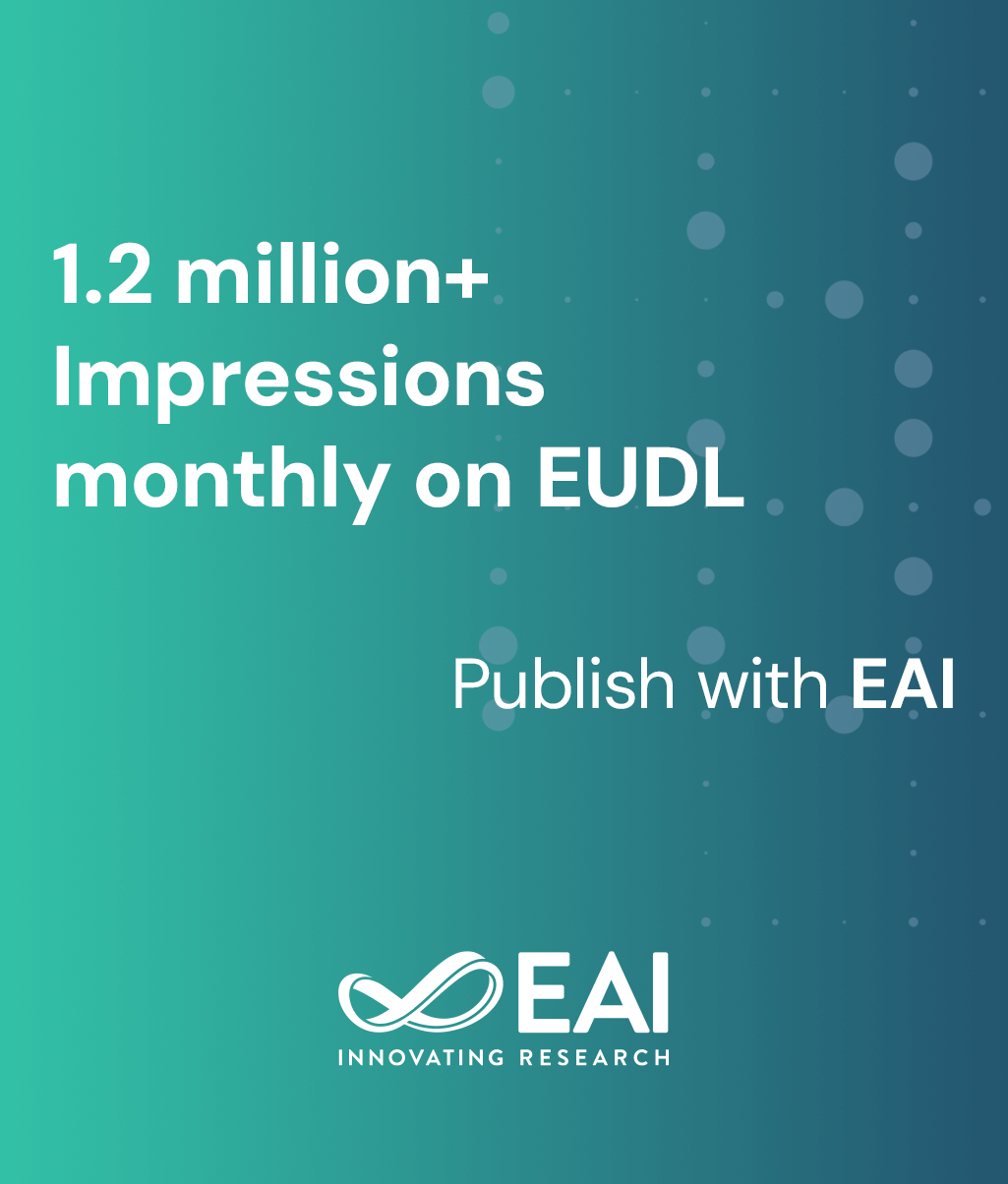
Research Article
Transformer Based Ship Detector: An Improvement on Feature Map and Tiny Training Set
@ARTICLE{10.4108/eetinis.v12i1.6794, author={Duc-Dat Ngo and Van-Linh Vo and My-Ha Le and Hoc-Phan and Manh Hung Nguyen}, title={Transformer Based Ship Detector: An Improvement on Feature Map and Tiny Training Set}, journal={EAI Endorsed Transactions on Industrial Networks and Intelligent Systems}, volume={12}, number={1}, publisher={EAI}, journal_a={INIS}, year={2024}, month={11}, keywords={Object Detection, Maritime border security, Deformable DETR}, doi={10.4108/eetinis.v12i1.6794} }
- Duc-Dat Ngo
Van-Linh Vo
My-Ha Le
Hoc-Phan
Manh Hung Nguyen
Year: 2024
Transformer Based Ship Detector: An Improvement on Feature Map and Tiny Training Set
INIS
EAI
DOI: 10.4108/eetinis.v12i1.6794
Abstract
The exponential increment of commodity exchange has raised the need for maritime border security in recent years. One of the most critical tasks for naval border security is ship detection inside and outside the territorial sea. Conventionally, the task requires a substantial human workload. Fortunately, with the rapid growth of the digital camera and deep-learning technique, computer programs can handle object detection tasks well enough to replace human labor. Therefore, this paper studies how to apply recent state-of-the-art deep-learning networks to the ship detection task. We found that with a suitable number of object queries, the Deformable-DETR method will improve the performance compared to the state-of-the-art ship detector. Moreover, comprehensive experiments on different scale datasets prove that the technique can significantly improve the results when the training sample is limited. Last but not least, feature maps given by the method will focus well on key objects in the image.
Copyright © 2025 Duc-Dat Ngo et al., licensed to EAI. This is an open access article distributed under the terms of the CC BY-NC-SA 4.0, which permits copying, redistributing, remixing, transformation, and building upon the material in any medium so long as the original work is properly cited.