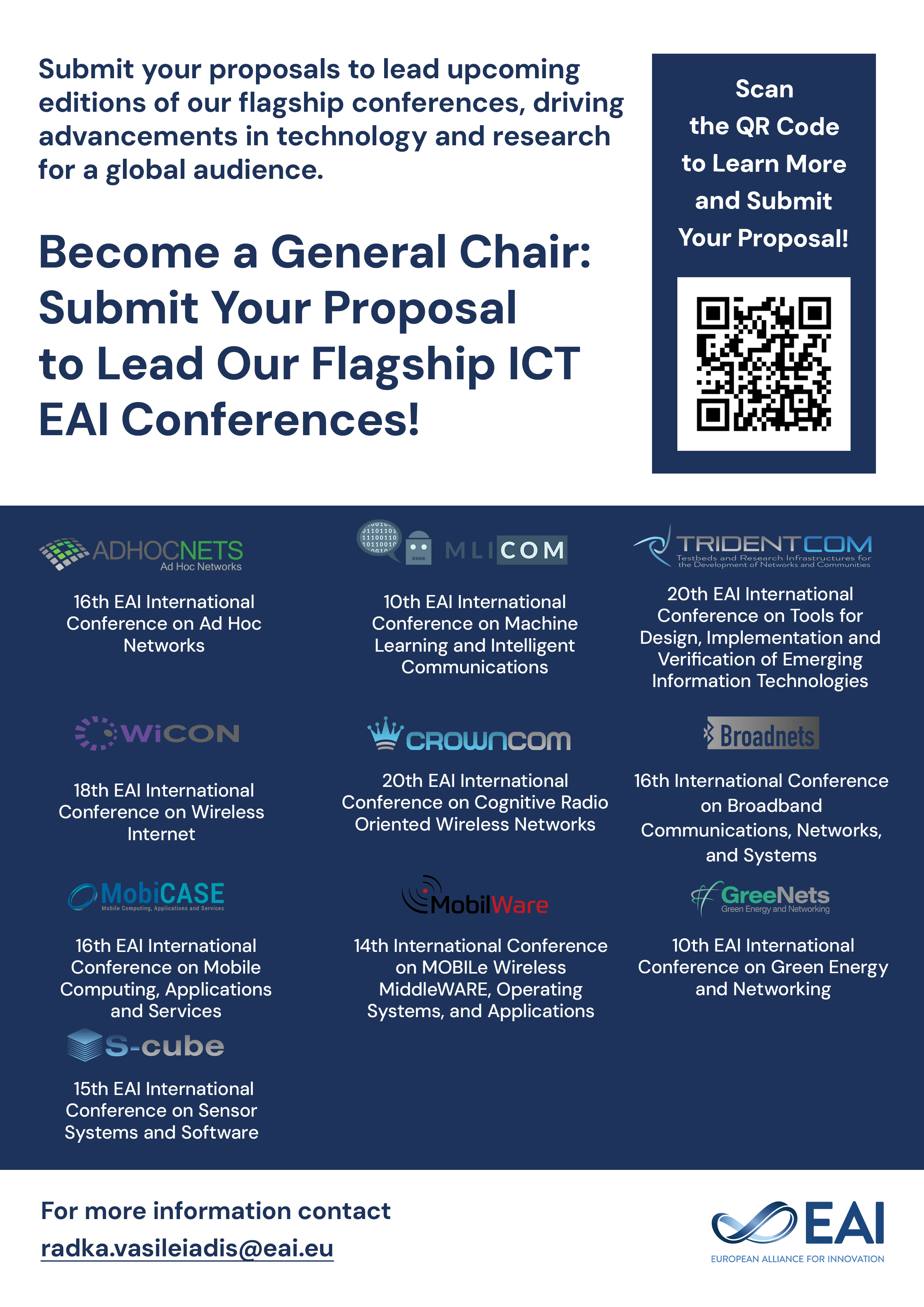
Research Article
Deciphering Microorganisms through Intelligent Image Recognition: Machine Learning and Deep Learning Approaches, Challenges, and Advancements
@ARTICLE{10.4108/eetiot.4484, author={Syed Khasim and Hritwik Ghosh and Irfan Sadiq Rahat and Kareemulla Shaik and Manava Yesubabu}, title={Deciphering Microorganisms through Intelligent Image Recognition: Machine Learning and Deep Learning Approaches, Challenges, and Advancements}, journal={EAI Endorsed Transactions on Internet of Things}, volume={10}, number={1}, publisher={EAI}, journal_a={IOT}, year={2023}, month={11}, keywords={Microorganisms, biotechnology, classification, clinical microbiology, food production, Amoeba, Euglena, Hydra, Paramecium, Rod bacteria, Spherical bacteria, Spiral bacteria, Yeast, SVM, Random Forest, KNN, CNN}, doi={10.4108/eetiot.4484} }
- Syed Khasim
Hritwik Ghosh
Irfan Sadiq Rahat
Kareemulla Shaik
Manava Yesubabu
Year: 2023
Deciphering Microorganisms through Intelligent Image Recognition: Machine Learning and Deep Learning Approaches, Challenges, and Advancements
IOT
EAI
DOI: 10.4108/eetiot.4484
Abstract
Microorganisms are pervasive and have a significant impact in various fields such as healthcare, environmental monitoring, and biotechnology. Accurate classification and identification of microorganisms are crucial for professionals in diverse areas, including clinical microbiology, agriculture, and food production. Traditional methods for analyzing microorganisms, like culture techniques and manual microscopy, can be labor-intensive, expensive, and occasionally inadequate due to morphological similarities between different species. As a result, there is an increasing need for intelligent image recognition systems to automate microorganism classification procedures with minimal human involvement. In this paper, we present an in-depth analysis of ML and DL perspectives used for the precise recognition and classification of microorganism images, utilizing a dataset comprising eight distinct microorganism types: Spherical bacteria, Amoeba, Hydra, Paramecium, Rod bacteria, Spiral bacteria, Euglena and Yeast. We employed several ml algorithms including SVM, Random Forest, and KNN, as well as the deep learning algorithm CNN. Among these methods, the highest accuracy was achieved using the CNN approach. We delve into current techniques, challenges, and advancements, highlighting opportunities for further progress.
Copyright © 2023 S. Khasim et al., licensed to EAI. This is an open access article distributed under the terms of the CC BY-NC-SA 4.0, which permits copying, redistributing, remixing, transformation, and building upon the material in any medium so long as the original work is properly cited.