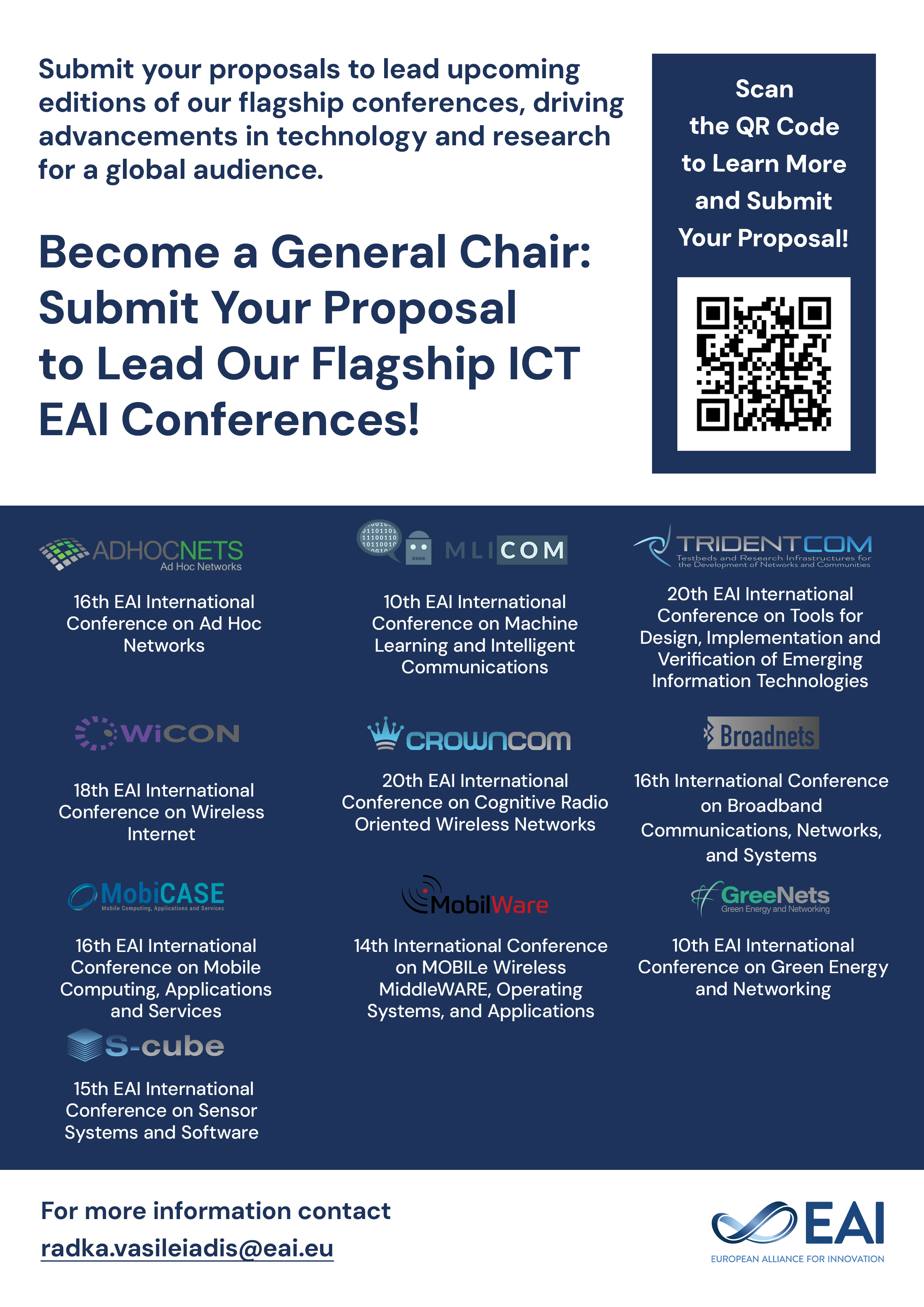
Research Article
Bi-objective model for community detection in weighted complex networks
@ARTICLE{10.4108/eetinis.v11i4.4059, author={Gilberto Sinuhe Torres-Cockrell and Roman Anselmo Mora-Guti\^{e}rrez and Eric Alfredo Rinc\^{o}n-Garc\^{\i}a and Edwin Montes-Orozco and Sergio Gerardo de-los-Cobos-Silva and Pedro Lara-Velazquez and Miguel \^{A}ngel Guti\^{e}rrez-Andrade}, title={Bi-objective model for community detection in weighted complex networks}, journal={EAI Endorsed Transactions on Industrial Networks and Intelligent Systems}, volume={11}, number={4}, publisher={EAI}, journal_a={INIS}, year={2024}, month={12}, keywords={complex networks, k_core, multi-objective, NSGAII, MOSA}, doi={10.4108/eetinis.v11i4.4059} }
- Gilberto Sinuhe Torres-Cockrell
Roman Anselmo Mora-Gutiérrez
Eric Alfredo Rincón-García
Edwin Montes-Orozco
Sergio Gerardo de-los-Cobos-Silva
Pedro Lara-Velazquez
Miguel Ángel Gutiérrez-Andrade
Year: 2024
Bi-objective model for community detection in weighted complex networks
INIS
EAI
DOI: 10.4108/eetinis.v11i4.4059
Abstract
In this study, we introduce an innovative approach that utilizes complex networks and the kcore method to address community detection in weighted networks. Our proposed bi-objective model aims to simultaneously discover non-overlapping communities while ensuring that the degree of similarity remains below a critical threshold to prevent network degradation. We leverage the kcore structure to detect tightly interconnected node groups, a concept particularly valuable in edge-weighted networks where different edge weights indicate the strength or importance of node relationships. Beyond maximizing the count of kcore communities, our model seeks a homogeneous weight distribution across edges within these communities, promoting stronger cohesion. To tackle this challenge, we implement two multi-target algorithms: Non-dominated Sorting Genetic Algorithm II (NSGAII) and a Multi-Objective Simulated Annealing (MOSA) algorithm. Both algorithms efficiently identify non-overlapping communities with a specified degree 'k'. The results of our experiments reveal a trade-off between maximizing the number of kcore communities and enhancing the homogeneity of these communities in terms of their minimum weighted interconnections. Notably, the MOSA algorithm outperforms NSGAII in both small and large instances, demonstrating its effectiveness in achieving this balance. This approach sheds light on effective strategies for resolving conflicting goals in community detection within weighted networks.
Copyright © 2024 Sinuhe et al., licensed to EAI. This is an open access article distributed under the terms of the CC BY-NCSA 4.0, which permits copying, redistributing, remixing, transformation, and building upon the material in any medium so long as the original work is properly cited.