. This is an open access article distributed under the terms of the CC BY-NC-SA 4.0, which permits copying, redistributing, remixing, transformation, and building upon the material in any medium so long as the original work is properly cited.