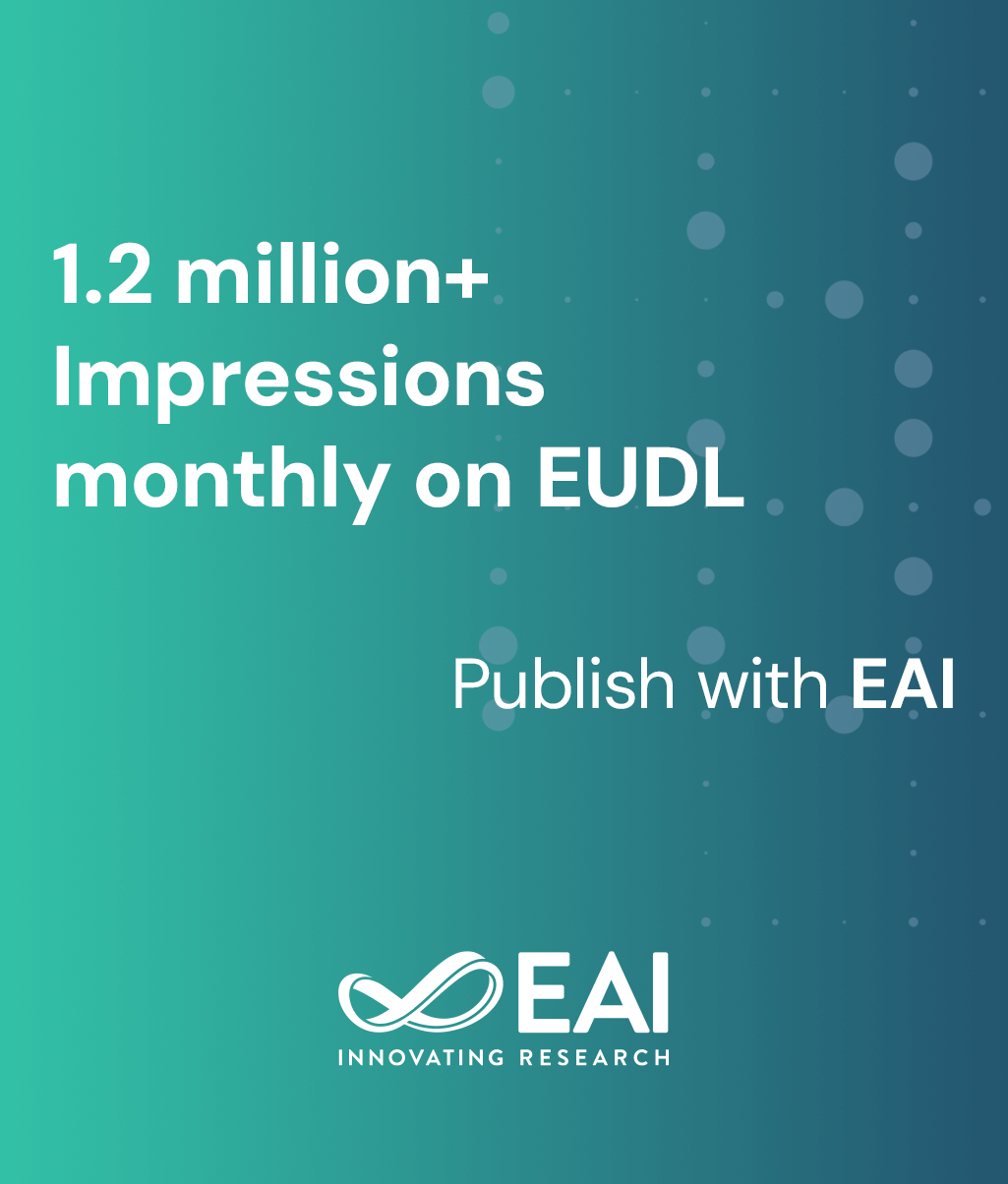
Research Article
Predicting the Severity of COVID-19 Pneumonia from Chest X-Ray Images: A Convolutional Neural Network Approach
@ARTICLE{10.4108/eetinis.v12i1.6240, author={Thien B. Nguyen-Tat and Viet-Trinh Tran-Thi and Vuong M. Ngo}, title={Predicting the Severity of COVID-19 Pneumonia from Chest X-Ray Images: A Convolutional Neural Network Approach}, journal={EAI Endorsed Transactions on Industrial Networks and Intelligent Systems}, volume={12}, number={1}, publisher={EAI}, journal_a={INIS}, year={2024}, month={12}, keywords={Pneumonia Severity, COVID-19, Convolutional Neural Networks, Classification}, doi={10.4108/eetinis.v12i1.6240} }
- Thien B. Nguyen-Tat
Viet-Trinh Tran-Thi
Vuong M. Ngo
Year: 2024
Predicting the Severity of COVID-19 Pneumonia from Chest X-Ray Images: A Convolutional Neural Network Approach
INIS
EAI
DOI: 10.4108/eetinis.v12i1.6240
Abstract
This study addresses significant limitations of previous works based on the Brixia and COVIDGR datasets, which primarily provided qualitative lung injury scores and focused mainly on detecting mild and moderate cases. To bridge these critical gaps, we developed a unified and comprehensive analytical framework that accurately assesses COVID-19-induced lung injuries across four levels: Normal, Mild, Moderate, and Severe. This approach’s core is a meticulously curated, balanced dataset comprising 9,294 high-quality chest X-ray images. Notably, this dataset has been made widely available to the research community, fostering collaborative efforts and enhancing the precision of lung injury classification at all severity levels. To validate the framework’s effectiveness, we conducted an in-depth evaluation using advanced deep learning models, including VGG16, RegNet, DenseNet, MobileNet, EfficientNet, and Vision Transformer (ViT), on this dataset. The top-performing model was further enhanced by optimizing additional fully connected layers and adjusting weights, achieving an outstanding sensitivity of 94.38%. These results affirm the accuracy and reliability of the proposed solution and demonstrate its potential for broad application in clinical practice. Our study represents a significant step forward in developing AI-powered diagnostic tools, contributing to the timely and precise diagnosis of COVID-19 cases. Furthermore, our dataset and methodological framework hold the potential to serve as a foundation for future research, paving the way for advancements in the detection and classification of respiratory diseases with higher accuracy and efficiency.
Copyright © 2024 Thien B. Nguyen-Tat et al., licensed to EAI. This is an open access article distributed under the terms of the CC BY-NC-SA 4.0, which permits copying, redistributing, remixing, transformation, and building upon the material in any medium so long as the original work is properly cited.