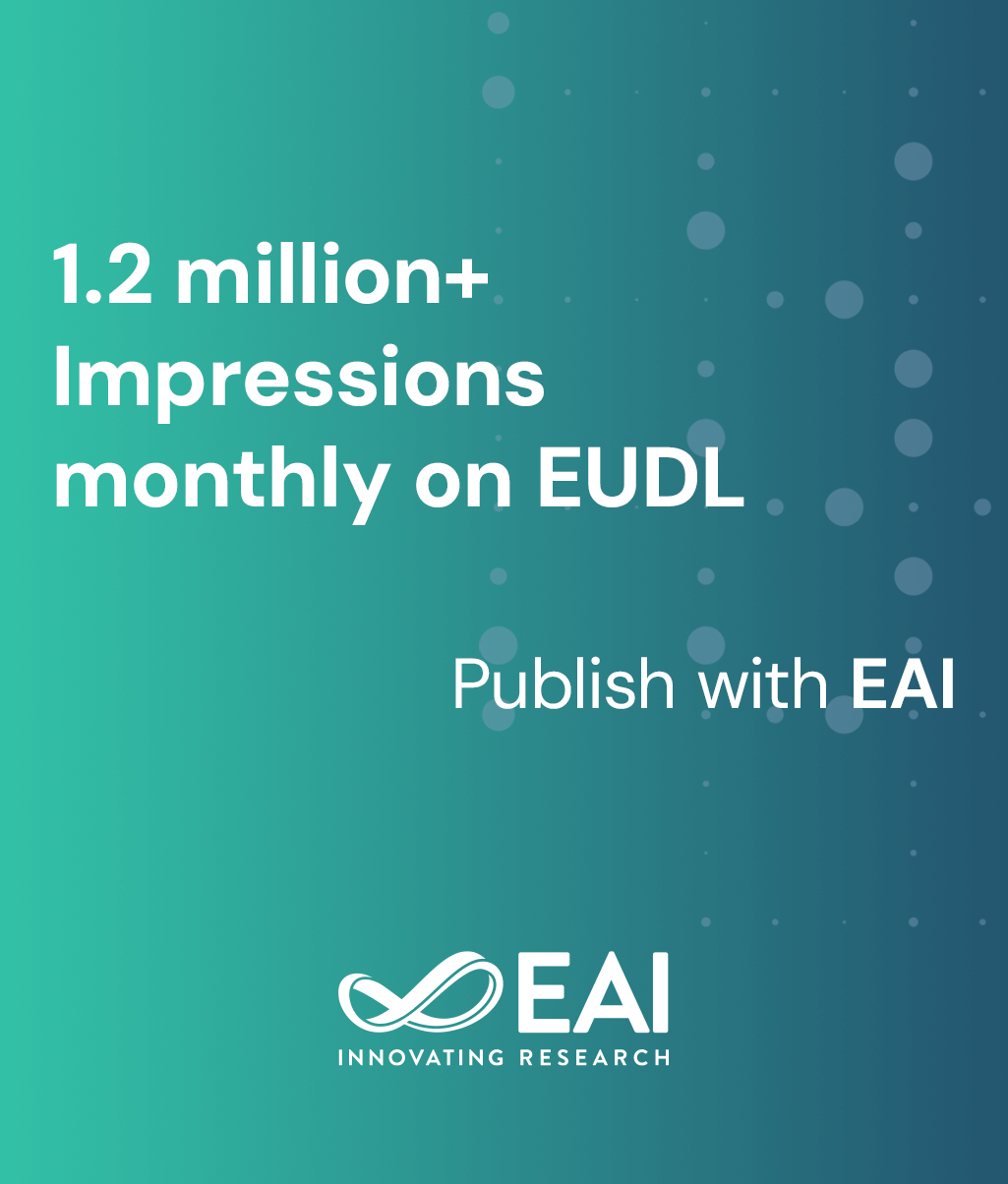
Research Article
Single-level Discrete Two Dimensional Wavelet Transform Based Multiscale Deep Learning Framework for Two-Wheeler Helmet Detection
@ARTICLE{10.4108/eetinis.v12i2.7612, author={Amrutha Annadurai and Manas Ranjan Prusty and Trilok Nath Pandey and Subhra Rani Patra}, title={Single-level Discrete Two Dimensional Wavelet Transform Based Multiscale Deep Learning Framework for Two-Wheeler Helmet Detection}, journal={EAI Endorsed Transactions on Industrial Networks and Intelligent Systems}, volume={12}, number={2}, publisher={EAI}, journal_a={INIS}, year={2025}, month={3}, keywords={Multi Scale CNN, Long Short-Term Memory, Discrete Wavelet Transform, Helmet Detection}, doi={10.4108/eetinis.v12i2.7612} }
- Amrutha Annadurai
Manas Ranjan Prusty
Trilok Nath Pandey
Subhra Rani Patra
Year: 2025
Single-level Discrete Two Dimensional Wavelet Transform Based Multiscale Deep Learning Framework for Two-Wheeler Helmet Detection
INIS
EAI
DOI: 10.4108/eetinis.v12i2.7612
Abstract
INTRODUCTION: A robust method is proposed in this paper to detect helmet usage in two-wheeler riders to enhance road safety. OBJECTIVES: This involves a custom made dataset that contains 1000 images captured under diverse real-world scenarios, including variations in helmet size, colour, and lighting conditions. This dataset has two classes namely with helmet and without helmet. METHODS: The proposed helmet classification approach utilizes the Multi-Scale Deep Convolutional Neural Network (CNN) framework cascaded with Long Short-Term Memory (LSTM) network. Initially the Multi-Scale Deep CNN extracts modes by applying Single-level Discrete 2D Wavelet Transform (dwt2) to decompose the original images. In particular, four different modes are used for segmenting a single image namely approximation, horizontal detail, vertical detail and diagonal detail. After feeding the segmented images into a Multi-Scale Deep CNN model, it is cascaded with an LSTM network. RESULTS: The proposed model achieved accuracies of 99.20% and 95.99% using both 5-Fold Cross-Validation (CV) and Hold-out CV methods, respectively. CONCLUSION: This result was better than the CNN-LSTM, dwt2-LSTM and a tailor made CNN model.
Copyright © 2025 A. Annadurai et al., licensed to EAI. This is an open access article distributed under the terms of the CC BY-NCSA 4.0, which permits copying, redistributing, remixing, transformation, and building upon the material in any medium so long as the original work is properly cited.