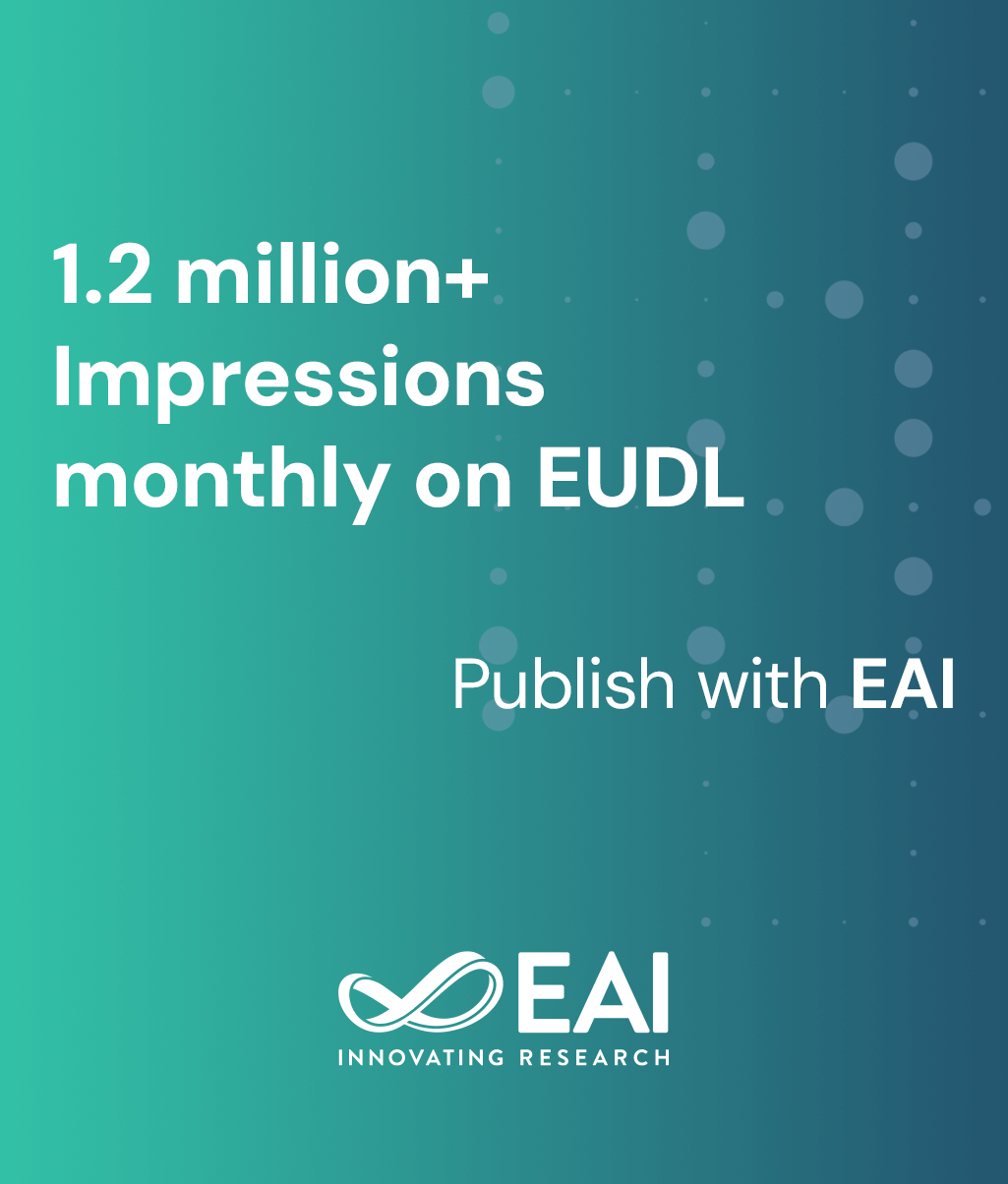
Research Article
Emotional Inference from Speech Signals Informed by Multiple Stream DNNs Based Non-Local Attention Mechanism
@ARTICLE{10.4108/eetinis.v11i4.4734, author={Manh-Hung Ha and Duc-Chinh Nguyen and Long Quang Chan and Oscal T.C. Chen}, title={Emotional Inference from Speech Signals Informed by Multiple Stream DNNs Based Non-Local Attention Mechanism}, journal={EAI Endorsed Transactions on Industrial Networks and Intelligent Systems}, volume={11}, number={4}, publisher={EAI}, journal_a={INIS}, year={2024}, month={8}, keywords={Convolution Neural Network, LSTM, Attention mechanism, Emotion, Classification}, doi={10.4108/eetinis.v11i4.4734} }
- Manh-Hung Ha
Duc-Chinh Nguyen
Long Quang Chan
Oscal T.C. Chen
Year: 2024
Emotional Inference from Speech Signals Informed by Multiple Stream DNNs Based Non-Local Attention Mechanism
INIS
EAI
DOI: 10.4108/eetinis.v11i4.4734
Abstract
It is difficult to determine whether a person is depressed due to the symptoms of depression not being apparent. However, the voice can be one of the ways in which we can acknowledge signs of depression. Understanding human emotions in natural language plays a crucial role for intelligent and sophisticated applications. This study proposes deep learning architecture to recognize the emotions of the speaker via audio signals, which can help diagnose patients who are depressed or prone to depression, so that treatment and prevention can be started as soon as possible. Specifically, Mel-frequency cepstral coefficients (MFCC) and Short Time Fourier Transform (STFT) are adopted to extract features from the audio signal. The multiple streams of the proposed DNNs model, including CNN-LSTM based on an attention mechanism, are discussed within this research. Leveraging a pretrained model, the proposed experimental results yield an accuracy rate of 93.2% on the EmoDB dataset. Further optimization remains a potential avenue for future development. It is hoped that this research will contribute to potential application in the fields of medical treatment and personal well-being.
Copyright © 2024 Manh-Hung Ha et al., licensed to EAI. This is an open access article distributed under the terms of the Creative Commons Attribution license, which permits unlimited use, distribution and reproduction in any medium so long as the original work is properly cited.