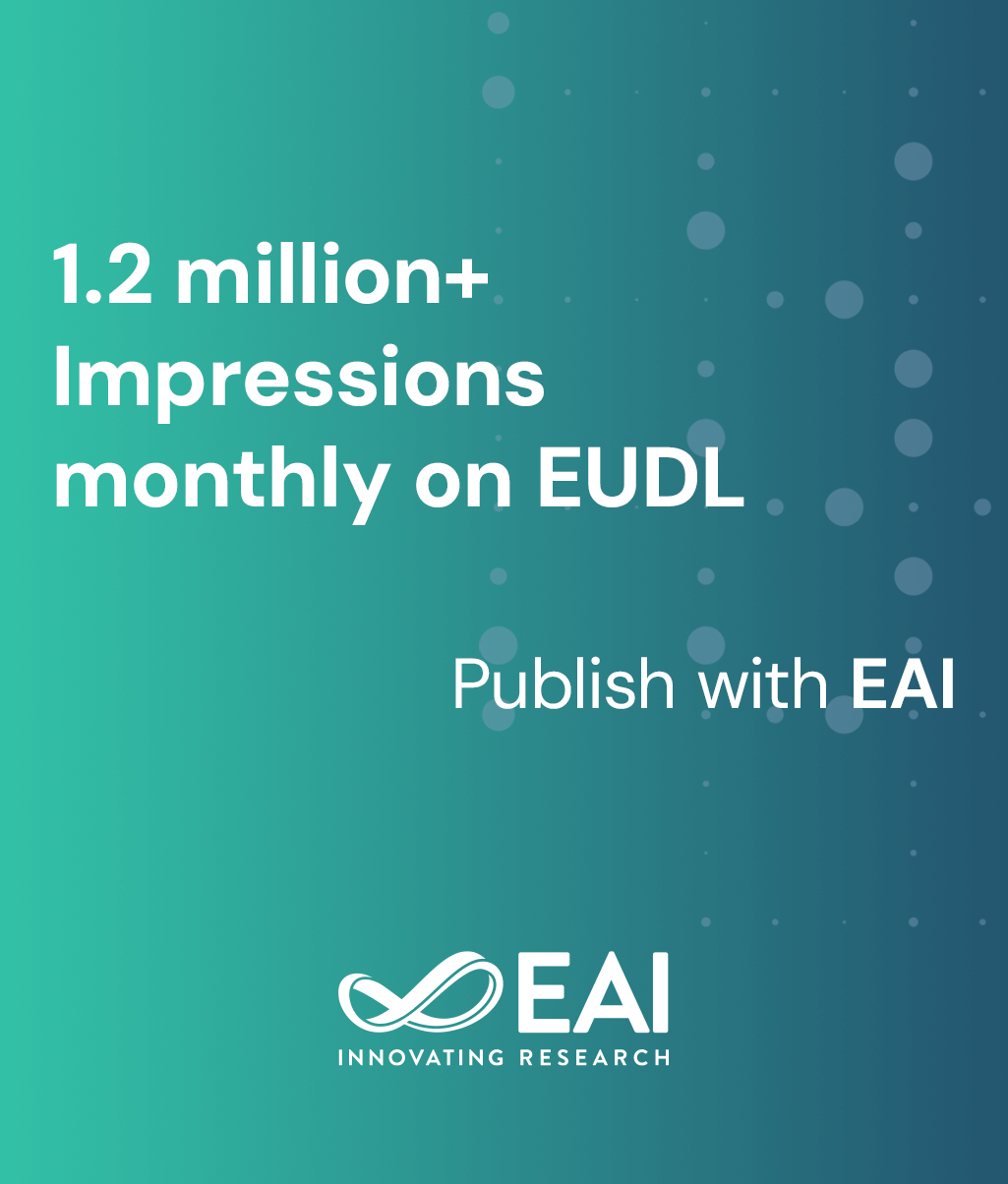
Research Article
Drug classification system based on drug composition and usage instructions
@ARTICLE{10.4108/eetinis.v12i1.5995, author={Hoang-Dieu Vu and Vu-Hien Pham and Quang-Dung Le}, title={Drug classification system based on drug composition and usage instructions}, journal={EAI Endorsed Transactions on Industrial Networks and Intelligent Systems}, volume={12}, number={1}, publisher={EAI}, journal_a={INIS}, year={2024}, month={12}, keywords={Drug classification, NLP, Drug Composition}, doi={10.4108/eetinis.v12i1.5995} }
- Hoang-Dieu Vu
Vu-Hien Pham
Quang-Dung Le
Year: 2024
Drug classification system based on drug composition and usage instructions
INIS
EAI
DOI: 10.4108/eetinis.v12i1.5995
Abstract
This study presents a natural language processing (NLP) approach to classify drugs based on compositional and usage descriptions. NLP techniques including text preprocessing, word embedding, and deep learning models were applied to a Vietnamese drug dataset. Traditional machine learning models like Support Vector Machines (SVM) and deep models including Bidirectional Long Short-Term Memory (BiLSTM) and PhoBERT were evaluated. Besides, since there is a limitation in the information of our own collected data, some data augmentation techniques were applied to increase the variation of the dataset. Results show PhoBERT achieving 95% accuracy, highlighting the benefits of transferring knowledge from large language models. Errors primarily occurred between similar drug categories, suggesting taxonomy refinement could improve performance. In summary, an automated drug classification framework was developed leveraging state-of- the-art NLP, validating the feasibility of analyzing drug data at scale and aiding therapeutic understanding. This supports NLP’s potential in pharmacovigilance applications.
Copyright © 2025 Hoang-Dieu Vu et al., licensed to EAI. This is an open access article distributed under the terms of the CC BY-NC-SA 4.0, which permits copying, redistributing, remixing, transformation, and building upon the material in any medium so long as the original work is properly cited.