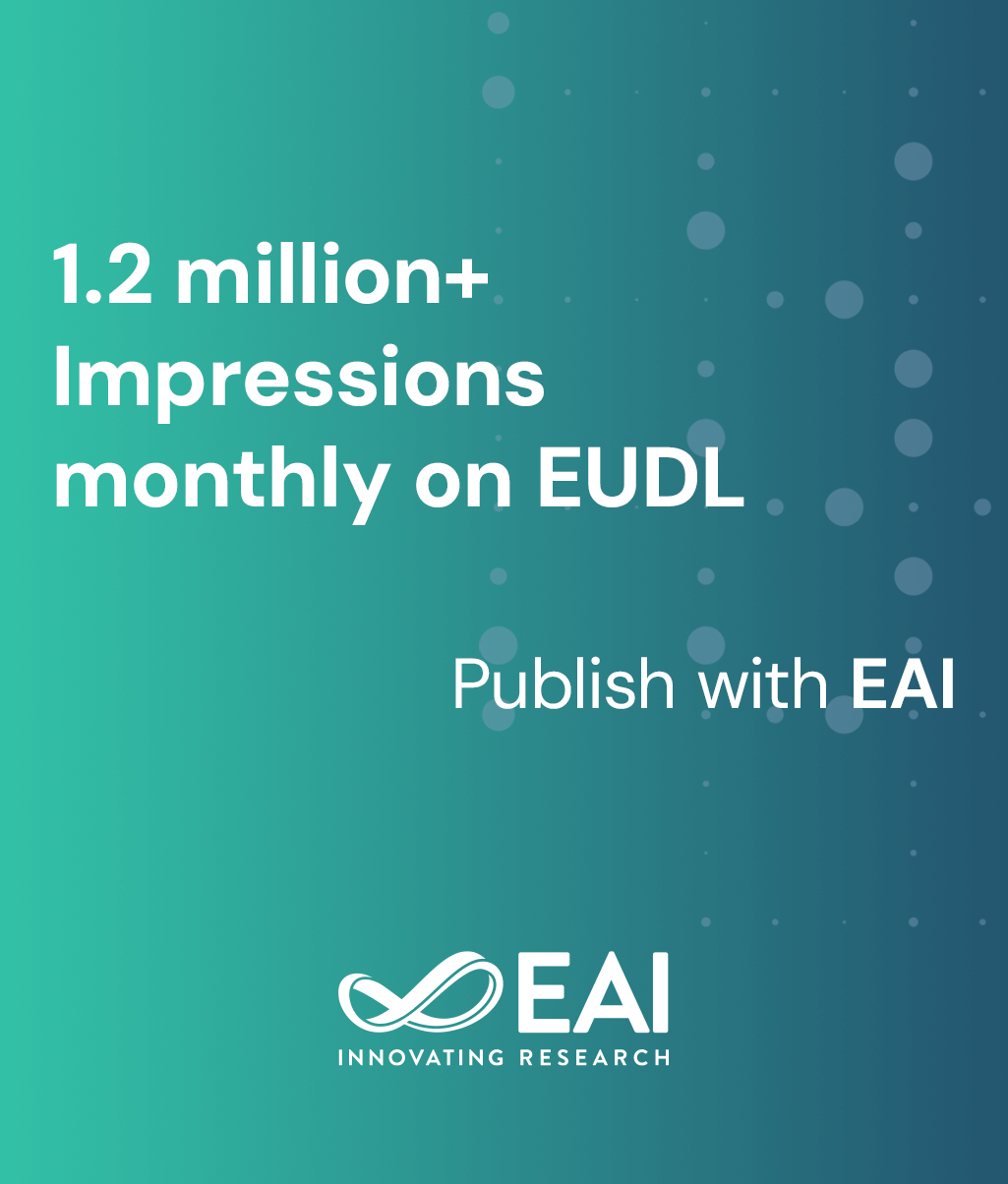
Research Article
A novel approach for graph-based real-time anomaly detection from dynamic network data listened by Wireshark
@ARTICLE{10.4108/eetinis.v12i2.7616, author={Muhammed Onur Kaya and Mehmet Ozdem and Resul Das}, title={A novel approach for graph-based real-time anomaly detection from dynamic network data listened by Wireshark}, journal={EAI Endorsed Transactions on Industrial Networks and Intelligent Systems}, volume={12}, number={2}, publisher={EAI}, journal_a={INIS}, year={2025}, month={4}, keywords={Cyber Attacks, Information Security, Graph Visualization, Temporal Dynamic Networks, Wireshark}, doi={10.4108/eetinis.v12i2.7616} }
- Muhammed Onur Kaya
Mehmet Ozdem
Resul Das
Year: 2025
A novel approach for graph-based real-time anomaly detection from dynamic network data listened by Wireshark
INIS
EAI
DOI: 10.4108/eetinis.v12i2.7616
Abstract
This paper presents a novel approach for real-time anomaly detection and visualization of dynamic network data using Wireshark, globally's most widely utilized network analysis tool. As the complexity and volume of network data continue to grow, effective anomaly detection has become essential for maintaining network performance and enhancing security. Our method leverages Wireshark’s robust data collection and analysis capabilities to identify anomalies swiftly and accurately. In addition to detection, we introduce innovative visualization techniques that facilitate the intuitive representation of detected anomalies, allowing network administrators to comprehend network conditions and make informed decisions quickly. The results of our study demonstrate significant improvements in both the efficacy of anomaly detection and the practical applicability of visualization tools in real-time scenarios. This research contributes valuable insights into network security and management, highlighting the importance of integrating advanced analytical methods with effective visualization strategies to enhance the overall management of dynamic networks.
Copyright © 2025 M. O. Kaya et al., licensed to EAI. This is an open access article distributed under the terms of the CC BY-NC-SA 4.0, which permits copying, redistributing, remixing, transformation, and building upon the material in any medium so long as the original work is properly cited.