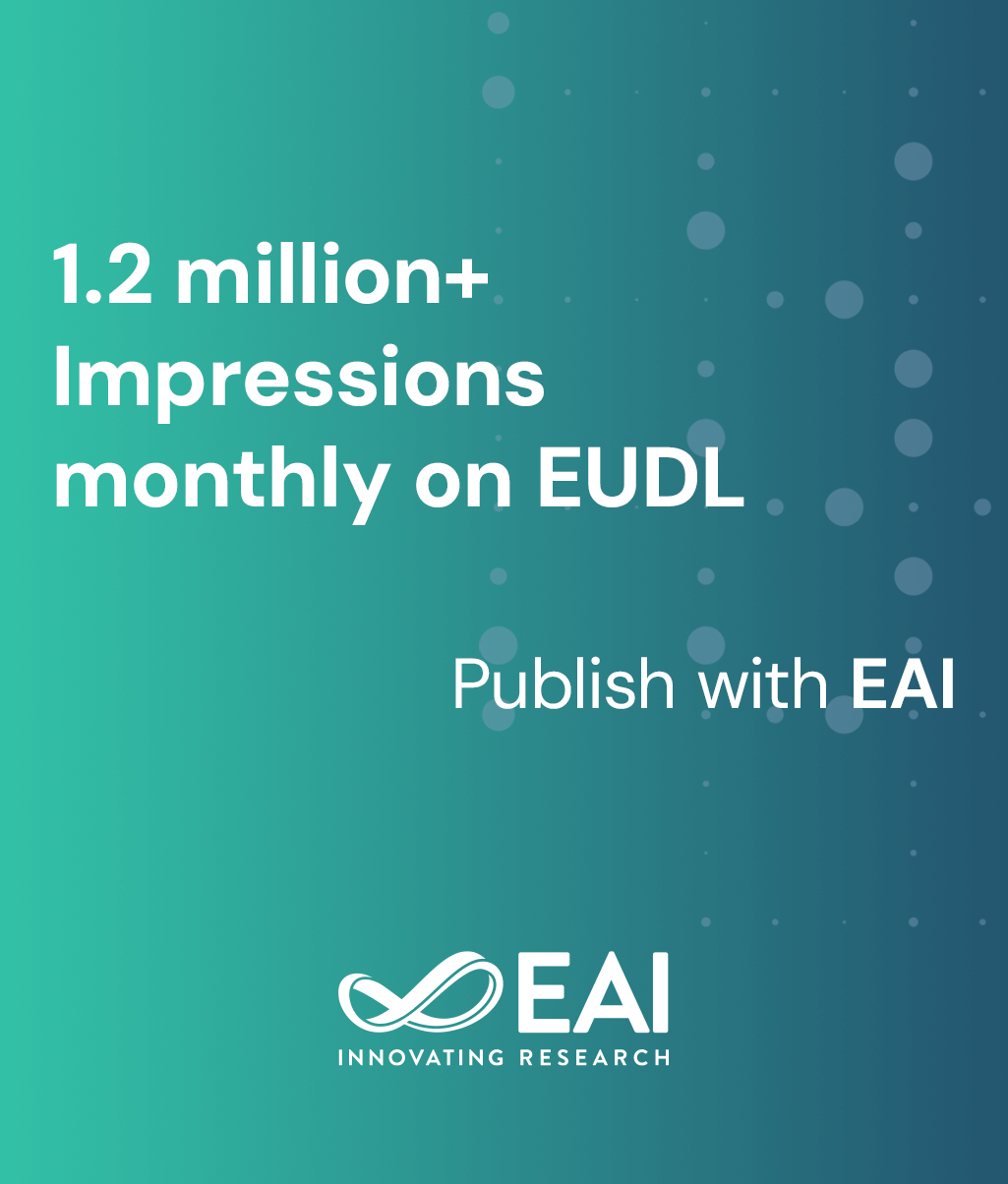
Research Article
Big Data and Knowledge Graph Based Fault Diagnosis for Electric Power Systems
@ARTICLE{10.4108/eetinis.v9i32.1268, author={Yuzhong Zhou and Zhengping Lin and Liang Tu and Yufei Song and Zhengrong Wu}, title={Big Data and Knowledge Graph Based Fault Diagnosis for Electric Power Systems}, journal={EAI Endorsed Transactions on Industrial Networks and Intelligent Systems}, volume={9}, number={32}, publisher={EAI}, journal_a={INIS}, year={2022}, month={6}, keywords={Big data, knowledge graph, fault detection, electric power systems}, doi={10.4108/eetinis.v9i32.1268} }
- Yuzhong Zhou
Zhengping Lin
Liang Tu
Yufei Song
Zhengrong Wu
Year: 2022
Big Data and Knowledge Graph Based Fault Diagnosis for Electric Power Systems
INIS
EAI
DOI: 10.4108/eetinis.v9i32.1268
Abstract
Fault detection plays an important role in the daily maintenance of power electric system. Big data and knowledge graph (KG) have been proposed by researchers to solve many problems in industrial Internet of Things, which also give lots of potentials in improving the performance of fault detection for electric power systems. In particular, this paper analyzes a distributed knowledge graph framework for fault detection in the electric power systems, where multiple devices train their local detection models used for fault detection assisted with a central server. Each device owns its local data set composed of historical fault information and current device state, which can be used to train a local model for fault detection. To enhance the detection performance, the distributed devices interact with each other in the KG framework, where the devices ought to achieve the regional computation in addition to the model aggregation within a specified latency threshold. Through searching for the vibrant qualities together with determined ability at the devices, we enhance the knowledge graph framework by the optimum variety of energetic devices together with the restriction of latency as well as data transmission. Particularly, two data transmission bandwidth allocation (BA) schemes are developed for the distributed knowledge graph framework, through which scheme I is actually bared after the instantaneous device state information (DSI), and scheme II utilizes particle swarm optimization (PSO) technique along with the statistical DSI. The results of simulation on the examination as well as convergence are lastly demonstrated to show the advantages of the proposed distributed KG framework in the fault detection for the electric power systems.
Copyright © 2022 Yuzhong Zhou et al., licensed to EAI. This is an open access article distributed under the terms of the Creative Commons Attribution license, which permits unlimited use, distribution and reproduction in any medium so long as the original work is properly cited.